HOSTS
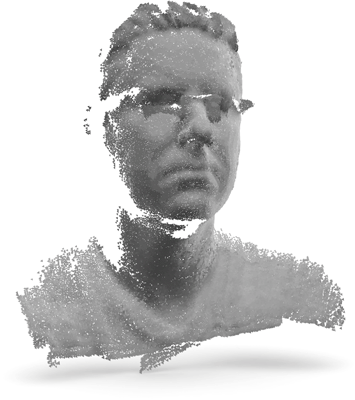
AB – Andrew Ballard
Spatial AI Specialist at Leidos.
Robotics & AI defence research.
Creator of SPAITIAL
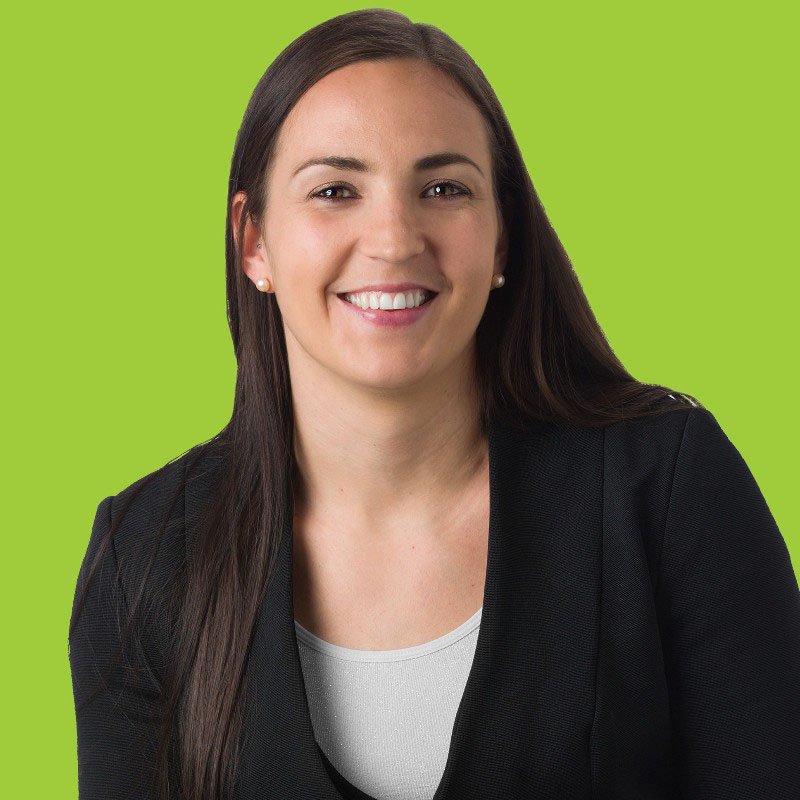
Helena Merschdorf
Geospatial Marketing/Branding at Tales Consulting.
Undertaking her PhD in Geoinformatics & GIScience.
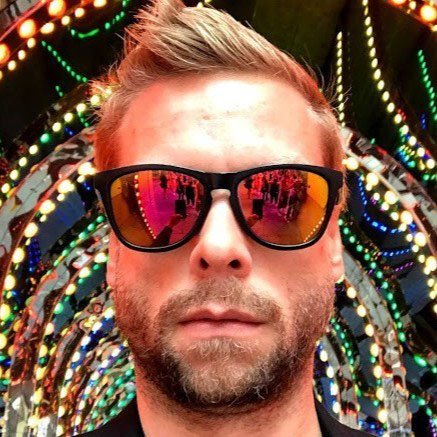
Mirek Burkon
CEO at Phantom Cybernetics.
Creator of Augmented Robotality AR-OS.
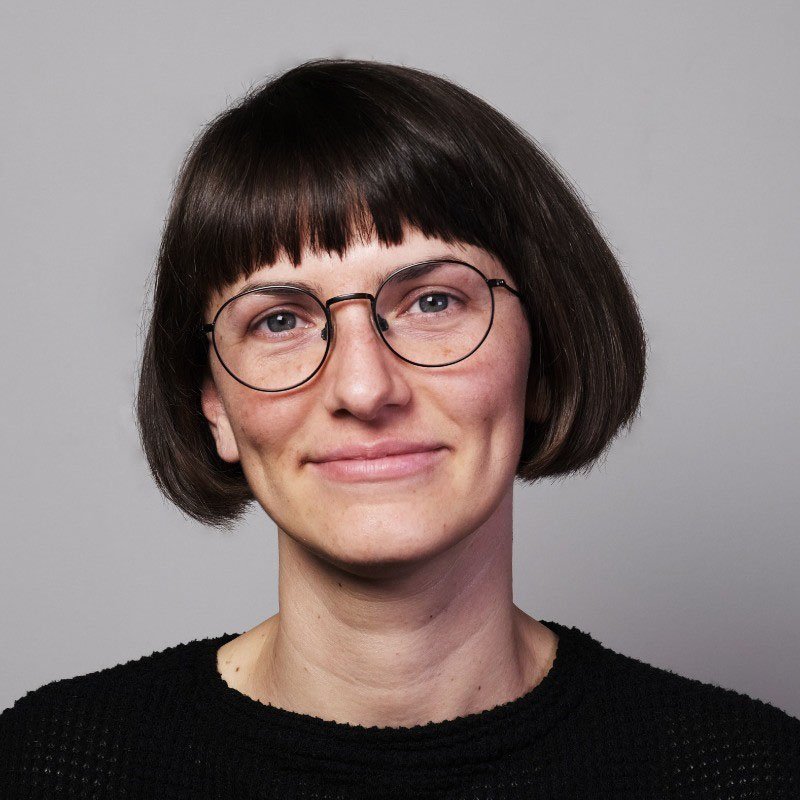
Violet Whitney
Adj. Prof. at U.Mich
Spatial AI insights on Medium.
