This week, AB talks with Phil Delaney, CEO of MapAI. This new company aims to provide a conversational interface for querying spatial data using large language models fine-tuned for spatial analysis. Phil discusses the motivation behind MapAI, its current state of development, and plans for future deployment and integration with existing platforms. He also shares insights from other projects he has been involved with at Frontier SI, a social enterprise focused on spatial technologies, including Farm Map 4D for monitoring land cover and Value Australia for automated property valuation.
We also touch upon the Australian Satellite-Based Augmentation Service (SBAS) called SouthPan, which provides precise satellite positioning corrections, and its potential $6 billion in benefits across various sectors in Australia.
https://www.linkedin.com/in/phillipdelaney
We’re also publishing this episode on YouTube, if you’d like to watch along in full living colour: https://www.youtube.com/watch?v=2lMGd1Bygec
Chapters
06:42 – MapAI: Motivation and Development
Phil discusses the motivation behind MapAI, which arose from a collaboration with the University of New South Wales to explore the spatial capabilities of large language models like ChatGPT. The goal was to create a fine-tuning layer that could translate natural language questions into spatial queries and provide appropriate responses, including maps and visualizations. MapAI aims to integrate with existing platforms and data sources, acting as a conversational interface for spatial data analysis. Phil shares details about the current state of development, including early proof-of-concept deployments with a major data company and local councils.
25:56 – Frontier SI and Other Projects
Phil provides insights into other projects he has been involved with at Frontier SI, including Farm Map 4D, which used satellite data to monitor land cover and assist land managers in sustainable practices, and Value Australia, an automated property valuation model that incorporated a wide range of spatial data and allowed users to simulate the impact of infrastructure changes on property values.
36:39 – Australian Satellite-Based Augmentation Service (SBAS)
Phil discusses the Australian SBAS called SouthPan, which broadcasts correction signals from space to improve the accuracy of satellite positioning systems like GPS. He explains the motivation behind SouthPan, its potential benefits across various sectors, and the economic analysis that led to its operational funding. Phil also touches upon the GNAN program, which distributes positioning corrections over mobile networks, achieving even higher accuracy on compatible devices.
Transcript and Links
AB
Well, g’day and welcome to SPAITIAL. This is Episode 20, and we are joined by a special guest this week. I have Phil Delaney with me. Phil is – goodness me – wearing many hats. We’ll find out exactly which hats he’s wearing today.
I was intrigued by Phil’s LinkedIn post a couple of weeks ago. He is CEO of the newly formed organization called Map AI. And yes, dear listeners and watchers, you can tell that I was intrigued instantly.
AB
Phil, welcome. Great to have you with us. Look, your history is wild and varied. And I say ‘hats’ is probably not even the correct terminology for it. Is there a plethora of many hats phrase we can possibly put on you?
But by all means, can you introduce yourself a little bit? Tell us a bit about some of the hats you wear right now. We’ll get up to MapAI soon.
Phil
I wear two main hats at the moment. One is as Deputy CEO and Chief Growth Officer of Frontier SI. Frontier SI is a social enterprise that’s been around for about 20 years. And we really sit between research, government, and industry to help bring interesting ideas through the real-world products and services in the area of space and spatial technologies.
And then through that, one of our recent innovations with our partners at University of New South Wales has been to combine generative AI and spatial analysis. And so through that, we’ve created a subsidiary company called MapAI, and I’m the CEO of that as well.
So I spend, at the moment, about half of my time with each of those two roles.
