AB and Dr. Mehdi Ravanbakhsh – an expert in the field of geospatial AI (GeoAI) – discuss a wide range of topics related to Mehdi’s extensive experience and contributions in the geospatial industry, including photogrammetry, remote sensing, and AI applications in various domains such as agriculture, forestry, fisheries, and insurance.
Mehdi shares his background, starting with his PhD research in Germany on road crossing detection from high-resolution aerial imagery, which combined photogrammetry and computer vision techniques. The conversation delves into the advancements in photogrammetry, from manual tie-point identification and feature extraction to the current state of automation using AI and machine learning. Mehdi highlights the challenges faced in the past, such as the time-consuming nature of manual processes and the limitations in creating large-scale mapping due to resource constraints.
The discussion also covers the applications of GeoAI in various industries, including agriculture, where Mehdi’s company, Mapizy, has developed solutions for pest control, crop monitoring, and farm insurance. In the fisheries domain, Mehdi shares his experience with a project funded by the Australian Marine Research Institute (AIMS), which involved automating the process of fish counting, measurement, and species identification from underwater videos.
Furthermore, Mehdi discusses his recent visits to countries like Vietnam and Indonesia, where he explored opportunities for national mapping organizations to benefit from Mapizy’s technology. He highlights the challenges faced by these organizations in creating foundational databases, such as national orthophotos and elevation data, and how Mapizy’s solutions can help reduce the requirement for ground control points and improve data accuracy.
The conversation also touches on the future of the geospatial industry, including the increasing availability of high-resolution satellite imagery, the integration of radar and LiDAR data, and the potential of low-earth orbit satellites for data acquisition. Mehdi can’t contain his excitement about the bright future of the geospatial industry and the continuous advancements in sensor technology and data processing capabilities.
Dr Medhi on LinkedIn: https://www.linkedin.com/in/mehdi-ravanbakhsh-phd-94674869
Watch this Episode on YouTube
We’re also publishing this episode on YouTube, if you’d like to watch along in full living colour: https://youtu.be/yjSRSUDOmpY
Chapters
00:02:31 Photogrammetry and Automation
The discussion delves into the advancements in photogrammetry, from manual tie-point identification and feature extraction to the current state of automation using AI and machine learning. Mehdi highlights the challenges faced in the past, such as the time-consuming nature of manual processes and the limitations in creating large-scale mapping due to resource constraints. He also discusses his work at the CRC for Geospatial Information in Australia, collaborating with renowned experts like Professor Clive Fraser and Professor Christian Heipke, who played a significant role in automating the photogrammetry process.
00:09:16 Applications of GeoAI
The conversation covers the applications of GeoAI in various industries, including agriculture, forestry, fisheries, and insurance. Mehdi shares his company Mapizy’s solutions for pest control, crop monitoring, and farm insurance in the agriculture sector. He also discusses a project funded by the Australian Marine Research Institute (AIMS), which involved automating the process of fish counting, measurement, and species identification from underwater videos.
00:54:35 National Mapping Organizations and Foundational Data
Mehdi discusses his recent visits to countries like Vietnam and Indonesia, where he explored opportunities for national mapping organizations to benefit from Mapizy’s technology. He highlights the challenges faced by these organizations in creating foundational databases, such as national orthophotos and elevation data, and how Mapizy’s solutions can help reduce the requirement for ground control points and improve data accuracy.
01:10:09 Future of the Geospatial Industry
The conversation touches on the future of the geospatial industry, including the increasing availability of high-resolution satellite imagery, the integration of radar and LiDAR data, and the potential of low-earth orbit satellites for data acquisition. Mehdi expresses his excitement about the bright future of the geospatial industry and the continuous advancements in sensor technology and data processing capabilities.
Transcript and Links
AB
Well, g’day and welcome to SPAITIAL. This is Episode 22. I have another super special guest with me. I think it’s fair to say my guest here is ‘the OG’, the old guard, the original, pretty much the master.
Friends, love to introduce you to Dr. Mehdi Ravanbakhsh.
Mehdi
Yes, you got it right, yeah, absolutely.
AB
That was the one thing I was sweating on. Nice one. Excellent. I have already passed the test. I’ve hit my KPI for the entire interview. Mehdi, thank you for joining us. Absolute pleasure to talk to you.
Mehdi
Yeah, my pleasure. Thanks for having me, AB and it’s nice to be in your show and discuss ideas with your audience.
AB
Look, I have a full board of ideas. I have full browser tab of things to talk about today. There is a lot that you have been involved with: touch points, professional institutions, companies, startups, universities – the list is massive.
We will go wide, we will go deep where we can. We’re talking remote sensing, we’re talking GeoAI, which is a phrase we’ve used here on the SPAITIAL podcast before. And it is — to help define it from, I guess, a spatial AI or GeoAI.
I’m kind of talking Spatial AI as both spatial computing and geospatial mixed with AI, but GeoAI by definition is the mixture of geospatial with AI. Can you tell us about, goodness me, your wide and storied past, but how this field is the one that has, you know, your name stamped all over it?
Mehdi
Yeah, just a basic introduction to myself. Actually, I have 20 years of experience in geospatial. So very long time I’ve been in this industry, I did my PhD in Germany. And at that time, photogrammetry just started getting into the digital space.
And my thesis was in the area of people call it photogrammetry computer vision, because computer vision started taking off at that time. So there was a lot of research in this space in Germany for image analysis for, you know, building road detection, this sort of thing.
And photogrammetry was a different discipline. But that was the start of it that using computer vision and see how you can do in the geospatial space. So essentially, my thesis was in road crossing detection from very high resolution aerial imagery.
At that time, still high resolution satellite imagery was not available. And so the aerial imagery was around three centimeter resolution was really big thing at that time.
AB
Would that qualify ias anything we would call high resolution today or is that just latent background?
Mehdi
Aerial imagery is a completely different animal. You can see that still the companies that in this space are active, they provide very high resolution imagery. This is something that matched the quality of the drone imagery, especially in the urban environment.
It has an upper frame. But yeah, that’s actually the start of my journey into GeoAI- using image analysis, computer vision techniques and the range of the geospatial processing to be able to help geospatial communities and businesses.
Then I was offered a post-doc position at the Frontier SI. At that time was CRC for Geospatial Information, working with the great guys in this space like Professor Clive Fraser and Professor Christian Heipke.