Co-founder of Spatial Pixel.
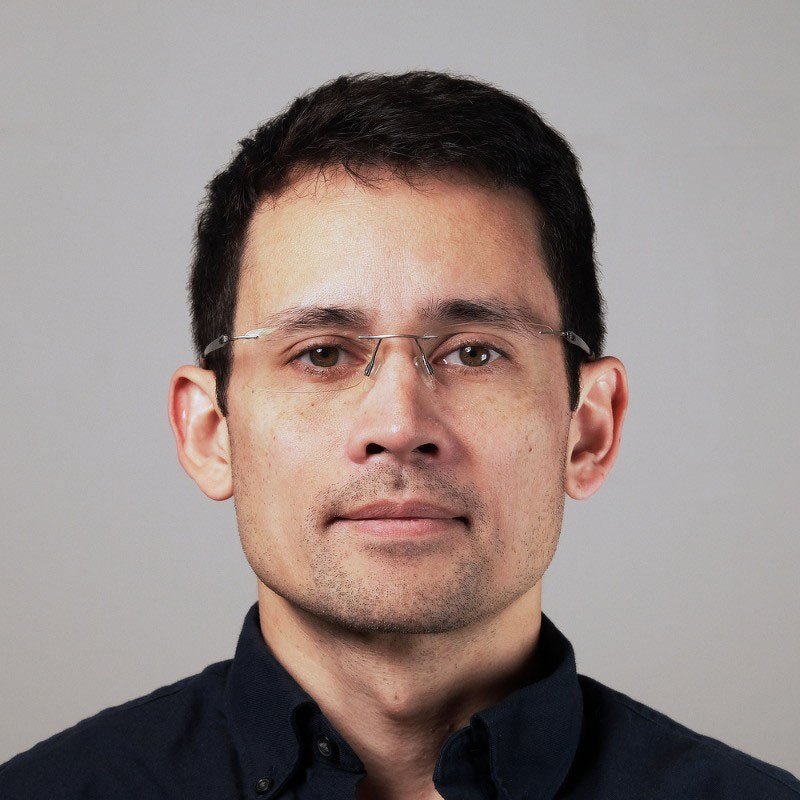
William Martin
Director of AI at Consensys
Adj. Prof. at Columbia.
Co-founder of Spatial Pixel.
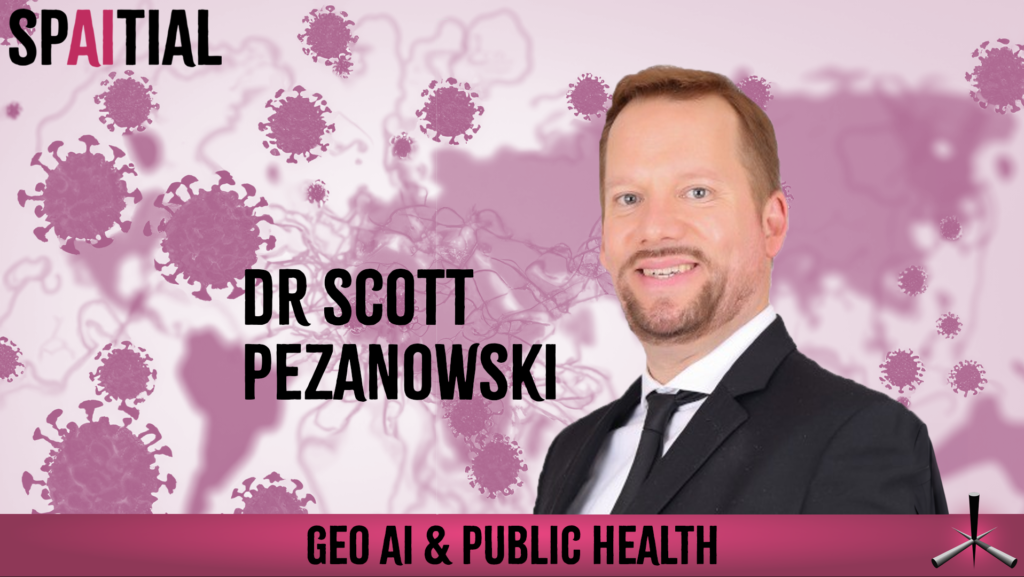
AB
Well g’day and welcome to SPAITIAL. This is the inaugural episode of a guest interview. Inaugural. Am I going to already question why is that word ‘inaugural’? Can you have the word ‘augural’? Anyway, it’s the first ever guest interview.
I’m joined this morning to my right, Helena, there in New Zealand. Good morning. How are you faring?
Helena
Yeah, good. Thank you. And very welcome to Scott. We’re really pleased to have you on.
Scott
Yeah. Yeah thank you so much. Yeah I am really, really happy to be here and actually honored to be your very first guest.
AB
The very first. In this country we’d call you the guinea pig. All good, there’s nothing to be worried about.
Only the two of us having a chat to you, we haven’t got the full team. We thought it might be a bit rude to have five people asking you questions at the same time. So there are two us here this morning, loving to having great chat with you. But look, if you have a few seconds, can you tell us a bit about yourself, a quick bit of your history, but also what you’re doing in that part of the world?
Scott 01:36
I’ve had a long history in the geospatial field. It’s kind of like kind-of weird how it all just worked out this way.
Even when I was a kid, I’m a maps guy, always staring at maps. Also, too, I am always kind like spatially aware. I always want to know what direction I am facing. There has been times in my life like you get out of the subway in New York City and you don’t know quite which way you are facing and it’s really disconcerting for me.