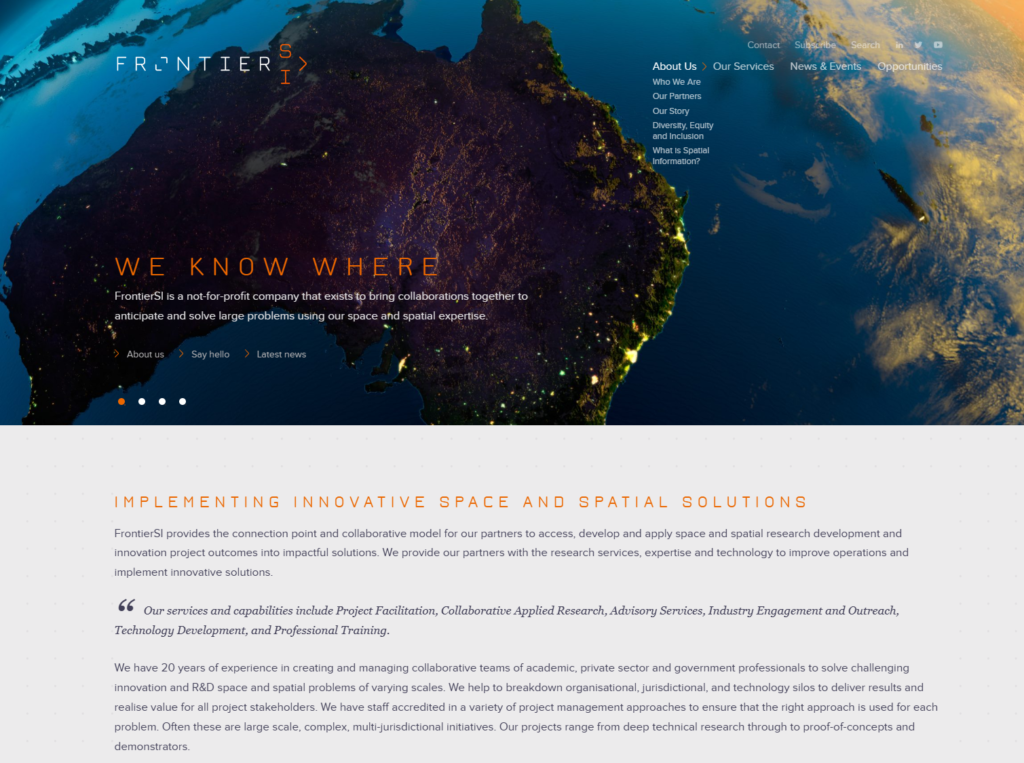
AB
Wow. So, we’ll tell you what, we’ll leave MapAI for a few seconds because that’s going to be a large tangent. Frontier SI. So, 20 years of a social cooperative. Can you explain what the brief has been there, some of the projects you’ve been involved with?
Phil
So, we were formed in about 2003 and originally funded under the Federal Government’s Cooperative Research Centre program. So that’s a program that’s been around for a really long time that looks at funding collaborative innovation in a whole lot of different industry sectors, everything from hearing to pork production to mental health.
And so we were funded as a centre to focus on spatial information. We had two rounds of funding, one that went for seven years, one for eight years. So that covered our first kind of 15 years of operation.
So we were funded through a government program, but that covered some of our funding. More than half of it came from contributions from industry, university, and particularly government agencies. We were a CRC for about as long as you can be under legislation.
And so at the end of that, the thing that our partners valued the most, even more than a lot of the solutions that we’d created, was the model of collaborative problem solving that we made available.
So we’re now coming up six years at the end of June, which will be six years since leaving that program, kind of standing on our own. I’m still looking at a similar mode of operation, but we do a lot more of the direct problem solving ourselves with some internal technical capability and specialists, particularly in the area of precise satellite positioning and in satellite image analysis and other image analysis.
But we still very much worked through that collaborative approach where almost all of our projects have some combination of government industry and academia working together to solve major problems that use space and spatial technology.
AB
And look, I need to mention, you’re also a local lad. We’re both on this side of the Southern Hemisphere, here in sunny Australia, granted it’s sunny as of this day, but it’s cooling down. That’ll give us some ‘place’ when we’re talking federal government – we’re talking about the Australian Federal Government.
I’d love to come back to that concept if we had time of the global positioning satellite in Australia. The acronym is definitely new for me, so it has escaped me. But I know that that has a lot of headway also around this part of the world.
Frontier SI had yet 15 years of fully funded CRC, and then the last many years it’s evolved into perhaps more traditional commercial kind of mode?.
Phil
Yeah, I mean, so we’re a not-for-profit organisation, but we like to describe ourselves as a social enterprise. So that really means that we use very commercially grounded strategies in order to make a substantial difference in social, environmental and economic outcomes.
So as a not-for-profit organisation, we don’t have shareholders. We do run a lot of projects that cover a really wide variety of work from independent advice that can happen anywhere in the world through to that technology development kind of projects and any surpluses that we make along the way through the projects that we run, then get reinvested into creating new technology like MapAI.
AB 05:57
Lovely segue. I’d like to thank the Honorable Minister for his question. That’s a sitting duck for us.