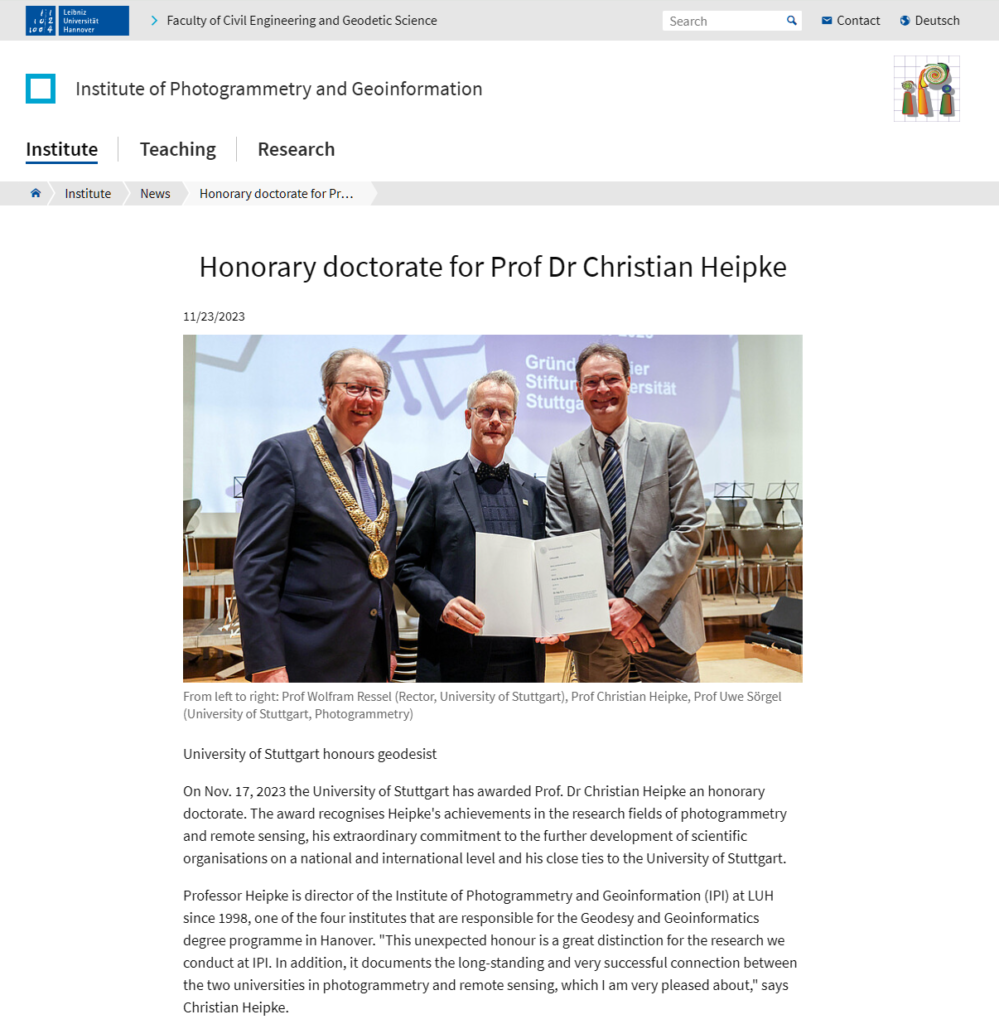
Mehdi
These guys were instrumental in our discipline helping, for example, Christian Heipke helped the digital photogrammetry to the whole process of photogrammetry processing become fully automatic, essentially triangulation, ground control point connection, interior orientations, photogrammetry.
These are a bit technical, but the whole procedure was really long.
AB
Absolutely ground breaking. By all means. Yeah. I mean, the idea of doing that is to actually bake, you know, the number of CPU cycles is huge. We’re talking 20 years ago, 15 years ago, were you working on small patches in high detail or very wide swathes of land in moderate detail?
What was the sort of the limitations and the wild dreams versus what the reality was in the early days of large scale photogrammetry?
Mehdi 04:34
Absolutely. I think the limitation of the photogrammetry in the old days was because that was a manual process. So even finding the tie point, finding ground control point on the image, that was a manual process and triangulation, particularly that triangulation is the most important part, especially making sure that the final output you get from images and reflect the reality, or we can call it positional accuracy of the output.
So to ensure that this positional accuracy is at the best quality and accuracy, you need ground control point and toy point. Toy point essentially sticks images together and ground control point sticks the whole model to the ground.
So that’s the whole, I think one of the time-consuming part. And another important part in the photogrammetry procedure after making sure that the position of accuracy and orbit observation are correct is manually capturing topographic object or man -made or natural object.
And this is a very time -consuming process, especially just an operator sitting in front of the screen, digitizing building, roads, trees, anything on the planet. So that was the main challenge for many organizations that the most time-consuming part.
So as a result, most of national mapping organizations, they were struggling to create, let’s say, larger scale mapping for the entire country. AI actually changed this. Still, there is a bit of human in the loop, but this process has been automated to a large extent.
AB
It’s such a joy to be able to take lots of photos – almost cheating now if you take high res video – but to walk around with a phone just around you. I was in a museum (different part of the world) grabbed the phone out, there were some lovely sculptures. I just did a LiDAR on the fly scan of them and now I’ve got a lovely 3D mesh of them.
Mehdi
Yeah, it’s fully automated. It’s fantastic. I know.
AB
How easy is that now compared to, look, I’m still reeling at the fact you said manual tie points. That would have limited probably your scope, but also meant that you were the authority on probably strong coffee clear the whole day and just sit there and just match up images so that that rock is the same rock as I’m seeing in photo A to photo B.
Mehdi
Oh, I think it’s a significant difference. It’s now super easy. For the grammetry, let’s say after automation of this photogrammetry procedure, as I said in 1992, that was started. In 2008 and 2010, that was almost fully automated.
They are not feature extraction. Essentially, feature extraction means collecting topographic or other features like road and buildings. Not this one, but this triangulation and making sure that the final output is right for the purpose.
This part has been fully automated in 2010. People said that this is the end of the grammetry. If you have photogrammetry, you need to update your resume and then look for jobs somewhere else.
AB
I was about to say that it is NOT the death of photogrammetry. It is simply a democratizing, kill me for saying the word, but more people can now do it rather than the hands of specialists. There’s always a role for that.
But the scale, I’m gonna point out there’s a company based here in Australia, Aerometrex. They have just done most of the Great Ocean Road coastline, which – my wife’s a geologist, she was going, we were gonna ride our motorbikes down there for three months straight, drone batteries, a lot of coffee and coffee stops to recharge batteries.
And in the two or three years, when we were deciding what kind of batteries, what kind of motorbikes we would buy, there are firms now doing that on the largest of statewide, not thousands of kilometers, but hundreds of kilometers, digital twins.
Is that the natural pinnacle? What can you see as the next step from digital twin to GeoAI?
Mehdi 09:00
Yes, actually, I was mentioning that in 2010, then their own K, and suddenly photogrammetry has become a very sexy word. So, everyone’s talking about photogrammetry, drones, and the automation of the procedure.
So, again, even for example, I’m an adjunct professor at UW School of Computer Science. Even people from the field of computer, they say that this technology is amazing. They call this photogrammetry.
Even in the computer science department, they were talking about photogrammetry, how amazing this technology is. And that was the start of drone imagery, fully automated procedure. It’s super easy for everyone to capture imagery.
At that time, you see that a large number of drone companies came to the market and offering mapping and different types of applications, which was great, I think, for the whole photogrammetry community and mapping community.
Because even in some, I guess, respect, it replaced some ground surveying as well, which was a bit risky, time consuming, and costly. That was great. Now, you mentioned that there are a number of companies active in this space, mapping coral reefs, digital towing.
Yes, this is a new trend, especially in combination of photogrammetry, laser scanning. This is ranging technology that can be combined with photogrammetry. But the type of technology that develops over time, particularly in technology, I think one of the fundamental techniques is image matching.
This image matching ensures that you see the details in the digital towing. And there have been some great development and breakthrough in this technology, because previously, it was not possible to create that level of detail.
AB 10:55
I know! My credit card got hit hard when I bought my drone, but it’s still valid. We were able to get down to one centimetre resolution – granted my wife and I were playing with rocks.
Rocks have a great habit of staying still and being high contrast, so you can find them again. So it’s perfect fodder for photogrammetry of cliffs. But now we’re talking sub centimetre and we’re talking not only the quality imagery, but the precision and the ability to determine when it’s a natural object.