So anyways, you know, I have a long history in geospatial. I got my bachelor’s at Penn State University. After there, I worked at MapQuest .com for two years. Then I went, got my master’s at University of South Carolina.
So I kind of like made my way down the east coast of the U .S. Um, then, uh, so I did my master’s on – I always say like at that time, I don’t know what you’d call – like mobile mapping? So this was a very, very early – *early early* stages of GPS enabled smartphones.
This was the prehistory of the Palm Pilot, 2D mapping. So there was 100 by a 100 pixel black and white screen. And I was able to put some imagery on that so it was kind of cool – like it actually worked and it picked up the GPS location and showed me you know based on where I it showed you how really you know images that you could kind of make out water bodies and stuff so it’s kind of cool so anyways then after that I worked for Esri so when I when I was getting my Master’s I took programming course Java at the time and And then that kind of helped me, also too, I wrote this application for my master’s in Java that helped get this job at Esri as an application developer in the DC office.
Scott 03:34
That was a really exciting time, working for some really like exciting clients there. And basically after that, so I went down the East Coast and then I came back up the east coast to my hometown, which is in my Alma Mater.
I was a researcher then at Penn State University, my alma mater, for basically like 15 years. I worked at a research center there called GeoVista Center. It was really a wonderful job. I love this job!
AB 04:03
Scott, what years are these? We’re talking late 90s by this point in time, or 2005-2010?
Scott
Yeah. So, so I started there – GeoVista Center – started in about 2005, Spent about 15 years there, also in the process. So that was great because it was, the GeoVista center was multidisciplinary.
So we were based in geography, but then we had so many collaborators from, next door to us was the College of Information Sciences Technology. We had computer engineering collaborators. We have landscape architecture collaborators, sociology collaborators even towards the end, a lot of folks who were handling some of the big data.
We kind of like a umbrella organization called, what was it, ICDS? What is it? Basically, it was of promoting big-data & data science. So we had a lotta amazing experience and I learned so much.
Basically, it was of promoting big-data & data science. So we had a lotta amazing experience and I learned so much. I was really blessed to be around so many smart people with so many creative ideas.
AB 05:11
So 15 years of research in the mapping in the geospatial world, that’s a phenomenal length of time. You said research as well as teams coming in, your background at ESRI must have given you a front row seat.
Were you pushing technical boundaries or were you pushing customer questions? Were you sort of trying to go into uncharted territory? And I mean that in a bad way with maps or you trying actually do larger data sets and get ready for a more public mapping world?
Scott
Yeah. So basically all of the above, I’d say. And you mentioned about sorta my experience at Esri and it was kinda like once I came, it was like, so we had this really fascinating project. It was the very first project I worked on.
This was, you know, keep in mind, again, this was like, maybe what, 2005 or something like this. So we had this, it was we were doing a collaboration, NSF funded, but then we are doing a collaboration with Port Authority of New York, New Jersey for like crisis, the, you know managing large scale crises.
They basically handle all of the security for the the area of the New York metropolitan area. So then we were building this system where it’s a large screen display. And at that time, we had this camera that recorded people’s gestures and speech.
And basically, you would point to this large-screen display and you’d say something like, there is an event here, please draw me a buffer around this point, this area here.
And so it was really kind of fascinating that basically when I came in, boom, right away, I’m kind like building the mapping interface for this.
So it’s really kinda cool. And it just, so right now, like large language models, super, super popular, everybody’s talking about it. So, it reminds me of the missing part of that particular project.
So, back then, so the speech recognition was really crude at the time, and also too, you would have to use really precise words and then once you would say something, it would recognize the speaker and it was kind of just like we had a whole bunch of sort of like if -then -else statements. If the person said this and this, then perform these actions.
AB 07:44
You were almost saying code, like a “go north”, “open this”?
Scott
Kind of like hard coded, yeah. But now I feel like, with large language models, this can easily interpret now what kind of a natural language, what you’re trying to say, and it can even give the instructions on doing the command.
Maybe the only missing part is like kind what people are working on now is connecting that large -language model to to action performing functions. So, yeah, so anyways, I’m telling this story, but so I don’t know if this answered your whole question, but basically then to summarize the way I felt about this research was we had our funding agencies coming, you know, the various funding industries like we had NSF, you Know, National Geospatial Intelligence Agency, DHS, but we have a project for centers for Disease Control and Prevention, CDC.
And basically, they kind of like have their problem. And it was kind like our job to try to figure solutions for their problems. So it’s like really super like, you know, stimulating and I love the, I loved all the meetings with, once again, like I was really blessed to be around some super smart people at the time.
So I learned, learned a whole lot.
AB 08:59
Absolute joy to beyond the leading edge. I mean, to being in a place where that was the question being fired at you, every alternate week, would be quite phenomenal. Can you look back at that? I mean, 15 years is a long time – a gold watch comes at 10 years? I can’t see a goldwatch on your wrist right now, but that’s okay – 15 years is a lengthy bit of time where you’re not only cemented yourself as being an expert in your subfield, but that whole team must have just been a go-to for, I take it, bigger and bigger questions.
Were you starting out with the vocabulary from the customers of, can we do this? Yes, we’ll show you. But, towards the end, it was like, you know, how big a question can you ask? And were you taking larger and larger chunks and steps and going from regional to nation -based to what I’m assuming now is a worldwide view of some of these tasks?
Scott
Yeah, so this is an interesting question. So there’s a couple of points about this. So one, we’re supposedly the experts. So for when we’re guiding them in a “this is what we think we should do”. And then you kind pointed out at the end, in a sense, one of the reasons I fit in well there was I had a lot of experience with web programming at that time. And that basically, and then in the process, I learned databases, we had projects where we were collecting millions of tweets at a time.
