In this episode, we list out the range of ‘megatrends’ and emerging patterns in the field of spatial AI that we’re are closely monitoring. Key topics include the rise of large multimodal AI models, the potential of agentic AI systems with specialized agents, the emergence of ‘mixture of experts’ architectures, the integration of spatial data and sensors with natural language processing, and the contrasting trends of high-end immersive technologies versus low-sensory ‘dumb phone’ experiences.
We show the widening gap between open-source and commercial AI models on our spatial.space AI/ML model timeline, speculating on factors driving this divergence and potential future developments.
Overall, the discussion centres on identifying and analysing cutting-edge advancements in Spatial AI, with a focus on their implications for a range of industries and applications.
We’re also publishing this episode on YouTube, if you’d like to watch along, and see the outlinks & screensharing *with your own two eyes*: https://www.youtube.com/watch?v=UYDI4qfAreA
Chapters
00:02:09 – Defining the Field of Spatial AI
We talk about the need to define the field of spatial AI, which encompasses spatial computing, geospatial data analytics, depth perception, and AI techniques. We showed spaitial.space – a 3D graph/map showcasing the interconnected fields related to spatial AI – acknowledging the amorphous and rapidly evolving nature of this domain.
00:08:28 – Emerging AI Model Architectures
William discussed three leading contenders in the design patterns of AI models: large multimodal models (eg GPT-4), agentic systems with specialized agents, and ‘mixture of experts’ architectures. He analyzed the potential advantages and trade-offs of each approach, emphasizing their accessibility to developers and potential for enabling new use cases.
00:23:05 – Integration of Spatial Data and Natural Language Processing
Violet shared an example of a company (Architect AI) working on translating sensor data and physical world information into natural language. The discussion explored the potential for integrating spatial data, sensors, and natural language processing to enable better understanding and control of real-world environments.
00:28:11 – High-End vs. Low-Sensory Experiences
We discuss contrasting trends in user experiences – with high-end immersive technologies like Apple’s Vision Pro on one end, and the ‘dumb phone’ movement emphasizing low-sensory, context-aware experiences on the other. The potential implications of these divergent approaches for spatial AI were explored.
00:32:55 – Open-Source vs. Commercial AI Models
The widening gap between open-source and commercial AI models was examined, discussing factors such as the high computational costs of training large models, potential government-backed open-source initiatives, and the potential for specialized, domain-specific models to emerge alongside generalist models.
Transcript and Links
AB
Well g’day and welcome to SPAITIAL. This is Episode 17 coming to you, I think, more towards the middle of April. Time will tell. The reason why time will tell is that it takes, well, this will be episode 2 of our video feed, which takes a little bit more than just the regular lunch break to get out to the world.
So, surprisingly, it’ll probably be hitting you around the middle of April. That’s okay, should be great. We’ve got with us today, we have Mirek, and Violet, and William, and we’re going to go on a fairly gnarly topic this morning, today?
Time zones, I must say, are hitting us quite hard. It’s now not daylight savings in Australia, it is in North America, so times are all around the world. Right now, we’re going to be talking about, on today’s episode, there you go, date relative or date inconsequential.
We’re going to be talking about megatrends, the kind of things that we’re actually keeping an eye on. Some of it is quite active, some is quite passive. The other thing is just the things that really stick in our attention, the things that whenever news breaks or topics emerge, we are literally on it, things we stop, drop, and read to make sure that we’re covering that topic and making sure that it really is something that we want to be watching out quite well.
By megatrends, I am talking about things that are not just about what’s happening next week or next month, but really the emergence of the large trends. Some of them are quite subtle, some of them we’ll try and just describe, but we’re actually going to go through some of the ones that we’re watching each and banter back and forth, talk about it and figure out whether they are going to be epic, partly epic, or whether they will be a solved problem soon.
AB 02:09
No, we’re not going to forecast and say we want this by next Christmas or things like that. Who was the person, was it Bill Gates or someone else who said that I only need one computer per continent?
We aren’t going to be going that far, we aren’t going to forecast the future. We’re just going to tell you what we’re watching heavily. I might start if that’s all right. One of the ones that is really on my radar that was obviously one of the ones that made this spatial podcast and now video quite critical is to define the field of spatial AI.
We are purposefully casting a net reasonably wide, but I would just want to point out that we’ve got, well, I might, well, hey, I will share screen, but for those of you not watching on at home, this is on spatial .space and this is a little playground that we built that really does show a couple of things that we’re watching out with this with this team.
AB 03:06
For those of you listening along intently, that was my turtle Hubert doing a dive off his platform. Great.
So spatial.space is a 3D graph map of some of the fields that we’re thinking are connected to spatial AI.
We kind of know that spatial AI is the mashup of spatial computing, geospatial data analytics, things with depth, but then the AI side is, I must say, probably one of the things that has really taken off quite large.
This map is actually editable by anyone. It’s linked to a JSON file on GitHub. Anyone can log in, add nodes, take away nodes, do some more cross -linking. It’s kind of funky. It’s a lovely thing. Let you play and let you have some excellent fun.