And perhaps with digital twins, the main peril was when you had something that was humans would know is flat, would always be wobbly wobbly and it would be hard to correlate. It would make everything into a mesh that was organic, as opposed to the grounds organic.
That building though, I want crisp sides. Is that part of that classification? And if this, then, you know, if it is something that I think is previously labelled, then I shall change my settings. Is that another meta automation tool on top of the photogrammetry landscape?
Mehdi 12:04
Yes, actually, I think this has been a challenge for photogrammetry for a long time when there is no feature like in the coastline and on the rock or on just the wall. For example, for indoor positioning, that always has been a challenge.
There have been a number of solutions in this space. Still, in such scenarios, people recommend to use photogrammetry in combination with ranging technologies. That gives some insight. But compared to the past, these days, technology is now doing much better.
So you have less challenging time working in the area with less features or less texture. So this is, I think, still, there is a challenge because we know that when you use imagery, you need texture.
This is something that AI algorithm are hungry for. They are looking for texture and pattern. So they don’t know which area to match to which area. So this is a bit of challenge. I think for such a project, I guess one of the recommendations is using ranging technologies or combining this with photogrammetry because you get the elevation data or depth map from ranging technologies.
You can combine with intensity from this.
AB
I’m about to say that the drone we bought hit the credit card medium was only sized, but 10, 12 years ago, an infrared camera would have required a second mortgage! I’m grateful to see that infrared spectrum along with RGB visible light spectrum is coming down into, I still think that one still hits the credit card a little bit, but it’s coming into prosumer into potential range to actually give us those other spectrums.
I’m going to jump forward to when you were involved with and use are still deeply involved with farming agriculture, smart farming. Have you seen that the potential for infrared with visible light allows for that full spectrum, more of that image analysis to happen beyond the human perception?
Mehdi 14:26
Yes, absolutely. I guess in agriculture, we know that this is really important to be able to collect it near and far for the health of the, for example, crops or trees or any vegetation on the ground in agriculture.
And I think the challenge in agriculture is especially last week I was in Vietnam and in Indonesia, we had a lot of discussion about challenges in this space. And previously, I had some projects in New South Wales in Goodowindi, essentially, for creating an accurate map of wheat, pest or disease.
I think agriculture is drawn out fantastic, but the challenge is the connectivity in remote areas. And we always, I guess, we recommend that there should be a solution around this. One of the projects we previously was involved was pest control through AI, essentially, rather than using a drone imagery, which requires lots of logistics, and you need to capture the data at – let’s say, a sub-centimeter / five millimeters – and you need to operate it in the cloud. A computer vision company needs to access this. And the whole process takes a couple of days or a week. And by the time that this map is ready, the map has already changed on the ground.
AB
Gotcha. Yeah
Mehdi
So one of the solutions that we recommend, especially in this space, rather than capturing the imagery of the entire farm, which is time consuming, you can just use everyday smartphone from farmers, put it on the wheel of the tractors, capturing every 10 seconds, single image.
And then that gives a hit map of the, for example, wheat or pest or any issues on the farm. Of course, that’s not highly accurate, but that’s, I guess, feasible solution for the farmers. That does not cause any data capture, because we know that data capture and logistic is an issue.
AB
…but to have 4K video processed every day requires hard drives, internet, battery density to recharge versus petrol, internal combustion engine, tractor, phone or GoPro strapped to it last years or a secondhand phone like that and taking a static picture, 10, 12 megapixels every X meters, you know, you could email those around much, much easier.
So yeah, the scale of well, data, IOT, photos, but then videos 4K, that makes perfect sense. Absolutely. Yeah. Not quite low fidelity, but right fidelity for the, for the problem at hand. Yes. All right.
Mehdi 17:20
And it’s quite almost online because you can collect the data, you can process in a couple of hours because the images are not too big, and you see that because the resolution is so high, it’s so close to the object, you see the detail.
You can process it, you can create the event, you cannot cover the entire farm, but from what you can extract, it’s really accurate. So you can extrapolate to the entire farm and you can put it in the tractors and you’re ready to go.
I think this is one of the great solutions, especially in Australia, we’ve got connectivity issues and I noticed the same issues in Vietnam. One of the things that the company came up with an idea that using a terrestrial station and using edge computing, essentially estimating a number of parameters on the farm like moisture and this sort of thing, but pest detection and insect detection was one of the solutions without uploading imagery,
they do it actually through edge computing, which is fantastic because although that’s not about the precision agriculture or smart farming, because for this one, you need to capture the entire farm and find the exact location of issues.
But still, I think for farmers to have some idea of what’s happening on the farm, this is great at significantly lower cost.
AB
No matter where you travel, even if it’s only on, you know, going to the top paddock, the side paddock, you’re right, you don’t get the entire acreage, but you’re getting data points that you wouldn’t get unless you, yeah, did it, you know, for 10 -fold, 100 -fold more robust way.
Any data is better than no data, nothing worse than bad data! but to get samples that give you that confidence in ads to story, you’re almost doing Google Street View for a farmer’s own premises.
Mehdi 19:25
Google should be also good. I guess any existing data sources that you can get access to, I think that’s great. That certainly add values to what you develop. And this is, I think, has been used in not only in agriculture, we also used it for the insurance as well.
So getting some estimation of the situation of the building. So for example, finding the number of floors or the situation in around properties, this is also very useful. So this is a great resource.
The only challenge with Google Studio is that it’s a bit outdated. But as you mentioned, when you’ve got some data, you can use it and you can enrich the dataset.
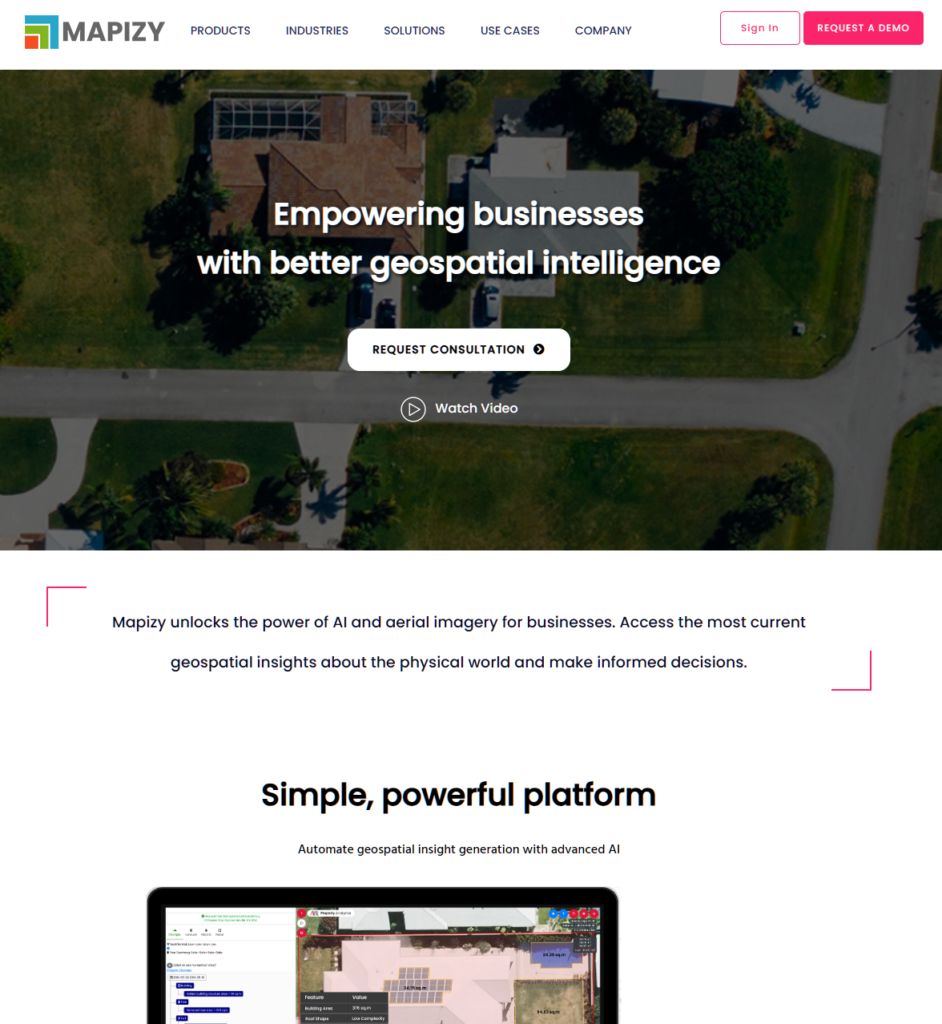
AB
Now, can I ask a loaded question as CEO and founder of Mapizy? Is this the kind of tool and techniques that you’re turning into products? out another way: what’s the focus of Mapizy?