MapAI, so the big announcement was three weeks ago. Can you tell us about how MapAI was formed? By all means, we have a teaser of what it is, a website, which we’ll link in the show notes.
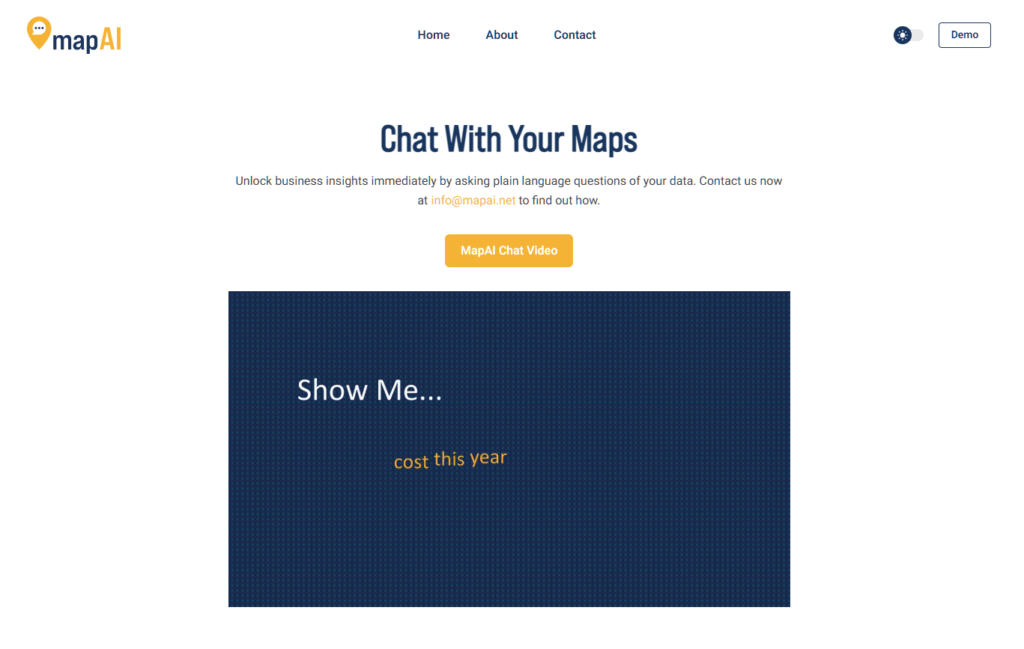
AB
If need be, we can do some screen sharing and I can talk to it for those listening in with headphones. But can you tell us about MapAI, its purpose, its future and where it sits right now?
Phil
Yeah, absolutely. So, like I said, we’ve had a long history working with research teams to try and take ideas through to real world products and services. One of the universities that we have a long relationship with the University of New South Wales.
And last year as part of just the regular discussions that we have around finding ideas that mentioned that they were kind of noodling around with chat GPT and trying to investigate its spatial capabilities and had seen certainly some good ability to translate someone’s question into code that we could execute.
But a lot of the understanding of the nuances of spatial information and why it maybe requires a little bit more special consideration than some other types of data meant that we saw a good opportunity to create some fine tuning on top of what at that time just ChatGPT was doing in that conversational AI large language model type work to see whether or not we could get a working prototype that would answer questions in a bit of a different way.
AB
The problem normally within the spatial world is that people don’t have the vocabulary to know what they can ask. So was this the layer that lets people ask deep, dark questions and have sensible prompting?
Phil
That’s exactly right. I mean, I think that what we were really trying to do then once we made that first connection that let people interrogate a single data set was to say, OK, great. One of the biggest things, as you say, that inhibits people better using the insights that they can get out of location information is that they don’t know what questions to ask.
They don’t know what data might be required. They don’t know how to translate between the raw question that they just need answered and what that might mean from a process and data perspective. Yeah.
And we wanted to try, and absolutely not for every application, but for a lot of simpler data sets, well -structured, well -understood geospatial data where people have regular questions. We wanted to be able to create a chat -based interface where someone could just type a question, and then the work would happen in the back end to create a query automatically that could be executed and would either respond to that user with a text response, some kind of table showing the information, or, of course, a map.
AB
Brilliant. That is, I think, where the future is heading. I know we had a chat last episode where we said the tools for the future will be less ‘fingers, keyboards, mouses’. It’ll be a good microphone and a glass of water.