What it does show is that there’s so many facets to this field that I think it is an amorphous graph. I won’t say blob, but it’s something that I must say we kind of have to keep on coming back to some of the topics that we’re covering and some of the things that I know I’m watching.
Some of them are actually quite central to this and others are on the periphery. I think we’re definitely leaning quite heavily into the AI world, and I think the geospatial side is one that I think now is really catching up quite quickly.
I’d like to get your thoughts team on some of the fields that have really risen to the top and some of the fields that probably on this list, on this graph, are things that, yeah, that is a thing that is really going to be taking off in the next 12 months. There’s some real standouts for you.
Violet 04:40
I think a lot of these are going to adapt, so things like user experience and data science, it’s kind of like everything right now is trying to, things that weren’t spatial like user experience are trying to grapple with having that as something they can more easily access so that there’s like a lot of adaptation happening there.
And then things like data science, you know, maybe is pulling more spatial things, but then things that are already spatial like geospatial data and that whole realm of GIS, I think now they’re grappling with AI emerging, so it somehow feels like a lot of these fields, it’s not necessarily that they’re individually taking off, it’s that they’re learning, all these fields are coming together a bit more because of getting stronger.
AB
Makes perfect sense. I think a very first version of this I drew as a Venn diagram and it was overly simplistic. It really was two fields smashing together and the overlap was quite massive and there were more things in the overlap in the union than there were in the extremities.
This is another view and it is definitely purposefully complex and nuanced, but by all means it’s got some of the things in it that I think we’re definitely leaning ourselves towards the AI -ML technology just because the plethora of news that’s coming out.
I think you’re right, Violet, that the geospatial world is there and is sort of leaching some of those techniques slowly, but it’s definitely not racing towards the middle as far as the AI world is almost overflowing into spatially aware 3D models, those kinds of things.
Violet 06:41
Yeah, I think I just saw a video last week from these folks at, I want to say at Carto.
Um, and they were actually posting a walkthrough of some, um, I think probably open AI enabled workflows, but just how much of the manual kind of plotting spatial data and whatnot was automated, like that kind of cleaning the data and plotting it, um, was all done now through natural language.
Absolutely. Commands and stuff.
AB 07:21
So look, my mega trend summary then I would say is that spatial AI has AI pretty much bleeding into the geospatial and the geoscience world at a great rate of knots, but probably the geospatial and geosciences world is not embracing it as fast as the tools are being thrown at them.
Probably it’s that speed of iteration of some of the techniques that we’re seeing being thrown in from the AI ML world are literally too fast to productize, to go from toy to playpen to robust to embedded red button press that does everything for you.
So hopefully my first trend is that that will actually come, we’ll see the left hand side of the Venn diagram run faster towards the middle and that’s something that’s pretty much going to be on my radar quite a lot of any tools and techniques that can actually harness some of the cutting edge being thrown over the fence from the ML world.
Next up on our list we’d like to go next with the trend that they’re watching.
William 08:28
I think overall, the trends that I’m looking out for are the ones that are the foundational, I would say, design patterns that are emerging around AI models. And right now, it looks like there are three leading contenders.
One’s the large multimodal model. And OpenAI released a couple of days ago. Their GPT -4 Turbo model, which combines vision, function calling, and structured outputs, which I think is super fascinating.
Because now you can, I showed it to the students in the class, and now they’re hopefully experimenting with it now in the wee hours, where you can upload or reference an image and then trigger functions based off of the content of those images.
And so part of the exercise that they’re going through now is, given multiple inputs, say you’re observing a space, can you create a kind of pseudo system that is aware of the activities in that space and then triggers various behaviors from that?
So that model is really interesting. I’m sure that Anthropic and Google have equivalents of it. I’m more faciled with the OpenAI models themselves, although I’m queuing up the Claude Suite opus and whatnot to go experiment with here in a few days.
William 10:06
Another pattern is this agentic future, which I’m quite a fan of, where you have individual agents that are specialized in their use case that can negotiate with each other around a task. And there have been a lot of interesting demonstrations of the potential power of that, like virtual programming workshops, virtual game studios, things like that that are really fascinating.
It kind of has the kind of hints of microservices architecture back in the cloud days when that started to become the trend and the sort of way to break apart the monolith. And it allows you to sort of swap out implementations without having to take the whole system down.
AB
their presentation of how they manage their Netflix empire early days where they had hundreds of microservices and servers responding to every possible facet of a user’s call. So you think we’re returning to that potential mode of bespoke specialty services that are now AI agents able to assist with each A1 trick pony.
William
Well, I’m not sure that it’s a return. I would call it more of like that kind of pattern is an established pattern. And we’re seeing how that pattern applies in the artificial intelligence realm. I think it only makes sense because that’s a much easier way for open source developers and sort of small companies and even medium sized companies to engage these technologies.
It’s like if I can, it’s that old question of, is two better than one? Are two brains better than one? I think if like even if you have these tiny models that are responsible for just individual tasks and they’re being tuned for them, maybe there are advantages to that.