Mehdi
Our mission is to make the mapping an easy task, because mapping is still a complex task. The mapping organization, they have a large department and group just in charge of making sure that the remote sensing observation correlates to the ground data, and the quality check, and all this sort of thing.
We know that it is still a challenge, but things have been a lot easier these days. Essentially, we in Mapizy, we started with forestry, counting trees through collaboration with a local drone company, and then we gradually explored agriculture and fisheries and other areas.
But after a couple of pivots, we found a bigger opportunity in insurance. There was a hackathon organized by Deloitte a couple of years ago, and Mapizy was one of the participants. One of the challenges in that hackathon was how remote sensing data can be used to assess properties for insurance industries through IAG and RAA.
These are two major companies. RAA is based outside of Australia, and IAG is a global insurance company. Mapizy was awarded by both companies to solve two challenges at the same time. We found opportunities.
We had a conversation with these companies. We said that if we develop such things, would you buy from us? They said, of course, this solution is not available if you develop something like this. Of course, a lot of things happened.
They didn’t buy exactly from us, but we had collaboration with them, and they helped us to develop this. Essentially, to be able to use various data sources, that’s Google Studio or remote sensing from satellite radar and aerial imagery.
Using this data and providing insurance companies with accurate condition of buildings, the risks from the surrounding environment, and the way that this has been designed is suitable for insurance because it’s a fully automated process.
Mehdi 22:45
Let’s say if you’ve got millions of property addresses in Excel file, you can upload into our platform. Automatically, this will be processed. Excel file will be populated with a large number of attributes, at least 30 plus attribute changes to buildings and properties.
Then they will be notified when this data is ready. But in the traditional sense, normally, some big insurance companies, like the GIS department and large team, they are in charge of doing this manually, which is a time -consuming process.
We did this in a way that is fully automatic. It uses kinds of machine learning on -demand processing. If you’ve got clients, we process it so the clients actually pay for the processing time. If not, everything actually will be in the cloud with no cost for us.
It’s a kind of sustainable business in this space because, as you know, GIS special space is one of those spaces that the GIS special data means different things to different businesses. Making a product that everyone uses GIS special data is really hard.
AB
It is, isn’t it?!
Mehdi 24:01
So, you have to be very specific and in this case, we just targeted the insurance market because although the agriculture is big, the forestry is big, the fish is still, I guess, compared to other people performing.
AB
High priority, yes, no, it makes perfect sense.
So what were the sort of things that you had? What were the sort of things you could add to that data set per property address? What were some of the low, mid and high level sort of inferred attributes you could add?
Mehdi 24:39
Actually, the information that we can extract from existing data in different categories. For example, the building itself. For example, what’s the quality of the rooftop? We can estimate the quality of rooftop at the tile level.
If there is a broken tile, you can’t find that. So that’s what the shape of the roof and the shape of the roof is really important when there is, for example, a strong wing or a tornado or things like this, the shapes matter.
And that’s one thing. And also the information about the land parcel, whether that’s, for example, a swimming pool or, for example, information about shoulder panel, whether there is a tree, a risk of tree to the building, we call it tree overhang, whether there is a shed in there.
Mehdi 25:36
So any types of things that could be liability and can create issues for the building and risk, we identify them, we quantify them. So that’s inside the land parcel. Should be started from building land parcel and out of land parcel is this size to water bodies distance to the bushland.
And the size of the bushland based on insurance definition should be over one hectare. And these are a bit of detail, but for all of this, we develop an algorithm that, for example, if there is a bushland, we identify the soil should be over than this.
We estimate the size of the building to all these objects that pose a risk to properties. And so this information, just one step. Another step is change detection of these attributes over time.
AB
I was about to ask, doing it once is spectacular, but it’s becoming back in a regular cadence and far more regularly, there you go, people wouldn’t be driving past or doing a light plane overhead inspection, so having it in the system and just go back to what asked the same question over time and if the value changes, red flag or start to warn, gotcha.
And then what sort of time series data can that give you per property? And also does that upscale to trends per suburb per region?
Mehdi 27:03
Actually, this is a great question because we know that in change detection, this frequency matters. For different applications, especially in the case of natural disaster or flooding, the frequency should be on a day or week basis because we see a lot of changes.
Insurance companies need to be able to very rapidly assess the damage and making sure that they’ve got enough funding in the bank to assess claims. This is critical in this difficult time. Having said that, when the business is normal, as usual, so there is no natural disaster, normally, this yearly update is necessary.
Every year, they update the policy. This is really critical for user experience because still there is lack of confidence and trust in insurance industries, not in Australia, in some other countries.
Mehdi 28:00
People, they say that the insurance companies, they update the policy, increase the fee, without no apparent reason. If you use this change detection and change analytics and say that, look, for this reason, for example, you added a shed or pole, or for example, you add a solar panel, you don’t have protection, you need to update your policy.
I think this is a great user experience that overall good for the industry. It’s essentially a data -driven decision and the update of policies based on the data. That’s change detection. Also, for claim assessment, this is also another important aspect that during natural disaster or for whatever reason, people lost claim.
But you know that although most of the people provide accurate information for insurance industry to be able to validate claims, they need the independent data set. We can provide them the current condition based on accessing the latest remote testing data.
You mentioned, for example, for regional Australia. Regional Australia, of course, is the challenge. I guess remote testing these days, very high resolution satellite imagery at around, I guess, better than 50 centimeter, these days are available.
You can get access to it a bit pricey because normally for regional, you have to order the satellite and pay a bit more. But the good news is that it’s available. If there is an emergency situation, you can purchase imagery, you can order in, let’s say, one or two days, you can get your imagery.
For major metropolitan areas and densely populated areas, we have no shortage of data. There are lots of data. Now, as you mentioned, there are some leading companies like Aerometrex, Nearmap, they are quite well known.
They capture data regularly. And so, this data can be used. Satellite companies regularly capture large cities all around the world, at least once per week. In the urban areas, always there is, I guess, abundance of data set.