So I learned all this stuff about how to handle big data. So, I was pretty good at then scaling up the projects. We’re going to first focus on a particular area, but then, okay. Now we want to scale it worldwide. So this was something, and then another point to this is, you were saying about how far do you go? When you do science, you always got to be really kind of careful because the more you’re kind of bringing in much more variables and then when you come to your conclusions then it’s a little bit harder to say with confidence that you’ve kind of done what you did.
This was always like throughout the process. It’s always “let’s try to keep a focus on this”. If we accomplish something else, it’s cool – but try and keep the focus of the main problem because this is the actual problem we’re trying to address.
AB 11:30
Yeah, gotcha. So there were ‘bright shinies’ everywhere. You probably had a blackboard or a whiteboard of things you would like to tackle in the future. But every task you were being put forward, you will try lock in most of those variables and just focus us on the task at hand, here and there and take slow strides, but in looking back, you took large strides towards making maps that, you know, really are there for larger teams.
Scott
Yeah, and so this is something like it makes me, you the way you’re talking, it make me think of, it didn’t always happen that way because for example, I mean, at that time I was doing a lot of coding, right?
And now at this point in my career, obviously, you want to code for a purpose, right? You want to solve an end goal, you wanna solve and end real world goal a lot of times.
Nowadays, looking back, there was many, many times, I kind of did stuff because I was like, this is really, really cool, you know? But in the end, it didn’t really serve that purpose.
You know like an end goal. So, oh, okay, this is really cool to do. But we really need to keep focused on what’s our end goal? What is the problem? The real world problem we’re trying to solve. And so this is another thing, like in the process of while I was a researcher there, so I mentioned that we were based in geography department, but then we had collaborators from next door, which is the information sciences and technology.
Now, I think it’s still called the College of Information Sciences and Technology. It’s multidisciplinary itself. My degree is actually in informatics. It’s multidisciplinary itself. But one thing I really, really liked about them, they were focused on using technology to solve real world problem.
So this is something that was really ingrained in me, even in my PhD is you really got to keep a focus on: who’s your audience? What is the problem you’re trying to resolve? How is it going to benefit them? How is going benefit society?
Helena 13:39
Yeah, I think especially in geospatial, there’s a tendency to be very technology-driven. So I’ve seen it referred to as like the ‘geospecial product trap’, where people build cool applications and do cool things with the technology, but it’s not really addressing a real -world problem.
And so then you’ve got this tool or you got an application and you realize no one actually needs it because it wasn’t actually problem driven, it was technology driven. And I like how you really come from that other angle, Scott, which is, you know, a breath of fresh air, especially in this like, heavy technology field that you’re operating in with the AI and the geospatial, but you are saying, let’s not be driven by the capabilities of the technology, lets be driven the problems that we can solve.
I think that’s a really powerful position and it’s really a position where you’re going to be able to help so many more people than if you just kind of get hung up in the technology and think like, this is all the cool stuff I can do and I could do anything for anyone. But then it is not really actually focusing on anyone’s problem in particular. So I know you’ve been working a lot in health field, I don’t know, do you want to get into that a little bit and tell us how the story continues from after your time at the GeoVista, was it, to what you’re kind of doing now and more kind of how you are bringing together these different crowns that you’ve got like the applied research strain and the geospatial strain in the AI and how your weaving all of that together.
I’d love to hear a bit more about that.
Scott 15:22
Yeah, yeah, certainly. So like I said, I really loved my time at Penn State. So many nice memories, so many great colleagues that I still keep in touch with. Some amazing people, maybe I should mention actually, some names.
I don’t know if any of y ‘all have ever heard of Alan McEachern. He was basically my mentor. And one thing I, so there was lots of things I loved about Alan, but one thing in particular. So he began his career as a cartographer. He got, you know, he got his bachelor’s master’s PhD in cartography, and then he became one of the world’s leading figures in Cartography. But then as he progressed in his Career, technology started improving.
And he just really, really he was a lifelong learner, which is something I took from him, You know, I took it to heart really and I feel like I’ve kind of done this in my career, my crew as well as like, you know. Whatever the technology comes I learn it so despite the fact that he really you know we would be in meetings and he’s talking about these programming concepts.
He’s talked about this data base issues he in Then towards the end of his career He even became so qualified that they became an adjunct like i said at the neighboring, um, you, know college of information and sciences and technology so So anyways, maybe another guy, a couple other people, Anthony Robinson, he’s amazing guy.
He’s head of their now the Penn State online, not only as a professor, but online learning. Was it master’s in GIS for online program? And then Brian Tomoshevsky, another, I feel like I don’t know, Maybe I shouldn’t have named names because now there’s so many I’m going to leave out.
I can’t forget about my advisor for my PhD, Prasandra Mitra. So this is kind of another thing. He came from the opposite way. So his degrees were in computer science. He got his PhD from Stanford in either computer science or computer engineering, complete. You know, what do you say? Like a tech geek, and then he worked for Oracle for a while. But then he so he came from other perspective that then He learned so much about some of these geospatial issues and now he’s like, you know, writing papers and all about geospecial problems So it’s this is what I was telling you about.
This multi -disciplinary environment was really wonderful. I left there with mixed emotions. I basically left there for to marry my my now wife – and hence why I’m in Kuwait. This environment really set me up that now I just want to continue challenging myself, trying to solve real world problems.
Scott 18:16
So you know Helena, You mentioned health, and technology towards health. So there was two, let’s see, two maybe even three projects while I was at the GeoVista Center. So we had one for the centers, the CDC, Centers for Disease Control and Prevention.
We built for them both the Pennsylvania Cancer Atlas and the – in turn, it was like the U .S. Cancer – we modified it, US Cancer Atlas. So this is like an online – AB, I mentioned to you about the action script in Flash. So it’s built in flash and action scripts. This was that time.