Cost wise, from an organizational perspective, different teams can own different capabilities independently of others and chip faster. Maybe there are advantages to emergent behaviors. If you put enough agents together, maybe there are emergent properties that can result.
And some of these models that can run locally are really fun to tinker with. And they don’t take much memory if you pick the right ones. And so maybe there’s frameworks like LangChain and MetaGPT and AutoGPT, those are sort of the first harnesses that we can use to experiment with it.
William 12:50
So I like that very much because it allows the technology to be accessible to folks that don’t necessarily have a PhD in machine learning. And then finally, there’s this emerging panel called a mixture of experts that’s emerging as well, that I understand the least.
It has more to do with the architecture of the model and both not only how it’s deployed, but how it’s trained. So that’s one that I’m just learning about. Mixed role, sort of the first one that hit the market, that got everyone’s attention, that sort of proved that that pattern was viable.
And it has trade -offs. Like the agentic model is nice because you can run individual models on different machines or different VMs or different containers and sort of distribute the memory footprint.
Mixture of experts requires a lot of video RAM. You have to load all of the bottles entirely in RAM either for the training or inference or both. And so that’s the latest one that I think is interesting to watch.
So I’m interested in all of these from an empowerment perspective as well as a capability perspective.
AB 14:08
William, in that mixture of experts, does that mean that they are all sharing some knowledge and sharing some of their data sets across the one bottle? Is that mixture of experts, obviously, then as different the ensemble mode, I think, was what it was called five or so years ago, where you would have separate VM, separate models, or bidding, or putting in their confidence votes of what data was coming through?
Is this a back on single metal? Is this a way to do some more nuanced interplay between competing models?
William 14:48
I think that from what I understand, there are multiple models deployed, and they share a foundation of parameters. And then what is tuned on top of them are each expert, so to speak. And that’s a separate set of parameters on top of it.
And so from that perspective, I think it has more to do with performance and specialization of these experts, and thereby the entire mixture being better overall at various tasks that it’s being tuned for.
That’s my current understanding, which actually isn’t very developed yet. But they do sort of share, from what I understand, the set of parameters that then the training process will rely on, and then the experts being sort of tuned on top of that.
But as far as its sort of deployment, and how it learns, and so forth, I’m certainly not an expert by any stretch exactly on how these work yet.
AB 15:58
There’s three patterns there that form the same trend of large language models are becoming increasingly specialised and different architectures actually might be competing for which one is more relevant to certain tasks.
William
Yeah, I think so, and I think each one of these, I think, has a different set of players that can, again, that can deploy it and use it. So developers who are very good at complex architectures and maybe web architectures, like web developers in particular, and mobile developers to some degree, are also very good at this sort of understanding that there’s more than one player involved in their architecture.
There’s client server architecture, there’s, you know, being able to, having to access multiple services, there’s a set of authentication services on one side and a set of backend services on the other, and coordinating amongst all of those is, I think, can be generalized into building AI powered systems using those skills.
And so you don’t necessarily have to retrain your entire developer staff in this AI world to understand how to create models from scratch. But maybe others do have that talent and will actually invest in the effort of training models from scratch and making their own multimodal models and whatnot that combine static imagery and video vision with the language model paradigm and glue that links all of that together.
But like any savvy developer today can get started with an agentic architecture now and run with it. And I think that’s super exciting.
AB 17:48
Mirek, trends to the old, looking out for, obviously.
Mirek
Yeah, I’m trying to figure out what to talk in this broad spectrum of things. It’s not easy I actually agree with William that that’s a strong trend of you know, we’ll see more and more expert systems here and there popping out and You might think of 2000s where every business needed a website immediately, you know something that they weren’t used to around 2010 ish everybody needed to be on social networks to talk to their customers and now you’ll have a your own AI agent that talks to your customers and partners or whomever about your business or product or code or Whatever whatever is is important to you and I think these systems will be able to to communicate pretty pretty pretty quickly also and you want you might form them, you know this consortium of experts and I think that’s really interesting.
I’m trying to sort of wrap my head around how all this translates to robotics for obvious reasons, that’s that’s that’s that’s where I work and I’m still not sure how you know I mean it it’s kind of obvious where this is headed and what the difference is like you might you might point back to computer vision and when you know it Took so much effort to to algorithmically recognize anything from grid of pixels and Compare that to how we do this today and how we change these systems and you know You have these zero -shot networks now that don’t even require any training for specific classes of objects so you see how all that is going away and and how Sort of conceiving these systems come down comes down to knowing what a certain Model can do for you and then training it with with the right data I think we’ll see huge improvements in everything robotics very quickly Because the problems there and we’ve been seeing it over the past few months all of a sudden You see the same hardware being utilized in a new way all of a sudden There’s this dexterity that we didn’t see before turns out.
It’s the software that you know is driving everything or a human at the other end, but As we use this human input to train these systems Performing the same tasks with with the same body model effectively it produces a striking results as These vision models compared to you know hard -coded Hard -coded software implementation or human human written program So it’s fascinating and it makes you think what?