AB 30:19
If we look on Google Maps, probably our houses haven’t … I mean, I still can look at the Google Map of my house here, and there’s a car in our driveway that we haven’t owned for seven, eight, 10 years.
You can sort of date … I think that was if there was “the black car”, then it was 2000 and large swathes of Google Maps, which is one of the predominant daily tools for us. Again, Ask Me Immortals, they don’t change too often.
It’s really heartening to know that there are companies out there that, if you do hear a light plane flying overhead, chances are it’s either, in my part of the world, down the surf coast, either a shark spotting, fire spotting, or it’s one of the commercial light planes doing those oblique, downward facing intermediate altitude, but high resolution, high frequency updates.
It really is mind boggling to know that there’s that much starter floating around, if only someone had a big credit card and knew how to get it, but that is phenomenal that there are companies who just specialize in doing, say, low altitude, high definition imagery at a high frequency.
AB 31:26
Have you also had, or how do you see the move to low-earth orbit satellites? Starlink, Mr. Musk, of course, doesn’t generally have cameras. They are internet comms, but planet.com is another constellation of low-earth orbit meant to be freely available, insert credit card here, satellite imagery, and visible bands.
Is that a new technique that’s coming through, a new data set that’s possible, or what are the advantages or disadvantages of low -earth orbit versus traditional plane -based imagery?
Mehdi 32:04
I guess these days there is a good source of high definition, high resolution satellite imagery. I say very, very high resolution because there is two categories, high resolution or very high resolution.
So, high resolution normally… I feel like those labels might have to move in.
AB
10, 20 years time to ultra, ultra high and no, no, no, really ultra high.
Mehdi
it’s yeah
AB
Absolutely, but sadly correct for today. Gotcha. What are those definitions? What do they have?
Mehdi
Yeah, high resolution from 1 to 5m, but anything below 1 meter was very high resolution and it started in 2010. In 2010, GEO, AI, IKONOS, QuickBear, these are satellite imagery that launched mainly from the digital globe.
Now they changed the name to Maxar and I was lucky that I was involved in calibration of some of these sensors with Professor Clark Fraser at CRC for geospatial information. And then Airbus launched playouts and other companies planted then came to existence and they have their own constellation.
Now we see that recently there is a good source of radar imagery, especially for flooding mapping for insurance, that’s very important. And especially for different types of, I say, complex applications that normally aerial imagery is a bit of a struggle to identify some of things.
You can use a combination with radar, especially over, for example, the marine environment and this application is also very good things. This is a new trend that you can see high quality radar imagery in combination with optical and so now, for example, at the current state, there are companies that they convert very high resolution satellite imagery.
They add more detail through AI. They add more better geometric definition to the detail, which we in my PC, we already had this technology. We were using this for a long time. These are new developments in this space that you can see that because, as you know, US companies, they are not allowed to go above certain threshold for security reasons. So they can’t really produce raw imagery at, let’s say, 20 centimeter resolution. They are not allowed. Although maybe in military applications, they use it, but for civilian applications, for commercial use, they are not allowed.
Mehdi 34:43
Having said that, I think this is still fantastic for many applications. For example, for insurance, we noticed that the same level of detail you can get from aerial imagery. You may not be able to get from satellite imagery, but still, the main features are there.
For example, trees, buildings, if there is a pool in there, you can see it. For solar panels, I think it’s still, I guess, algorithms are struggling to find solar panels on the rooftop.
AB 35:14
You’re looking for edges that may not be there very much. Yeah, absolutely. Can we talk about, we did before we pressed the record button here, we talked about super resolution. And I guess this is one of the, sorry to put a pun in or a dad joke, but this is one of the superpowers of remote sensing or GeoAI that are probably most categorised by me eye rolling whenever watch movies for the last 20, 30 years of, you know, ‘enhance, enhance’ and magically mission impossible ‘insert spy movie, sci-fi movie here’.
You know, you can’t read someone’s newspaper from over their shoulder from a satellite. I don’t think so anyway, but tricks of Hollywood notwithstanding, I’ve been flawed in recent months and in the last year of the advances in super resolution, things that you would not have thought possible.
It’s talk about a dark art. It’s also on the cusp of, I’ll say it carefully, keep it family friendly, making stuff up. There are techniques using AI to (I’ll ask the question in a second, apologies!) but to look at obviously with high resolution source, if you had it first, you could down-res it and then ask AI how you get from one to the other. So how you would up-res it in the future, but it still feels like it’s not real. It still feels like we’re seeing images that are, have a resolution that is, yeah, tree level, can kind of figure out the ages of buildings vaguely if you squint.
But then these techniques are looking at edge detection, minute subtle changes in well -calibrated digital imagery and back inferring or down inferring, shadows, doors, things on roofs, things that if you really squint it, a human might be able to say, oh yeah, there could be a car parked behind that house, but I wouldn’t want to bet my life on it.
And these tools can, to different levels of competence, detect another doubling, another four times, another zoom level in that is just unbelievable.
Can you tell us about, am I joking? Is super resolution a thing and how much have you been able to embrace that kind of technology?
Mehdi 37:25
Yes, I think that’s that’s real. And one of the I guess the development behind this is very accurate and recent image matching techniques that and technology is you know, to create, to capture a lot of imagery through a combination of lenses through camera system that just with the one single shot, you can create a depth model, right depth model.
And so essentially, the geometry because traditional photogrammetry is overlapping imagery. When you use drone, you capture imagery in a way that should be 60% overlap. And this one is completely different.
So essentially, the way that lens has been organized within the, the camera, you can capture a lot of images in different lens distances. And then through this, you can create a depth map, a single shot.
Mehdi
So perhaps from that…
AB
I see – so from the one viewpoint, but different zoom levels, that gives you enough of the perspective shift to reconcile what it was in 3D?
Mehdi 38:33
So that’s a new technology that created a lot of opportunities in various applications, not only mapping, but in the, for example, in food processing and monitoring. But having said that this technology by Tesla has been used, just single camera has been used to create a depth map.
We use the same technology in another project for fish measurement and the quantification of fish population, especially in the, this area is called closed range photogrammetry because you are close to the options.
You are not that far. And in the underwater environment, we use normally stereo cameras. We use just a single cameras. And when we capture the picture of fish, we use an AI techniques to create the depth map.
And we use that through, of course, it requires a lot of training data. We create training data through photogrammetry, through traditional photogrammetry, but train the AI models. This is exactly the way that Tesla is doing.
So the way that Tesla creating 3D model online is through the same technique. They use it to serial laser scanning data with streets. And then they use a single image to train the model to understand the depth.
AB 39:53
That’s phenomenal. I must say I was in a separate field two years ago with robotics and the trend was happening there too of say tractor wheeled vehicles. And at the start of that journey, those robotic platforms had multiple cameras.
And yes, they could do depth perception on the fly if needed. But the computational power to do that on the fly was pretty harsh. And if they lost one, ironically, having lots of eyes and lose one, that really hurts.
Versus if you have one eye or probably two, but both of them doing the same task in a monocular single fashion, then using AI to help you with that algorithm means that you’ve got redundancy still, but any one camera can just figure things out mostly by itself.
So that reduces the risk of overthinking and having spurious data throw your model into a spin. Less is more. And to quote Mr Musk calling the Tesla out, he famously in one of the interviews regards SpaceX said “the best part is no part”. And to have AI let us do that only two years later is just phenomenal. The depth perception, grayscale mapping from single images, even on dodgy cameras.