How do you present the data – amounts of cancer rates, but then also all of the demographic data? How do you present it in a way to a user that they can understand it, they can filter it they, can search it and, and try to gain knowledge from, from the visualization?
Then we had another one, it was called Health Geojunction.
This was taking large amounts of online media, news articles. So basically like news, articles, extracting place mentions, key topics, what are the topics and trying to then map. map. So doing some geo-parsing, basically.
We’ll extract the place names, put those place names on a map, and then providing, once again, a visual analytics system to let people visualize where are the diseases happening, where the connections going on between various diseases, the placements that are co-occurring.
And then finally too, there’s one other, so a project I worked on for quite a long time, it was called Senseplace 2 & Senseplace 3. So this was a really interesting part. It actually, it really successful.
Recently we won this award for one of the paper from IEEE. We wrote a paper. It was amazing that we got recognized – it was called the Test of Time award.
It’s 10 years since we wrote this paper about our research. It was kind of like whoa, I can’t believe it has been 10 years. But basically, this project, there was kinda like it stood the Test of Time.
It gets cited a lot, and it generated a lot of discussion. So what was about kind have a similar concept about analyzing text.
So at that time, Twitter was getting really, really popular for things like people would talk about all of their societal problems on Twitter. We built a system that was really designed to extract meaning from this incredible amount of Twitter data you would get. We focused on crisis management, but then really it could apply to almost any domain.
Scott 21:36
It can even apply to businesses these days, like a large business wants to analyze, okay, our customers in this area, how are they responding to our products? How are their responding our competitors?
Things like this. So it was kind of interesting. So basically, given my background, multiple projects focused on health, basically technology towards public health, you know, improving public health outcomes.
So then recently too, I got a project, I was working with the World Health Organization. So this was once again, so this was some folks based in the African office. Congo is the regional office, so basically their, what do you say, domain is the vast majority of the African continent, it, excluding like some of the countries in the north, Egypt, excluding Egypt Libya, a couple of countries.
They had this data set, I forget exactly what it but basically, it’s a case. Oh, yes, yeah, it is. It’s comprehensive. comprehensive way. When when you hear data scientists saying like good, you know, you really need good data.
They had good data, so this is a really good good thing. So it’s basically like suspected case counts and death counts for almost all of the diseases that the infectious diseases that go on in the continent for every week and for every district.
So not at the country level, not at state level but at district level. Kind of equivalent of a U .S. county. And so we did some exploratory analysis and we, you know, we did spatial autocorrelation. One thing was, like, we were able to find different, all of them had a strong spatial correlation.
So it’s not really not, it is not hard to kind of like think about this, that if you have cases in your neighboring district, there’s a good chance it will affect you. But what we were able to do too, is quantify it.
For the different diseases, there is a different amount. And so we’re quantifying it, quantify the differences for diseases. But then also too after you do this, you get to local spatial or auto correlation.
When you’re getting your hotspots, your cold spots, your outliers. If you’ve got a cold spot, but then you got high district among them. So it was really, really neat kind of work. I don’t want to talk about this too much because we got a paper still in review.
AB 24:30
Well, that’s fair. We can’t talk about something that hasn’t quite hit the airwaves yet. But just to roll back a second. So everyone knows at the last few years, the dreaded R value, you know, the COVID years have taught us to watch that number like a hawk.
Was this research actually trying to correlate not the virology anyway, how bad the viruses were, but the speed and the density and trying to actually put time and space to that R value, was that kind of the focus of that research?
Scott
One focus was the spatial distribution patterns. The factor is that we chose the model. For example, we choose precipitation, temperature, population density. There’s a couple other ones and mainly because like for one, they, oh, what is the population near a water body?
Scott 25:28
Because water bodies are very important for disease transmission, either directly or indirectly. So then, so what are the most important factors for the various disease, for this spread of the diseases?
What are the most important factors? Then the other thing is making a prediction. So making up prediction on cases, based upon these criteria, these factors, precipitation, temperature – all of these… will your district have cases or not?
Scott 26:07
So this was the thing basically making a prediction. We were able to do really quite good. I’ll have to be honest with actually, predicting the number of cases, we didn’t do so well. This is like, you know, if you’re familiar with machine learning, this kind of like the, it’s called regression. So predicting actual number cases. It’s a little challenge. And to honest too, I mean, we’re doing this over, obviously the African continent’s vast, you know, vast.
It’s really not surprising. But when we turn this into a machine learning classification problem – are there cases or not? – then we started getting really good success.
It’s a little bit setting up the machine -learning problem a little differently, than we were able to get really success, basically in the goal of, So, if you’re able to predict cases or not, kind of at that local level, but over a large geographic scale, and on a weekly basis, in a matter of milliseconds, then you are successful at this, it will be really powerful.
AB 27:24
That answers the question I was just going to ask. Was this work to actually try and get close to real time and be of use tomorrow, the next week? Or was this one of those deeper questions of let’s look at previous years data and make broad sweeping recommendations for next year’s possible viruses?
Was it short, sharp and in this case binary, or was it that long running question that you had time up your sleeve?
Scott
Yeah. So the model we built, I mean, it’s more about the near term. Also too, when you do machine learning and you create a model, if you want that model to predict a long time in the future. I mean, are things change or environment change, things like this.
So you kind of got to update the model as you go. You got a retrain and all. So basically it was intended. The model that I built, it was like, you know, say, for example, if you have if you have OK, next week, it’s going to be raining in this rain prediction.
You know you have all these variables, you have a prediction, then you’ll be able to predict case counts. And basically, with a little bit more work, I wouldn’t see any reason why it wouldn’t be successful like a month in advance, two months in events.
AB 28:35
It feels like the global weather forecasting trend, by means every nation has their own body that looks at the next five days, seven days. There is a growing trend of worldwide weather models and look, they’re not always better, but sometimes they are surprising.