Mirek 20:49
What’s the role of human engineers in all this right then? Effectively I think we still need to push down prices of hardware and figure out how to compute all these new things that we still can’t compute and how to power it and Somebody needs to put all these things together But as we just mentioned we might expect a lot of expert systems being able to negotiate.
You know these complex things So where’s where’s the role of an engineer and all that and I think that’s quite interesting to ponder
AB 21:27
So the world of hardware is not catching up to the speed of software updates, software advances? I wouldn’t… In perfect sense, it can’t iterate at the same virtual zero -one -zeros.
Mirek
I would say the software was lagging and still is lagging behind hardware and what we can do with hardware, but it is so difficult to program these things. We touched on humanoid robots many times. I still believe the economy will dictate other body types more optimized for the task at hand, but the intelligence might just come as a model that is just out there open sourced and you just slightly customize it and here we go.
The biggest task then is maybe integrating all these parts, coming up with a design that is suitable for the job at hand. All these expert systems will probably help you with that a great deal, so it’s like the machine helping us design itself in the process, which is quite interesting, but it’s one way of looking at it.
I don’t think it’s going away. I think we’re just getting started.
AB
Very nice. Violet, some trends that you’re watching. We are, you know, a third of the way through 2024. Don’t need to forecast what’s happening by the end of the year, but the things that are on your radar that when they pop up, you are just reading, ingesting.
What’s your top picks?
Violet 23:05
Yeah, well, definitely something that I know a lot of folks here care about is anything that’s spatial and anything that’s really like comes from the physical world. And having come from the realm of teaching like physical computing and working with sensors and actuators and stuff like that.
I think it’s really interesting to see how this whole realm applies to things that aren’t language, but are able to use language. So just this week, this company called Architect AI is this company that I had known some of the folks at Google back when they were part of ATAP, which is like this really great, I can’t remember, it’s like advanced technologies, something at Google, and everyone there was really focused around really innovative products usually having to do with material and physical world.
So like Project Soli, which was wearable, and they worked on things like the radio sensors for the Android, so that it detects your presence as you walk up. So that whole bunch of folks from that group ended up going and starting this company.
And I’m just going to share my screen because I think it gives a sense of what I’m talking about, give a little bit more visual context to this trend. So here it is, Architect AI. And so what they’ve been doing is working on ways of taking sensor data, something that they do work with a lot, and just translating that to things like natural language.
So it seems like they have this use case, I think they’re working with Amazon now around tracking things like how a package is being handled, you know, so it’s saying the package is being shaken versus it’s being handled gently.
But they’re looking at all kinds of different sensors, they’ve done a lot with video detection, and so things like, you know, dash cams. So everything they’re doing is like, how do we take sensors and things that are abstract data and tie that with language as well, so that we can detect what’s happening in the world in human language.
Violet 25:54
So I think it’s just going to be interesting to watch how things in the physical world, particularly things like sensors, I play with if this, then that a lot. And so thinking about things like Williams talking about where we have agents that can go and execute things in the physical environment, but also being able to detect and understand those things with natural language, being able to control those things with natural language.
I think that’s going to be really interesting. And I hope we see a lot more things that allow us to move out of the kind of canonical computer, mouse and keyboard world and back into the real world.
AB 26:43
Back away from our desks, so not even standing desks. I reposted on LinkedIn a beautiful video showing, I think about a 20 year evolution of the desk and then all the applications falling into the computer as notepad and calendar and things.
And then more things rolling into that computer as the computer gets better and better and then tablet and then phone. And the last frame was a pair of sunglasses, aviator sunglasses as perhaps the whole thing can be rolled into just what all four of us have on our faces right now.
Probably symptomatic of spending too many. I hope not, I hope not. No, okay. As in even less interface than that or it doesn’t require batteries or inside out versus outside in.
Violet
Well, I’m just saying I’m a very avid non -supporter of glasses, VR glasses, and mixed reality glasses. And so any opportunity I have to say that I’m against it, I will say it.
AB
Excellent. Stand is noted. That’s a great hill to die on, and by the way, it’s the hardware world in that space is ramping up. It’s almost a mega -train, but it’s not exactly, it’s not following Moore’s law exactly.
Violet 27:55
Actually, I can say another trend related to this, because I think it’s important. I think another really important trend to watch right now is what’s happening with the, what do they call it? The no phone.
William spot me here. What is it called? The low sensory phones?
AB
As in feature phones going back a level to less interface?
William
The dumb phone movement!
Violet 28:28
Yes, yes, that’s what I wanted to say. So the dumb phone movement, that I think is something really to watch. And I think it relates a lot to what’s been happening in AI and spatial AI, something that we’re definitely watching, which is, I think we have this one trend, which is the Apple Vision Pro, it’s like very high sensory kind of, we can do everything, so we’re going to do everything all at once.