Now, it is just unreal to be able to see it’s been fantastic. So you have been involved in the calibration of underwater versions of that. How did you get involved with
Mehdi 41:28
Yeah, because as I said, I was lucky to work with another world leader in photogrammetry at RMIT University, Professor Mark Shortis. And he’s one of the leaders. And actually, we work three years on the research project for fish measurement calibration.
We use pool environment for calibration of the camera. So that’s, I think, one of the important steps in any remote sensing photogrammetry, you need to have a test fish. Of course. But then that’s great, quadrupole and pool environment.
So you need to calibrate things to ensure that the measurement you get is actually correct. Well, if I…
AB
If I can ask about the fish measurement, if you’re looking from a satellite down, you probably have things like a house or a car or a road. There’s probably times when you can grab out the virtual ruler and sort of maybe self calibrate or at least bring us off back.
But surely with fish, murky water, how do you know whether it’s a, was it a little fish close to the camera or a big fish far away? How do you get any sense of scale if there is not a lot else apart from murky, muddy water?
Mehdi
Yeah, I think the thing is that if you believe in the physics, I think that’s the physics principle. I believe in the physics level. Because we use underwater, we use two cameras, and it’s like the principle we use in photogrammetry.
So you see the same object from two different perspectives. Yep. So therefore it must be a depth x. Yes. And you can estimate the distance, so that’s one thing. But the most important in all the distance, we need the distance just to get fish length.
Gotcha. So essentially, length measurement is the most important thing. So if you know the depth, this depth will be used for measurement of distances.
You know, in reality we normally use DTM or depth to rectify the, I guess, positional discrepancy through caused by depth or height. So this is for creating, let’s say, orthophoto, you need to create this data first.
And for fish measurement, of course, we don’t have topography because this is underwater. But yeah, and the view is like an oblique view or a vertical view. Sorry, horizontal view rather than vertical.
But you need to be able to create some sort of depth map that gives you some scale for measurement. Normally, for the grammetry, I think this is one of the fidelity of the scale. You need to solve the scale issue.
So the scale issue is solved by the depth map.
AB
Gotcha, can I ask what were the applications of FishCam? What was the name of the project and what were the uses for it? Fish ladders or fish farms? What was the?
Mehdi
Yes, the application actually was supported by Australian Marine Research Institute, AIMS, and the grant was supported by the Australian government, 250 applications received, and four has been funded.
Mapizy was one of them, because we had track record and fish measurement accounting. And then the purpose of this was that AIMS, Australian Marine Institute, they collected videos all over Australian coastline.
They captured, let’s say, one hour videos in 100 -meter depth, close to the shore. And then the challenge they had, they didn’t have enough resources to manually go through the videos, count, and measure fish and identify species.
AB
This is a really time consuming process.
Mehdi 46:03
Yeah, but I’ve been, as you know, this has been a research topic in many universities, but the challenge is that most of this work was not suitable for industry and was not suitable for the Australian environment.
Mehdi 46:17
Because fish species, the quality of the water, and also the hardware, so everything was different. So we used the existing system that Ames was more comfortable with, and they used it in their operation.
We didn’t want to change the existing processing workflow for them, because they already used some of the tools and techniques. We just automate the procedure, especially to be able to make it more accurate.
And hopefully, in the future, we replace humans for this test. And one of the interesting things about the length measurement, which I noticed during this project, was that when fish are moving, there is a rotation in the body of the fish.
And then you need to, with AI, you need to identify whether this fish is vertical to the camera, or there is any movement in the body. When they are in both cameras, when they are exactly straight, then it’s a good time for measurement.
Otherwise, the measurement is not accurate. Now, to be able to find those important moments, you need to try.
AB
Boundaries of fish, yeah, indeed.
Yeah. And tracking for fish is different to cars, you know, because, you know, a large number of them come together. So they, they change direction suddenly.
AB
Such work in self-driving car and car safety technology, which is brilliant. Love it to death. But it is all from the point of view of four or five feet off the ground, forward-facing down a road. Love it, because it means we can try and solve that problem as, you know, to as nine -nines as possible.
But there’s not a lot of other data sets that are cars around. There aren’t a lot of long-running drones. And so to have sterescopic imagery from around the coastline and have it just supplied with, I guess, the metrics known of the camera distance, the focal length, the cameras themselves to get rid of distortion, that’s just brilliant to be able to harvest that.
AB
Can I ask what the accuracy classification was? Was it comparable to humans? Not as good, but more massive, therefore reduced error by volume and by more measurement points? Or was it starting to surpass a human’s capabilities?
Mehdi 48:45
So the accuracy was around 1cm, that was the phase one of the project, we achieved accuracy around 95. But the standard was a bit higher. So we developed a process that tools that for AIMs to be able to feedback the result to the training loops.
For example, if you notice that the fish has been identified wrongly, you can remove it for the entire video because we track every fish for the entire video. So this is the whole procedure because we know that in an AI project, this is really critical to be able to develop a feedback loop and improve the quality of the AI models.
Mehdi 49:35
We developed that for AIMS. And having said that, the most innovative part for us was the measurement. So this measurement was the most time -consuming part because you need to track fish and then you need to measure into different frames and you need to measure all of the fish.
So imagine that for each of the fish, you need to track them for a long time. When you see that it’s a good time, in that perfect frame where they are just silhouetted.
AB
So I can see that the AI is doing multiple tasks at the one time. I’m also, you did probably didn’t notice, but my jaw just about dropped to the floor where you said you are not only tracking fish, I can’t believe we’re having a 10 -minute conversation about fish, but I’m actually literally astounded.
Not only are you tracking a fish, but you just said you’re actually tracking the individual fish. That technology for humans was pretty well impossible science fiction a few years ago to bring it back to the domain of cars.
It was very common for a forward-facing camera on a vehicle to say, that’s a human, no worries. I can identify that, the silhouette’s known, even give it an ID, but then a few frames later if they happen to go out of shot is me and come back.
Before recent times, that would be rocket science, it would just be classified as a new human giving a new ID and would be consistent throughout the frames, but not consistent as I’ve seen that one before.
You’re able to do that in a brand new domain is just shocking technology and phenomenal that you’ve been able to adapt. Getting into the weeds and asking the big, nerdy question now, were you starting from scratch?
AB
Were you able to harness large, open source models that had some of the domain and latent domain knowledge or did you literally start line one, position one with training data and here’s what we need to do?
Mehdi 51:29
Yeah, I think AIMS was great in providing, they actually created a large volume of training data of the fish species, and they made it public for everyone. Gotcha. So if you are a researcher, you can use those open source data sets.
If you are a company, you can use it. And this is great. I’ve never seen such things in other countries. It’s a bit hard. So it requires a really dedicated team to create this, especially fish population.
There are a variety of species, and unless you’re an expert, it’s really hard to recognize them. At least I must have learned a lot. Well, at the end of your project.
AB
Can you now recognize fish at a glance – your superpower?
Mehdi 52:14
Yes, at least in a broad category, I can recognize that it’s still a challenge, not recognizing the different types of things. But yeah, I guess this is a good thing about geospatial industry that although it’s kinds of not these days, merged with computer science, computer vision, machine learning, this sort of thing, there are lots of overlap.