I think our voice boxes are going to get slightly hurt during the next couple of years. I’m always reminded by the Hollywood scenes of, you know, when someone is talking to their AI chatbot – it kind of makes perfect sense when it’s one-to-one, but I can’t foresee an office full of a hundred people all talking to their computer at the same time?
I think that would be like, I guess, a phone call center, but without any end customers.
Phil
So, that’s right. I think the other complication there is that we can often type faster than we can talk. And the other great thing is that we can edit before pressing go, which is still something that we haven’t managed that real time editing of the question that I’m trying to ask via voice hasn’t really got there yet.
There’s no such thing as “backspace, backspace, backspace” in vocal terms yet. I’m not sure if we’d want voice dictation to be “no, no, dammit, hang on, wait, stop that, no, no” – you know, the “no, yeah, stop that, pause, please”?!
AB
So, MapAI, is it cloud-based, or is it going to be local-based. How does it interrogate data sets? Do you have to introduce it specifically, or you can marry them together?
Phil
So the way that we’ve planned MapAI to work, we figured that there were enough platforms out there, whether they call themselves geospatial or not, there are enough platforms out there that have location -based information that we didn’t want to introduce yet another platform to the sector.
The way in which we want to work is to be a white-labelled solution that integrates into existing offerings that really creates a chat layer between a user and the data that a platform is already managing.
At MapAI, we don’t want to be a UI company, unless there’s a big gap. We certainly don’t want to be a data management company. The idea behind what we’ve got is that the data stays where it is at the moment.
The platform stays where it is at the moment. We just add in two new layers. One is a context layer that helps us to describe what data we can interrogate. And the other one is a question and answer API that feeds the questions from the user to us in our cloud environment.
And then we feed back the code that’s required to then answer that question for the user.
AB 11:45
That’s very smart. No one needs to reinvent every single wheel – and interfaces alone are perilous and large… and expensive! And if you can get away with, again, having the microphone at no interface, that’s a great option.
But also, same with last week’s chat, an interface-less interface: how do you tell someone what they can ask? How do you prompt the user in those early stages to do some other training, learning or exploration mode?
Phil
Yeah, that’s a good question. And the way in which we’re doing that at the moment is by focusing our early proofs of concept on quite defined applications. So rather than a free for all, come and ask any question of all this data that we’ve got.
We’ve got a defined application, which is, you know, for example, a customer service team inside a council or an asset owner trying to forecast where there might be demand for new types of their assets in the future and having a very defined use case, which is only about asking questions along that particular problem.
AB
So the users, they may not be completely across all what they can do, but they at least the data set is home to for them. They are already across what its purpose is, its intent is, this just introduced them to new ways of actually finding out what’s
Phil
Yeah, that’s right. I mean, I think that it’s easy to get carried away with the potential about where this might go in the future, but this is all still very new. So our focus has been at the very first instance, just trying to test whether or not the large language model actually answers questions in a useful way.
And so to do that, we want to make sure that the scope and focus of where our first deployments happen are really clear.
AB
Yeah, it makes perfect sense. So you say you’ve got a fine-tuned layer on top of ChatGPT. That by itself is reasonably groundbreaking. It’s pretty darn hard to do any fine-tuning on large language models.
How did the team come to that conclusion and how did the team tackle that? Was that a lot of seriously supervised training or just exploratory work to actually be able to find that extra layer to have that command set active?
Phil 14:09
It was a bit of a combination of both. And the other thing that I’ll say is that we started with ChatGPT, but the way in which we are deploying our fine-tuning model is as a middle layer that can work in between a user and any large language model, be they, you know, the ones coming out of Amazon or Meta or some of the open models that are out there at the moment as well.
Our aim was to provide a fine-tuning solution that could be a large language model independent as much as possible. That way, we could integrate with any solutions that people were already using from a large language model perspective, but would also mean that we had the flexibility not to be locked into a particular large language model solution, as we’ve seen happens with many large corporations as they get kind of more share, they can then do what they want with kind of pricing and usage and that kind of thing, and that can cripple a business that has a sole reliance on one model.
In terms of the way in which we go about creating that fine-tuning, I don’t want to say too much – mostly because I’m not the technical person here – but what I can say is that we have a whole range of question-and-answer types covering a wide variety of potential spatial concepts and spatial questions, and then start to look at how we create pairs or multiple matches between what a question might be trying to ask and what the spatially appropriate method for answering that question is.