And then there’s this dumb phone trend where folks are really exhausted from having so much interaction and stimulation. And so things like the humane pin, and some of these wearables are really trying to combat the like, do everything everywhere all at once, rather, we’re going to like, take just the tiny piece of information you need and put it in context, because we know where you are spatially, and we know what you need in the moment.
So I think that’d be really interesting to watch that kind of like, low sensory, just the thing people need and try and almost like dial back how much attention someone needs to get things, which is, I think probably like a non visual medium, maybe it’s not glasses, maybe it’s like audio.
AB 29:44
very good. It also harkens to your previous trend of using sensors for not different purposes but from the one sensor how much can we do with it not to overwhelm but to learn more without having to have millions of sensors overwhelming things.
So yeah the the less is more and I definitely hear you of we are definitely boldly going into a high -end, gee that old Microsoft versus Apple ad campaign spoof parody of it’s not the I’m a Mac I’m a PC but the tech bullet points exploding off the screen of buy this because it has x or y versus and not this is back versus apple but just into your point of this does something for you and that’s why you should buy it as opposed to it’s got 61 gigawatts of yada yada and you know mine’s faster than yours yeah usable as opposed to feature driven.
To that topic I’ll share screen – another thing I’d love to show you is and get everyone’s thoughts on this – is another playground on spatial.space.
This is a history or a timeline of AI ML models taken from the awesome LifeArchitect so he’s the author of the data I’m just putting it here, but the trend that I’d love your thoughts on is the widening gulf between open source models and commercial models and really those that are yes associated with a credit card those that are released but not available for consumption and then that massive dial down of the open source.
I’m quoting the three amigos a lot – the ‘many plethora’ of just you know the masses of smaller models that are good/great that you can use at home but the gap between you the leaderboard and the ones you can run on a personal computer or you know use for shekels-per-hour on Hugging Face is just massive.
Claude is the biggest one. Yyou know Open AI is living here – turbo is not on this list yet I guess hasn’t sort of been fully announced – and of course Open AI 5 is (I’m getting my numbers mixed up!) the next Open AI iteration is being rumored but this many many models and if you in fact zoom in these sort of places there are so many things in these bottom sections I’m not going to say these are the penny dreadful stocks – they’re not – they are just models that are trying to do more with less and there’s no shortage of them.
There’s dozens down here in the in the small parameter bucket. There is a mighty fine line here at the 540 billion parameters that must be a logical place to stop but that gap we have commercial models just raging ahead and they are starting to form their own ski jump and then everything else is hovering around the it’s good it’s useful but it’ll probably not get used or it’ll be eclipsed by something else that’s coming later thoughts on emergence of all that widening gap…?
Violet 32:55
Let’s all just raise a trillion dollars and do an open source. I don’t know how we’re going to make money, but…
Mirek
I would say that this comes down to how much it costs to train these models. So the way I understand it, these LLMs are trained in multiple stages, and in the first stage you train it to learn, to understand the language that you’re then going to continue using.
And that might be some of the base models you have there as open source, because I would think that some models then take that, or the company publishes this bit as open source, but then continues in training and feeding the model, specialized data, and training it in a certain way to make it behave and act in a certain way.
And I believe it comes down to an open source community not being able to spend as much as open AI, because it’s ultimately about the cost of compute these days, and until we figure out how to train these models in a more efficient way, it’s going to be like this.
Violet
Do you think there’s ever the potential that we see some major government type project that would, almost like PBS -like, sponsor a larger model that’s open?
AB
Gotcha. The Canadian national model, but a nation backed as opposed to, yeah, there have been a few efforts by open source to group together to do things. I think they’re probably down here in the middle of the mix.
They’re not exactly anywhere near the, near the top, though…
Mirek 34:44
I think we might see a Wikipedia model, you know, coming straight from the Wikipedia Foundation. And that might be open source and just funded with with contributions such as the Wikipedia is. I think we’ll see all sorts of different expert systems, and this might be one of them, but I think it’s you know, the cost of really acquiring the data and then running the training is so vast for your everyday hacker or, you know, enthusiast.
I mean, maybe what we just saw the Nvidia announcement might change that, right? Maybe, you know, these days you can get, you can host your website in AWS for 20 bucks a month. And, you know, there were days where this wasn’t possible.
You had to have a computer physically connected to the internet, paid for the connection, made sure that the server is running and all that. And if you wanted more traffic, you need more, more hardware, more metal.
And this changed. So I think we’ll see that trend coming also. And with these cloud services trying to be as efficiently utilized as possible, it might drive the cost down. Also, I think that’s the biggest driver for the disparity.
AB 36:08
Yeah, makes sense. I know that with every new advancement in the ML world, we are all all of us looking to see whether there’s a code release or a press release along with that and I think the ratio is kind of not approaching 50 -50.
Some of the biggest ones of course are press release and death by press release and we’re seeing those press releases being moved so far forward say hey we’re going to announce something that we may or may not release later in the year but I think that probably leads itself to the fact that the later releases are almost never open source.
We get a lot of things that are, you know, the large usable models are definitely the trend of these are the ones that you want to throw down the credit card and play with to assess and then with APIs work into your current project.