But you learn a lot working with different industries and end users and you learn the various things from agriculture, forestry, fishery, to insurance, oil and gas mining, urban planning, and various different applications that I think the beauty of surveying engineering and geospatial these days is one of these things that you will not find in other disciplines because other disciplines are just focusing on one thing and one thing.
AB
Almost anything, we are looking at the patterns to be able to transfer that from top -down imagery and what can I infer to the worst of murky waters around the coastline of this country is phenomenal, but I can hear that there’s the same patterns, the same challenges thrown at you, not the same tool sets, but the same way of tackling the problem in a logical and masterful way.
Can I draw back through your recent, and I’m going to say very recent LinkedIn history, can I ask what you’re doing in Vietnam and Indonesia in the last couple of weeks? You’re obviously linked to some Australian trade, attache, what’s it say, holidays, mission, thank you kindly, I was about to say holiday, there probably wasn’t a lot of time sipping a pina colada on the beach.
Can you give us a bit of a rundown on what you weren’t doing on the beach, but your kinds of meetings and the kinds of firms and the kind of teams you’re meeting with?
Mehdi 54:08
Actually, I’ve been very active in last year visiting different countries, especially in Asia and the Middle East, exploring opportunities and see how they can benefit from our technology. One particular category of clients are national mapping organizations, and especially they are struggling for creating foundational databases, let’s say, for example, national ortho photo.
And as we know that this data is critical for the economy. Once you’ve got a good quality foundation data set, you can distribute this to businesses and they can benefit from this. For example, orthophoto or photo map is one of them, a grand database of grand control point or secondary grand control point is another one.
Mehdi 54:58
For example, the national elevation data is another one. That was one of the projects that we worked with the national mapping agencies in Thailand, Saudi Arabia, and Indonesia, helped them to reduce the requirement for grand control point.
It’s a bit technical topic, but essentially, in order to be able to reduce the requirement for collecting grand control point by sending people to the field with the GPS, collecting the data, bring it back to the office during the geo-referencing of satellite or aerial imagery, this is a very time -consuming.
We developed a technology that can reduce the requirement of the grand control point, and this work was awarded by the American Society of Geometry and Remote Sensing, a couple of years ago. This technology is very unique, and we know that in the world, a few people can do such things.
We helped this space and mapping organization with this technology. That’s one kind of line of work. Another was in different markets. For example, in Thailand, Indonesia, agriculture is a big thing.
Forestry and crop performance, farm insurance is a big thing. Unlike Australia, which is property insurance is equally important. In Asian countries, we noticed that they are significantly different, and we need to pivot to agriculture.
That’s because Australia properties fall apart from each other a couple of meters, and you can see a nice backyard with a range of things. In Asia, you cannot find this. Often, you can see that you don’t see the border between properties.
It’s really hard to differentiate them unless you use the cadastral boundaries. Again, these cadastral boundaries in Australia, we are lucky because this is open to the public. You can get it, but in other Asian countries, this data is not available, and you need to be able to bear through some connection to get it, or you need to justify it.
AB 57:09
So in both cases, you’re talking of a national foundation database that is open, free data and but that the quality is all for SIGMA, it’s all the nines and it is constantly being tended to, there’s data governance, there’s data conservation and tending to it.
So there’s a common repository that people can trust and expand on versus competing regional and sub-regional and I’ve got a patch of data here that’s kind of okay, but it doesn’t match up with the national set.
Yeah. Can I actually just walk back a couple of terms we just talked about there? If people aren’t exactly au fait with them, I’ll give a lay definition and when I’m wrong, please correct me. Ortho, so ‘orthophoto’, orthographic, essentially means top down what we would normally associate with a paper-based map.
So it’s the, not the flattening, but it’s the view from the satellite view from space, the literal top down, there’s no 3D per se, it is just the, if it was squashed to a 2D, what would it look like?
AB 58:13
Another topic there which I’d love to talk about is a ground control point. Historically, survey points are the ones that we’d use if we’re doing surveying large sways of land and you can still run across them in many countries of the world, concrete blocks, there’s almost one on your local mountaintop that would be used and a brass plaque with a dot in the middle that your surveyors would lay there.
Ooh, got a light, that tool, but now we’re talking digital versions of those, so known nodes, known places, but rather than putting them physically in the land, what you’re saying is you can then find a way where if you find a really solid known point in your imagery, in your data, you can then go out or you can have someone go out with a GPS with multiple satellite signals and get such an accuracy on this one point, you can almost stretch your map to fit where it should be, is that like a retrofitting of the data set? So rather than starting with known data points and working forwards, you can work from a data set and infer where it needs to be to be 100% correct to that land terrain.
AB
What’s the advantage that a nation state would have in maintaining its own geospatial data?
Mehdi 59:57
I think one aspect is currency and the level of detail, because in the elevation data, we know that there are data from NASA that is available worldwide, but at the national scale, to speed up the construction project and different types of projects, you need better quality data.
And that means no more detail and more up -to -date, and then you can provide businesses with this data so they don’t need to create this data for themselves. So that’s one thing. But coming back to your question about Asia, one interesting use case that I noticed this time, especially in Vietnam, was illegal building detection through drone imagery, which because not previously, I thought illegal buildings happened only in Australia.
But in Asian countries, they got buildings and like in Vietnam, especially to be able to use their own imagery and find, especially people, it’s amazing. Sometimes they set up buildings overnight and it’s not a real building, but they know they just set up in this way and they start building.
AB
And then after a year passes, there’s statute of limitations and hey, I know that’s not real, but it’s been there for so long. It is now. So what you’re saying is it’s vital for every nation to own that data because of cultural norms of differences and also the fact that you do need people on the ground to validate that a global data set just doesn’t have the resolution.
The classic elevation data is from NASA from the space shuttle. They basically, as they were doing laps and laps and laps, they would have a single laser rangefinder would give single elevation as it was doing all its hundreds of laps.
That data is, well, 80s and 90s and I don’t think it was ran in the 2000s, but we’ve had lots of inference to upgrade that data. We’ve had a lot of calibration to make that data better.
AB
But that still probably is the baseline for the world’s elevation data. I know I was using it for a certain Australian client who shall remain nameless. That’s OK. And I was being challenged of what’s the accuracy.
And I’ll embarrass the team who I was talking to saying, look, it doesn’t matter where I live. Elevation is 12 metres or 12.0572 metres. And elevation is a guess. If you move two metres left, right, north, south, east or west, it’s going to change.
It’s nice to be close. But being correct to seven decimal places is probably overbating the cake slightly. So but that’s what the satellite was able to give us. It was sorry, the spatial was able to give us the first pass, but it’s an estimate based on thin data points..
Mehdi 01:03:09
Yeah, I think as you mentioned, elevation, I guess one of the biggest challenges in mapping community because still, AI still isn’t able to give us very, I guess, acceptable accuracy elevation data, and still we have to go through the regular processes or use the ranging technology that is a bit expensive.
And we know that if we use the traditional techniques in the mapping community, elevation is something that you get indirectly through a lot of computation. So just getting this data, you have to go through a lot of processing.
And so as a result, it’s a costly data set. Yeah. So it’s a bit of challenge, I guess, still in the mapping community, although they’re picking the laser scanning, ranging technologies like radar, LiDAR, but this technology is still, still expensive and they need to
AB 01:04:07
Can I tell my pub trivia point? I hope you’ve heard it. Probably all our listeners and viewers won’t have, so I’ll go through 10 seconds of it and I’ll see if — please, again, correct me when I’m wrong!