But it’s just that concept of extrapolating up from a large area to the world used to be impossible. We definitely won’t be doing that, but that’s now becoming potentially tangible. So yes, when you’ve got a graph database, a chart of the whole world, potentially one day there might be someone down the track who decides, “hey, let’s go for broke and do the whole world in one fell swoop”.
Scott
You touched upon the value of our work. I mean, it’s not like what we were doing was really groundbreaking in itself to predict disease, but the fact that you could do it at scale and in fractions of a second, I mean this is what’s really kind of like the key point to it.
And it is not just simply like a prediction, and it isn’t just, oh, we’re going to predict this, what you can then do is give a prediction but do some modeling.
Helena 29:49
So just to clarify a little bit, Scott, because I guess not everyone listening to the podcast come from the geospatial background, since we’ve kind of got also the scale on a different scale when we’re talking about the indoor navigation, robotics, the architect, and so on.
So from a geospecial perspective, we have obviously got those different data layers that you’re talking about. We’ve got the geography, so we’ve got water bodies, and we have the human factors, we got different populations, and the layers with the education level.
So we have all these different layers that we’re layering on top of each other for a typical geospatial analysis. Then you are bringing in the AI component. And so, can you explain a little bit maybe about where kind of geospatial starts and ends in the AI takes over?
Like, is it that you’re basically, you can, your modeling the as is with typical geospatial methods, and then the machine learning AI does the predicting, or is there not a clean cut like that? Or how do they play together to go beyond what’s possible with like, pure geospatial capabilities?
Scott 31:09
Yeah, so this is an excellent, excellent question. So I’ll kind of talk a little bit about the current state, but then I will kind of mention where at least some people think things are going. So right now, I mean, most of the, like when we do geo AI, we love to stick on this geo in front of everything.
So, maybe it’s like lack of better term, ‘Geo, AI’. I don’t really like it, but I don’t know what else to call it, but in the end, the AI model is the same as everybody else. We’re using RNNs, CNNs. All of this stuff. It’s all the same.
Also too, for some of the challenges like computer vision, doing machine learning on satellite imagery, it’s basically some of the same models that you’re going to do computer vision on on regular pictures, really not too different.
So for my all kind of comment about the project, you know, this particular recent project is so for the geospatial part, I used a lot of geosphere spatial part for pre processing for. For one, for one the spatial autocorrelation, the special hotspot analysis, Because to be honest, that did lead into us choosing some of the factors that we did choose.
Also too, for example, I mentioned, what’s the population near water bodies? So that, you kind of did like some, your standard way back buffers and overlays, overlaying, getting the precipitation, but then bringing in the waterbodies from open street map, doing a buffer around them and making some calculations.
In the end though, all of this sort of geospatial processing, in the end I had kind of like a structured table. The result of this was a structure table, so it’s like basically for every district for every week, we had the number of cases, the amount of the average precipitation, the average temperature.
So it’s like basically a Excel table kind of in a sense. And so then the AI came in, I treated it basically as a tabular type of AI model. So one thing I also considered, but we weren’t able… I didn’t try this. I wanted to try this. So I treated this particular problem as, you know, like I said, trying to get things into a structured table treated as that type of machine learning problem. But in my mind, if some of the data… No, actually, it went down to the disease data, the actual disease. This was aggregated by district. However, in my mind, if we had a little bit finer spatial resolution, similar to the temperature data, the temperature datasets precipitation, this was basically from your NASA, you know, satellite data.
Scott 34:29
If we have the case counts, but on this kind of like resolution or similar, then you could actually treat it as a computer vision problem. So this is kind like, I’m trying to to describe for this particular project, how the geospatial and AI fit in.
Now, kind of like for the future, so I’ve heard a lot of talk in the kind of geospaceal, geo AI is getting like AI models that inherently no space. So for my, once again, my particular case, in being in this structured table, it really, maybe the model could learn Um, this particular district is near this, but, but it wasn’t very clear. If I would, if I were to treat it as a computer vision issue, uh, a computer version problem, maybe it would have been a little bit more spatial where the model is able to know the, you know, the pixels around it at all. But, but I think what, where some of the AI models are going is being able to inherently know geographic relationships. relationships, not only nearness, but some of the other geographic relationships that all of people in the geospatial…
Going back to what is it, the first law of geography, which is… Even for this WHO project, it was really… It applied when I told you about spatial autocorrelation is that, you know:
Paraphrasing the first law of geography is that everything is related to everything else in the world, but near things are more related to you.
Scott 36:14
And so if we have AI models that kind of like, account for this, this natural, then I feel like they’ll be able to solve a lot of problems.
So doing it the way you were using the GIS world to prepare your data for a regular 2D data set and that gave you the results and the tools and leavers for your clients to pull to be able to test and use different scenarios and what could you call that a good 90 -something percent, excuse me, a 90 something percent answer which gave people confidence, the ability to play.
AB 36:58
You’re thinking ahead to different modes, different ways to increase, you know, one more signal level, one more level of confidence in a data set to incorporate richer data, more variables. Is that all on the drawing board? Is it a wish list? is that you’re going to pass the can to someone else in 10, 20 years and they’re going solve it. How soon can you see these sort of problems going from that regional level down to the next level of cell?
Scott
And another thing just too, which was valuable from this machine learning. So machine learnings, many of the models, they produce what’s called feature importance. So how important was that particular factor in to making its prediction?
So this was another thing that that we were kind of so it’s not it doesn’t always so it basically you got to keep in mind that this was for the machine learning model, not necessarily the real world.
But it gives you really, really good hints at what are the most important factors for making that, you know, out of rainfall temperature, it quantifies the importance. So that, once again, gives you a lot of information on a more knowledge on what you try to tackle.