I love the Hugging Face, the teacher-student, the decimated model, the yes can we learn from a large model and make it one-tenth the size kind of approach. That definitely helps in bringing it back to us mere mortals but I’m thinking that the rise of the large models, the incredibly well -funded, commercially backed worldwide releases are going to be the driving force until we ever see a plateau of features and then we’re going to see this noise of models being released that are open source or micro payments.
AB 37:29
They’re going to be useful/handy but my pattern or my trend that I’ll be watching is when do we start to use these lower models get just as good as the big ones and that equitability is really not happening just yet.
We’re seeing the large ones are still taking the share of the limelight.
William 37:51
Well, that’s sort of the way the tech rolls these days. I guess I wonder if these larger models are so not only because of the nature of the technology itself, like you need more parameters for more and more sophisticated behaviors, but also those models are intended for large markets.
They’re generalist models. But also, there’s the kind of hype cycle that’s fueling all of this as well, because the tech industry is really trying to figure out, can we do it? How far can we push it?
How far can we push the hardware, the size of the models? Like, what is the potential of the technology at all? Which is somehow characterized how the tech industry has gone to market, so to speak, in a lot of ways.
When you sit in a product role, you tend to think the other way. You tend to think, OK, where is there a pain that I’m going to address in this market? And can I exchange that pain for my product plus a price that then I can take back to my stakeholders?
William 39:16
And I wonder, because no one has raised their hand to say, I need 3 trillion parameters by next year. So it’s not a market -driven thing in terms of there being a single direct customer, so as a kind of straw person argument.
And so I do wonder if that trend is more about the sort of can we do it, the kind of space race -like nature of it, which is eerily similar to some of the hype we saw around phones after the iPhone came out, and also just the tech boom in the late 90s.
AB
the PC race of the 90’s?
William
Yeah, totally. Although, I mean, the anecdotes that you hear about those days are like people throwing around money, not even knowing what they were doing to begin with. But I might argue that there was something similar in the Web, sort of cryptocurrency Web 3 boom, like the way that Ethereum was incubated.
It was one of the founders, Joe Lubin, created interested in the mission of Web 3 and cryptocurrencies could go there and just create until projects really addressed a need. And so thereby born Infura and Metamask and some other really well -known Web 3 products.
So I think that’s what we’re seeing here. Even the sort of use cases that you see are the obvious ones. Like, oh, we’re going to create a chatbot for like customer service purposes. And I think every company is sort of grappling with what that looks like.
William 41:11
And it sort of makes sense because they’re designed for sort of generalist conversations. However, like I wonder if really what we’ll see is are these other folks that are, if they have models to work with, they’ll start really experimenting with actual on the ground use cases.
Okay. Well, I don’t need a generalist model that can have, that can like recite all of human knowledge articulately in a way that I will fool professors into thinking that I’m an intelligent PhD student, that kind of cheering test -esque type task.
But what if you need something that just triggers off of sentiment, right? And I’m a brand and I’m really interested in the sentiment around my brand. And so there’s services like this that exist already, but maybe AI will empower another set of startups, for example, that are sort of watching a space, the Twitter sphere or the blue sky sphere or whatever.
AB
on the decline, yeah, where is the place to get sentiment? Is it more company -focused than, as you say, general -focused?
William
Totally. Maybe there’s a better sentiment analysis future. I just picked that out of the air. I have no idea.
AB
If I can go around in conclusion: do we think there’s a megatrend from Violet, the product, the software, the dumb phone; from Mirek, the going from large tools to everything in your world; William talking about models, that is really — we may be beginning to watch a fold back, a rollback, anyway, a mixture of the large technological curve is almost certainly going to be there, it’s going to keep on pushing things forward, but there’s going to be a second movement of things that are relevant, product focused, just in time, just for me, and not every bell and whistle and everything does everything, so not a personal movement, but a more relevant movement.
Is that something that in all of our fields is something that might be on the cusp of emerging now? Seeing nods, seeing thinking, seeing yeah, yeah. I just think it’s
Mirek 43:31
it’s all these things and William’s point was actually very spot on. I think these companies are chasing, it’s a flagship product, right? ChatGPT is a flagship product, so it needs to know everything, be able to talk about everything and to everyone.
But it also makes sense, I guess, because this emergent behavior that seems to us like intelligence, it comes from that much data. And if you don’t feed it as much data, you might be expert in something, but it’s gonna be quite stupid in any other way.
Like there was this case where I think it was BMW somewhere, put this chatbot on their website and somebody convinced it to sell it a new BMW for like two dollars or something like that, that happened.
And you don’t want that, so you need like certain level of, you know, like at least the human level of intelligence, you don’t need to be like all knowing cyber guard, right? To be a chatbot on a website.
But I think you’re right that it doesn’t have to be as gigantic models to be practical. And we’ll see those specialized systems that maybe don’t require that much energy and compute and data to be useful.
AB 44:54
I’m reminded of a famous quote. I don’t know who the author is. Someone will be able to tell it to me. But if we’re talking about a general model, what’s the quote is, when you look around at the average intelligence of the average person and realize by definition that 50% of the people are not as smart as that, that’s a bad thing.