Apparently in the 1920s, maybe 1910s, England sent out a very expensive team of surveyors to get the height of Mount Everest. They knew it was the tallest mountain in the world, and thay they could do early estimates, but this time they said, “right, we’re going to do this properly scientifically”.
They started from one of the Indian coastlines and they worked their way methodically over many, many months to get the angle from their last known good to finally get the angle and they could cite Mount Everest.
I’m going to change tabs here for a second. Yep, we now know that Mount Everest is 29,031 feet plus or minus eight and a half inches — or bit under 9,000 meters, but the feet was the important one. They had error in their data, but they came back.
Well, when they finally took the final measurement of Mount Everest, they calculated it to, drum roll, 29,000 feet, and they went, oh no, we can’t possibly after a many multi-month, multi-million pound endeavor, go back home and say that Mount Everest is 29,000 feet on the dot. It would be as though we just said, “oh yeah, it’s 29 ,000 feet”. In a history of significant digits, they added, I think, six feet to it. They said 29,006 because then it sounded like they knew what they were talking about.
Of course, the number has been updated since then, but that just shows that the importance of elevation and how close do you get depends on the year, depends on the day, depends on everything. The elevation question is still something that, yeah, is a tough call.
Not only does snow and make things rise or fall or water and precipitation makes everything, the valleys get deeper and the hills slide down more, trees and foliage. There is almost no good answer for elevation here into that joke story.
Was I kind of right in that story? Had you heard that story before?
Mehdi 01:06:17
Actually, not at this level of detail, but it was fantastic and fascinating to see that people are interested to get the measurement very accurately. This is so critical to be able to get this. I think this is, of course, to get the attention of people worldwide, but in other applications, elevation is so important.
We noticed that in various applications from now at the age of climate change, estimating the height of the tree. I didn’t know that it’s so important because the height of the tree has something to do with shadow and shadows links to the consumption of water through air cones and this sort of thing.
So for local councils, it’s so important if you just give them the coverage of the tree, that’s not enough. They would like to know the height of the tree as well.
AB
I’ve noticed that there is almost no human superpower ever for estimating heights of things. Even estimating a telephone or a power-pole close to you, and you’ll be off by a massive factor.
There’s been times when I’ve flown the drone, kept the camera level and went excellent, it’s 14 meters high. It’s vital when you’re flying a drone to know that the highest thing in your vicinity is 15 meters high, therefore I’m gonna stay at least, you know, 10 meters above that.
But humans don’t have any multi -thousand -year history of estimating the height of things, it’s just not in our toolkit. So, glad to hear that.
Now, Mehdi, can we turn from what you’re doing last week in the last few weeks, you’re just sitting around the world, can we get you to put forward your vision of the future?
What is, well, first of all, what’s on the dance card for Mapizy, but also what are you most excited about in the next two or three years, even five years? Don’t go so far out that we have to put in, you know, 20 years on flying cars and that kind of thing, but what are the things that are really for you, your things that get you out of bed every morning, the things that you’re looking forward to seeing come to fruition soon?
Mehdi 01:08:25
Yeah, absolutely. I guess this is really important to know about the latest technology. And for us, to be able to help businesses with better quality data, with their new application, new use cases. Recently, we worked with mining companies for carbon measurement through satellite.
And this is something really great for mining companies, because it’s exactly fit their criteria. Because with satellite, we can access remote areas, we can get extra information they need for the carbon measurement.
The same concept for, for example, the farms. It’s really important to be able to have farmers to make sure that they are compliant, they reduce their carbon footprint. So these are the new things for insurance companies to help them get better quality data faster, more detail.
And this is something that we are working on. And there is no limit in this because more sensors and data becoming available cheaper, on a more frequent basis, we would like to be able to access this imagery, process faster, create the insight that industry needs to have.
And working with different industries, not only just insurance. This is actually my passion working from national space and the mapping organization through to local drone companies, companies like Toyota, Airbus, we’ve worked in the past for a time and we’re still having road mapping.
So doing various things, although we have a platform and product, but we are not limited to just one product. We are essentially a product and service companies. And this is actually my passion to learn from other industries doing different things.
And because that’s one of the reasons I quit my job in academia, because I did just building detection for 10 years. And I said, I don’t want to do building detection for the rest of my career. Very cool.
So that’s the great things about us. And you know, the future of geospatial is really bright. It’s one of the interesting science and technologies that is so critical for a nation because every nation has a geospatial agency.
That’s so important to be able to support various other organizations that they rely on this data. And the future is more sensors, better quality sensor. And so that’s a great future, I guess, for geospatial industry.
AB
Absolutely. Like the last few chats I’ve had, I support you a thousand percent. That’s the convergence of these fields that I’m loving to follow. The fact that the geospatial world is finally gone from famine to feast of data and more data is always better.
So this day and age in 2024, data is plentiful and we can store better, we can process it better. I’m looking forward to when that’s even more of a just a hand wave than it is today. It’s the days I think in the 90s when you’re playing with couples of dozens of digital images to try and rectify them for photogrammetry to now I can go into a museum with my phone and just casually scan a picture of a 3D mesh of a sculpture while I’m passing it by.
I’m looking forward to when the grandkids, I don’t know, the hollow lenses on the headsets and I’ll say it when these glasses that we’re wearing now are everything that we need to overlay data with the real world.
That’s the sort of the spatial AI that would be epic in my books, but you’ve actually been on the forefront of this field for such a wonderful period and it’s heartening to better get some of the sense of the data journey you’ve had, the business journey, but also that learning journey.
I’m wrapt to hear that the lessons that you’re playing with right now are transferable to other nations and you’re able to use that wealth of experience to assist other countries to get their own data sets into healthy states and really fast track.
AB 01:12:35
Absolute joy to have this chat with you. We’ll put thousands of links in the show notes. These tabs I’ve got over here which I’ve been casting my eye at the last couple of minutes and goodness me there’s so much I would love to cover with you and dive deeper.
Mehdi, can we get you back in a little while? Can we also anytime you’ve got something that is of great interest to this team, can you give us a call? We’d love to catch up and talk about another one of these topics that we’ve barely scratched the surface on.
Mehdi
It was an absolute pleasure to have a chat with you, discussing the interesting things in our geospatial industry. As you know, we are a world wide, we are a small community, but we have the biggest impact on various industries.
So it would be great to, as you mentioned, to be back in your show in the future and give you an update about the latest development in this space.
AB
Oh, that’d be lovely. And I, and we shall all live vicariously through your worldwide travels. I dare say your passport is well stamped. So my congratulations to you. Again, thank you for your time. Absolute pleasure.
Thank you. Goodness me. We’ve, we’ve had a long interview. We shall leave it here, but from everyone at Spatial, we’ll catch you on the next episode from us. Farewell.
HOSTS
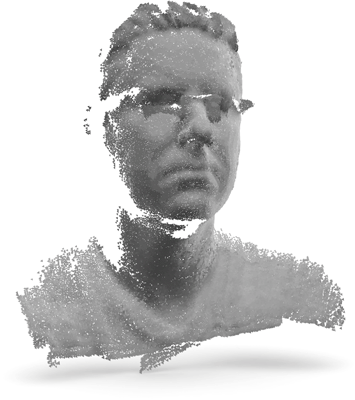
AB – Andrew Ballard
Spatial AI Specialist at Leidos.
Robotics & AI defence research.
Creator of SPAITIAL
To absent friends.