AB 15:43
Perfect. A separation of powers of large language models of choice. By all means, if you started this concept late last year, and here we are in, well, we’ll call it May, but actually it’s the start of June by the time this episode comes out.
It’s been another few epochs even this year of iterations of not only the large language models, but even the fast following the HuggingFace open source world models, student-teacher, decimated local models that have, sure, they are a few percentage points down in terms of efficacy and brilliance, but they are local – and mere mortals like us can run them on pretty beefy units.
So this does open the door now to not having to even be reliant on the big four/the big five in the cloud. Is there a local mode in the future? For local versions of this kind of tool?
Phil 16:40
Yeah, definitely. I think there’s two approaches to that. One is the co-pilot type approach. It’s still all based on a cloud solution, but one that’s ring-fenced to an organisations cloud tendency, so nothing needs to enter or exit the fence that’s around what an organisation owns on the cloud.
And the other one, certainly a little further in the future, I wouldn’t want to put a date on it, but we absolutely talk about it, is the ability to have a model that can be deployed, you know, cloud-based, server-based, even, you know, powerful local machine-based, as that pair between our fine-tuning and a locally deployable large-language model.
AB
That’s spot on perfect. I think the pendulum is going to swing from “let’s go cloud and let’s find the value and the power of these tools” to okay, sure it’s great, but we’re now racking up not only queries, but we’re racking up internet traffic in and out to get our data in and out.
This was the question of “where is my data?” That’s right. And what are people doing with it? I imagine you’ve met the kind of people in your past 20 years who still want to see their spinning hard drives and LED lights flashing in a server room somewhere…? I dare say those kind of people are not multiplying, but there are still many fields where I want to know where my data is.
Can you give us like a use case of the kind of special queries that MapAI can help with? Pick any of your virtual customer bases, but what are the kind of unknown unknowns that MapAI can reveal that you can do that mere mortals would not really know how to do unless given this kind of tool?
So I want to start by saying that nothing that MapAI does is something that can’t be done by a mere mortal, as you phrase it. But what I would say is that a lot of the work that it does is trying to alleviate a lot of the base work that, say, our very busy GIS teams might be doing.
Responding to simple questions, like trying to get a filter of a subset of data from within one data layer, or looking to find the addresses that are around a point, or just some relatively simple one, two, three combinations of datasets and features within that dataset to understand questions around assets that might be a risk of flooding or locations to target for a particular type of demographic profile.
All those questions that are relatively simple, but in that feedback of, I need to find an analyst, I need to wait for that analyst to be free, they will provide me an answer, I need to iterate that a little bit and then get back that can take hours, days or weeks, depending on how busy a team is.
The idea here is that for a lot of those more simple applications, we can just type in a question, look at the answer, then refine the way that we asked the question.
AB
So in the bulk of digital queries, the dead easy ones are dead easy. The interface probably gives it to you. The other end of the spectrum is the gnarliest, biggest, deepest analytical questions, probably reserve that still for the analysts to do the big gnarly multi-week. “I want to find every single house that has, starts with a letter Q where there’s one dog and one cat”. That’s a query for someone who has more time on their hands, but that leaves the middle wide section of “find me all the, how many primary schools are there within an X radius at this point” or “refine this”…?
Phil 20:41
That’s right. So the common, I guess, starts to a lot of the queries that go into MapAI at the moment are show me, or show me where is, find me, summarize, or display, something like that.
AB
Yeah, gotcha. Powerful tools that are probably by definition the highest volume kind of things you’d like to know.
What state is MapAI in? The website… it’s a little bit light. So only about three pages?
Phil
We’re only… So we’re about six months in to MapAI development in terms of real time. Especially when you take out the Christmas break and all that kind of stuff in terms of the effort that’s gone in so far.
And anybody who works with the research world knows that often it’s hard to get a lot of useful work out in a short timeline for that, particularly as the problems that we often tackle with our universities are pretty big and long-term.
Saying that, the team in the City Futures Research Centre at UNSW have done a really great job in continuing to release and improve a version that can be kind of deployed and demonstrated. And so it was only kind of four months in that we had a version that we could ask questions of.
And while we don’t want to be a UI company and we don’t want to be a data management company, obviously to show that it works, we had to take a bunch of data. So a couple of months ago, we had a dump of a range of data out of the New South Wales government in Australia’s open data website, things like planning zones and properties and addresses and roads and those kinds of things.