What are the factors you really try to address first?
Helena 38:21
I’m also really curious, Scott, about how soon you think that the geospatial, sorry, the AI model that knows space or that understands geographic space, How far away is that, would you say?
AB
Go on, make a prediction. This will be in big bold text on the episode post : )
Scott
I don’t know, please! No, no, I’m not going to pin myself any, uh, I dunno, when things are going, all I can say is probably faster than I think, actually.
Helena
And what, what problems still need to be solved for that to become a reality? So I know we’ve talked on the podcast in the past about like the issues with AI and space in general, But in the context of the geographic space, I would think there’s a lot of specific rule sets and so on.
But what are the hurdles that remain until there is a model that can actually do that? What’s the big missing piece?
Scott 39:19
To be honest, I don’t know. I feel like I can’t answer that question. So basically, this might be a question for, so while I was at Penn State, I was also around some experts in what you say, like spatial cognition.
How do people think spatially? How do you people understand their place, where they’re located? Things like directions, wayfinding, things like this. So I would say you get those people and you let them talk to the actual people building the algorithms, and then you can find sort of the missing piece maybe.
Helena
Yeah, it’s a really hard one, isn’t it? I mean, I know I focused a little bit on my research about formalizing place, you know, as in like how humans experience space being like places. We don’t think in coordinates.
In terms of places, we think, in terms of neighborhoods. We think of, kind of personal reference spaces and that’s really the incredibly hard concept to turn into zeros and ones as you need it to be able to kind of um teach it to a GIS system for example.
So it’s just one of those big problems where personally I always thought without knowing so much about how the AI works in that sense that potentially large language models might give us a new avenue to explore that concept.
You know you mentioned before with the Twitter, the sentiment analysis, I don’t know if that kind of large scale language analysis of place sentiment could potentially be an avenue that would help formalize the concept. Do you have thoughts on that?
Scott 41:09
Yeah, you’re really, so like I said, one gentleman, Alex Klippel, now he’s at a university City in Germany. He was originally from Germany, he came, he was my colleague for a long time at Penn State. Now he’s back in Germany. I think now though, it’s kind of like getting into virtual reality. But he had a lot of this, you know, kind like what is place also to some other folks that came through. Brian Tomaszewski, now he is at Rochester Institute of Technology.
So when we went, yeah, exactly this when we were doing this sense place, that’s the name actually, the name kind of came about. Actually, Brian Tomaszewski was the one who kind of coined it, sense place.
And then we he did SensePlace 1. And we, I mean, together with us, but then it was like, then we built sense, place two, since place three. But it’s kind of like we had this, that was one of the overarching things, I’m not sure we ever really kind of, like answered any questions towards that.
But this was, one thing is that if you get a whole lot of text, and people are talking about places, they’re talking about other things, and you’re starting to get in natural language processing, sentiment analysis, emotion analysis.
Some of the other natural light language, processing concepts, people mentions, you know, named entity recognition. So people mentioned disease mentions you know whatever the name density is. You we always kind of like had in the background minds, this is a really good opportunity to kind of like try to conceptualize exactly what you’re talking about.
How do people define plays? And also too, like, you know, we mentioned this earlier, a lot of the research in this area is at a really, really fine level, doing things like user studies, a very narrow population.
Scott
But can you scale this up? So can you scaleless to the world, some of those ideas. So this was something, yeah, that was… We talked about it a lot. I’m not sure we really kind of tackled it directly, but in my mind, this is a really good opportunity to use big data to try to understand how people actually conceptualize place.
Helena 43:30
Yeah, absolutely. And I think it’s also interesting that you mentioned scaling it out to a world level. I think that there’s a whole lot of other issues wrapped up in there that, you know, possibly people don’t really think about, like, the different kind of cultural impacts of language.
I don’t know. Perhaps, I mean, if you’re living in a different culture, I’m sure you’ve observed that people talk about things quite differently in different cultures, like places. The concept of place is very different across different cultures.
And so what happens when we analyze these, you know, big kind of English texts is quite easily that we kind paint a very Anglo centric world view of what place looks like without really kind recognizing that cultural nuance.
And I think there’s always that danger. And potentially with like this kind of large scale analysis would even have to look across different languages, across, you know, different cultures and really create something that brings in more than just the English.
Scott 44:43
It reminds me of another project we had. So this particular project was focused on wayfinding. And actually, I think Alex Klippel was on this project. So he was really interested in way finding.
How do people get to where they want to be? How do they, you know, kind of like do it in their minds. So how, for example, in the US, we have we have our MapQuest route directions. And it says go go on this street, take a right, you know.
Go down this distance, then take it right. Sometimes we use landmarks. But then, then like I said, Prasadja Mitra was on his project, he’s originally from India. He’s like, yeah, We kind don’t do that there.
You know? He was kind like it’s all about landmarks, you know, it is all about landmark there. So, how people, even for a task like this, this is just a small part of place, wayfinding, is extremely different depending on where you live in the world.
In Kuwait too, they have street addresses, but honestly they don’t use them much. So they have like a actually it’s really I like their address system. It’s really kind of intuitive. So, they, have although honestly it is a small country, so it, is it a little bit easier to manage.
SBut, basically they have the, they have Kuwait City, the areas. So Kuwait city, The different kind of suburbs, Kefaun, Haldia, but so, so basically you say, you would say something like I live in Kefaun and then Kifaun is divided up into block.
Every area is divided into blocks. So I go to Kefaun, I lived in block 2, and I’ve lived on street 64, and live on house 2. So this is say your address, kind of. You wouldn’t say it kind like a US way.