I imagine that loosely applies to general models as well. The fact that they can cater for a general market with comprehension, understanding, read the entire internet, great. But I think we’ll be seeing the emergence of other models that are gonna be company -specific, domain -specific, personal -specific.
I know in the kind of fields I play with, there’s a lot of people who don’t want a general model that’s on the internet. They want one that has read and ingested their own datasets 100% and is industry -weighted completely.
So that is one. I think that’s gonna be the rollback of how do we turn the advances we can see, capture that, bring them down to worth and run them on a machine that’s not gonna be costing a million dollars a month.
AB 45:59
Alrighty team, thank you so much for that. That is a fairly meaty list, but that’s perfectly fine. We’ll make a bit of a blog post and I’ll try and index these into some logical fashion. I know there’s more are going to emerge as the year rolls on, but really thanks for your time to figure out what are the things that are on our radar right now.
I think there is trends and there’s mega trends that even with the four of us, we’re starting to watch out for. So I know that my Google alerts is set for many things. My archive .org search is set for many things too.
There’s more bots out there that are trying to feed me with knowledge that I am still being overwhelmed. I now need a meta bot to sort out which of the things are actually top of my list. It is often quite tiring to keep up to date and stay somewhere close to a leading edge, just to use and to figure out what is real and what’s not.
Hopefully this forum will try and at least put together some things that are relevant for this niche topic that’s emerging. But by all means, we are not oracles. We are simply just saying what’s caught our eye.
So hopefully, by all means, all links are in the show notes. Have a read and we’ll be able to follow up in the coming weeks. Well, from all of us here, thanks so much for listening along and for watching along.
We can actually wave now knowing that video works. So from all of us here at Spatial, we’ll catch you on the next episode. Bye bye.
HOSTS
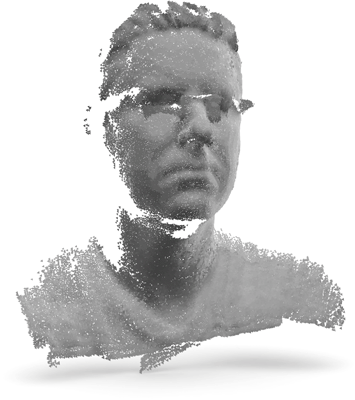
AB – Andrew Ballard
Spatial AI Specialist at Leidos.
Robotics & AI defence research.
Creator of SPAITIAL
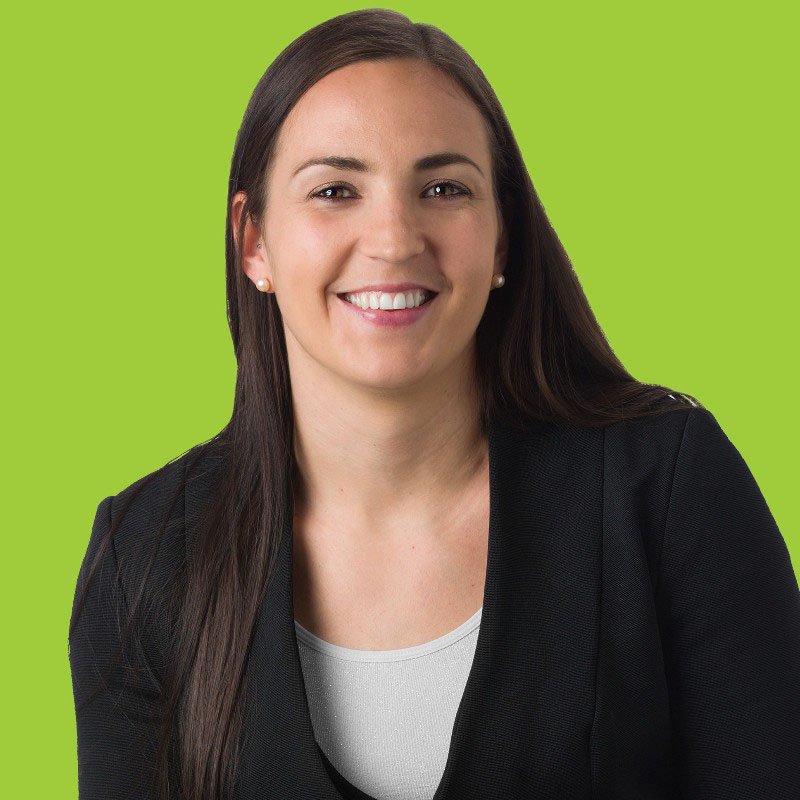
Helena Merschdorf
Geospatial Marketing/Branding at Tales Consulting.
Undertaking her PhD in Geoinformatics & GIScience.