But honestly too, it’s really, and then the street signs, all of the areas you’re trying to put a little map on. You’re drawing on this road, lock two, here’s block one. It’s kind of like, I think they did a freaking job with that. They lit out the roads too actually, the highway system really well, because it is kind like you’ve got Queen City, you got First Ring Road, then you got Second Ring. Then you have Third Ring road and it kind just goes out.
And then also too you’ve got like these spoke highways going out which is like what is it 30, 40, 45, 50. It’s really kind of intuitive but this is all of this kind of stuff is, like you said, it’s like really, really different depending on where you were, where are you in the world and one.
Scott 47:34
So one thing I learned from my really really smart colleague at Penn State was everybody everybody plays differently you know like there’s so much variability in how you you kind of like are you a spatial person?
I gave that example before about like if I don’t know the direction I’m facing I feel like uneasy because I am a very very spatial person.
– I used to play a lot of golf, and I remember playing one particular golf course where there was like two holes and a very windy way through the trees and you couldn’t see the others a lot of times. You can see other holes but I never played it before and I didn’t know what direction I think and really uneasy the whole time – I felt was really kind of like something just here because I don’t know where I am I also have to know where am and what direct which I’m really I feel like I really good at.
When I hear my father-in-law, he’s like, “how is he even going to get around Kuwait City”? It’s really no problem for me.
AB 48:48
Very nice. Yes, Scott, I do share that same point of view and I wonder actually if that’s common or it’s uncommon. I generally know which direction I’m facing vaguely and it is really nice to be able to if I am ever riding or driving somewhere.
Going somewhere for the first time is a regular normal challenge and the Melbourne thing is which car lane do you have to be in five Ks before you get there? That’s our normal problem. But I’m always fine the second time, which is always a nice sort of vibe.
But I must give a shout out to my cousins who were sailors. I can tell you right now I am kind of facing South – but my cousins were crazy wild: they knew they were facing “south, south west”. And it’s like, okay, that’s a level of a distinction that is a super power that you need to try and find a use for!
Scott 49:33
I’m much more reliant on Google maps. It was like before it was, like once I drive somewhere, boom, I know it all, you know, I would drive from Carolina, from Pennsylvania, South Carolina, no problem. I want to drive 10 hours. I don’t even need to, you don’t know directions. I know where all of the basically the turns – but now with Google Maps, I feel like it’s taking me longer to know the route and I am using maps longer until I actually know to go places.
So I think like I’ve a little bit too reliant on the technology. Maybe yeah,
Helena
That definitely happens!
AB
Scott thank you so much for your time. Absolute pleasure to have this long chat with you. It’s been fantastic…
Look, your application for Unpaid Intern at a SPAITIAL has been accepted, but we’ll have to get back in touch with you whether you can fill the role… But your qualifications… only just pass. To be honest : )
Scott
Ha! I love talking about this kind of stuff. I like talking this stuff, and also too, it’s I learned that you’re doing… You touch the part, or you are a little bit more focused on the spatial part versus I’m little bit more geospatial.
But honestly, I feel like what you’re doing here with SPAITIAL, combined technology, combined with AI, and I mean, I love talking about the kind of stuff. And also, another one of my, what do you say?
You said about something ‘a touch controversial’ earlier. This is one of mine controversial things, is that I think in the geospatial world, we play up the importance of the geo-spatial part a little bit too much, I feel, because I think like so many of those problems could actually be solved if you treat it as Cartesian space.
You know, instead of like having to deal with the curvature of Earth, all this stuff, maybe this is something like the geospatial people, they want to, you know sometimes a little more and a lot, don’t get me wrong, in a a lot of cases is really, really important.
AB
Oh, for sure. There’s always the purists versus the pragmatics.
Helena
And then there’s always a ‘spatial specialist’, isn’t it? You guys heard of that? The spatial specialist. It’s kind a of a geospatial thing.
Scott
I remember when when Google Maps came along, and I was you know, I was in in geography in all and oh my god, how could they possibly use Mercator Project? Oh my gosh, like, you know, I mean, I understand Mercators Projection has, has its problems, but you know because they were able to treat, basically treat the world as X, Y, they, were doing able, to do so many amazing things because they simplify the problem and then they’re able to, do some amazing, so this is kind of just one example, I feel like.
Helena
And the vast majority of Google Maps users probably don’t even know. Yeah. It does. I think most users of of google maps, they have no idea about projections anyway, so it’s really kind of, you know, back to what we were saying before, it solve a problem for your user and they don t care about, projections that needs to be abstracted under the hood, which Google maps has done a fantastic job of.
Scott
Most of the time when you’re using Google apps, I mean, you are looking kind of like local, so you aren’t really even going to notice the difference. I hope I didn’t upset any of my former colleagues when I made that comment.
AB
I tell my teams all the time that there are no Oscars or Emmys for coding. Yes, there probably are a couple of awards in deep dark places on the internet. That’s not to be a cop-out – but if it works and it’s reliable and you can sleep at night and it’s elegant, simple, and does the job… the job done.
We can solve some gnarly problems with a lot of code and as long as we’re okay with it, that is the job. So it is great to hear that pragmatic sense coming through.
Scott
You and I talked about this a little bit yesterday. Both of us kind of like having coding in our background. It’s like no matter what, you’re going to have some issues. So you kind of got to like pick your battles.
So what are the really, really major problems? Am I able sacrifice speed? Am able the sacrifice something just isn’t quite vital, but I am able solve a bigger problem?
AB
Brilliant. Well, Scott, thank you so much for your time. We’re going to stay in touch. And by all means, we’re gonna come back when that paper of yours is announced and ask a few more questions about it if that’s all right.
I know we are leaving the audience hanging. Come back for the next exciting episode of “will Scott be able to talk about his forthcoming paper?”
Bye!
Scott
Masa lama.
To absent friends.