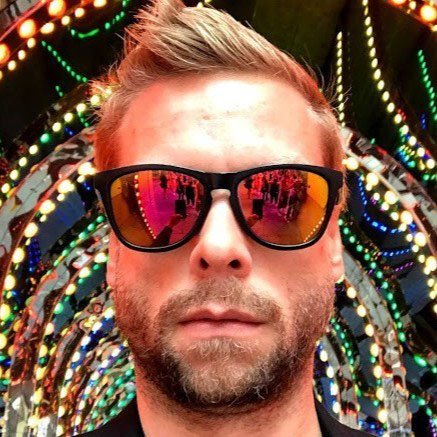
Mirek Burkon
CEO at Phantom Cybernetics.
Creator of Augmented Robotality AR-OS.
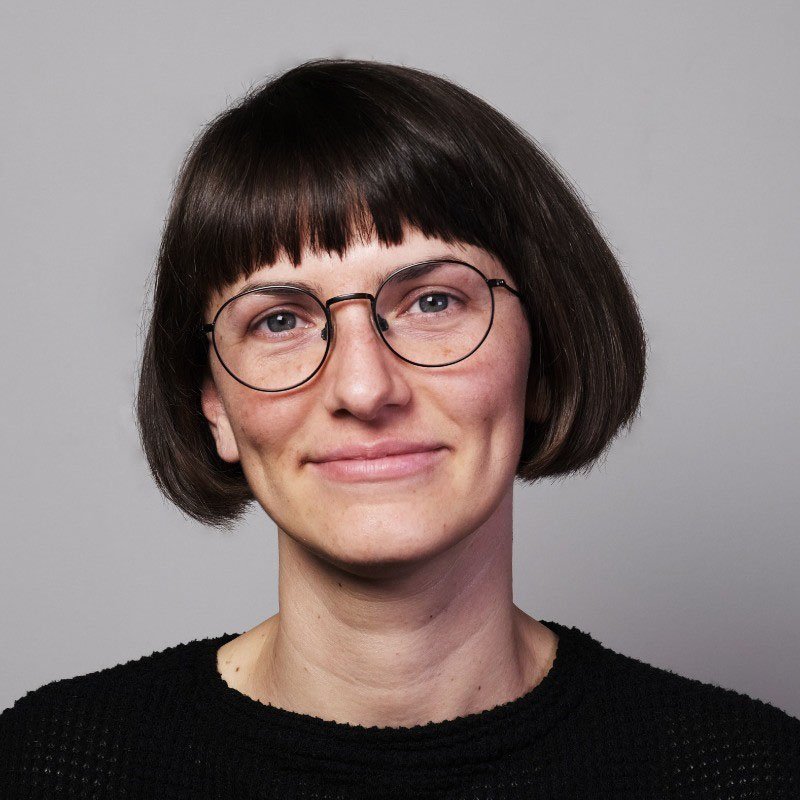
Violet Whitney
Adj. Prof. at U.Mich
Spatial AI insights on Medium.
Co-founder of Spatial Pixel.
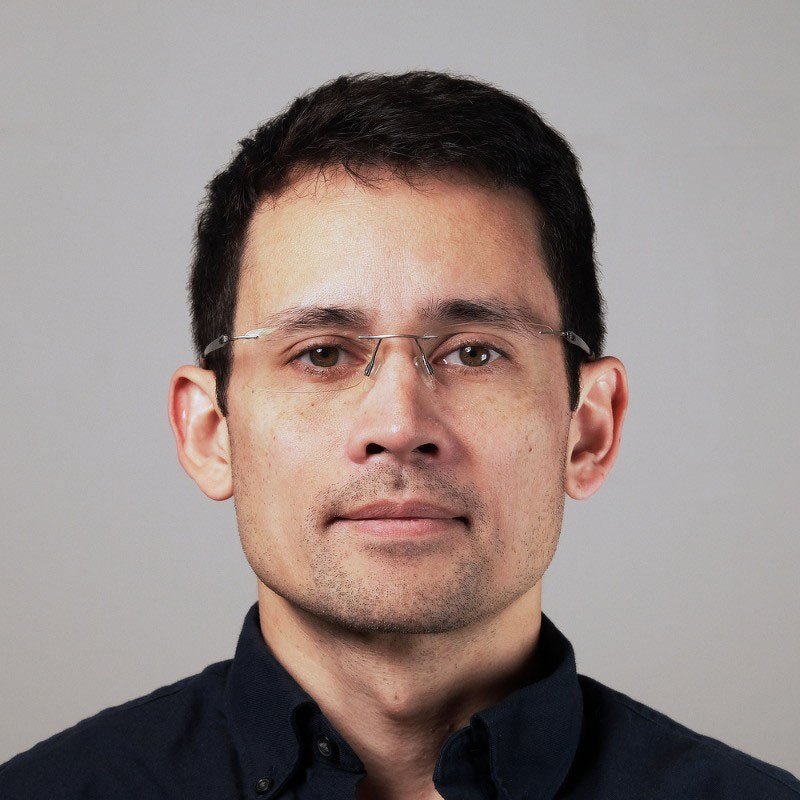
William Martin
Director of AI at Consensys
Adj. Prof. at Columbia.
Co-founder of Spatial Pixel.
To absent friends.