And we described that and we put it into a simple user interface and then started asking questions of it and used that as a way to kind of refine how the model worked, the kinds of responses that it gave.
And pretty quickly, we saw that even the version as it was then could provide a lot of really useful work, say for people that only wanted to ask questions that needed, you know, three or four or five different data sets to be combined in order to be able to answer them.
Phil 23:02
So we started to have a look at identifying a few organisations that we could then prove this concept with, even with this really early work out in the real world. So we have now… And I can’t talk about it publicly quite yet in terms of specifics, but hopefully I will be even by the time that your podcast comes out, ideally.
But we’re working with a major data company as a way to integrate with a lot of the work that they create in terms of unique national -scale data products and a way to query those in a brand -new way.
And then we’re also working with two local councils, one for more of an external type application in helping to answer questions that come in from the community. Things like, you know, when is my bin night?
Or what are my planning zones? You know, can I open a hairdresser at my address? These kinds of questions. And then the other one is more of an internal use case in supporting field staff to better understand the sites that they’re going to visit.
So even with this early large language model, you can see that with each of those applications, there’s probably five to 10 questions that are pretty… Predominant. …repetitive, but site independent, so that you need to answer them in a slightly different way, depending on where you’re going.
And we can answer them using kind of a handful of datasets. So it’s with those that we’re now kind of rolling forward to demonstrate this in the real world. And having those agreed was our kind of prompt to then let the rest of the world know that MapAI existed.
AB
So we’ll put in show notes or a extra update if the podcast and the video beats this extra news. But by all means, the show notes will hold the source of truth for the latest release of MapAI.
Cheers for that. Look, just changing tack a bit. MapAI by all means is gonna be the right kind of tool for – we’re talking local councils, we’re talking service based organisations, those who already have spatial or a location bent.
I roll back to some of the other things that are on your bio, which are quite fascinating. I’m looking at FarmMap 4D, are there more sorts of tools in a similar vein? I guess we’re getting deeply into agriculture, farming and the permaculture world. Can you give us a brief about some of the spin-off companies and side projects you’ve had from Frontier SI?
Phil
Yeah, sure. So there’s probably two good examples. I can talk about Farm Map 4D and then I can talk about another more recent commercialisation, which was for a company called Value Australia.
Phil 25:56
So Farm Map 4D was actually – nd its original name was the Natural Resource Management Spatial Hub – this was the reason why I was employed by Frontier SI in the first place almost 10 years ago. And what it was was a collaboration between a range of natural resource management organisations, you know, government-funded organisations that look after the environmental management of a particular region.
Focused on the middle bit of Australia, the really kind of dry and arid bit, where we have a lot of cattle grazing. And the idea behind that was to take remote sensing data, so images from satellites where we have derived insights about the quality of somebody’s land, and take that away from being just a tool used from government to monitor the performance of land managers into something that we could put into the hands of those land managers to help them better make decisions, right?
That was the whole idea behind that. So it used a derivative satellite data product called Fractional Cover or Fractional Ground Cover that this was. What that actually means is that it simply takes a whole lot of satellite information, calibrated to a whole lot of ground sample sites, to tell you for each little square of your land over the last 30 years, how much of it is a live vegetation, dead or non-photosynthetic vegetation or bare ground, right?
So, and when you track that over 30 years, you can start to have a look at how sustainable is the level of cattle that I have in a particular paddock. So are we getting to the same level of green cover every year, or is that slowly declining over time?
We were going to have a look at how the practices then impact on things like erosion. So as we’re overgrazing land, that leads to a lot more runoff of the good soil on top, which goes into the streams, and then Queensland washes out into the reef and causes a lot of problems there.
So again, this is about the long -term trends and helping people understand things like sustainable carrying capacity, erosion risk and things like that.
AB
Up 30 years worth of data on this site>
Phil 28:10
I was working on NASA’s Landsat data at the start and then using some of the European Space Agency’s data from Sentinel to then enrich that for more recent time at a higher resolution. And it was run as a collaboratively funded project between a range of different organisations.
But as all projects do, they come to an end and people didn’t want to see the end to the solution when project funding runs out. And so sometimes having that operate as a government service is the best way for that to happen.
That’s what we do with our positioning work. Sometimes releasing something open source is the best way to get it maintained. And sometimes putting it into a company is the best way to have a sustainably managed solution.
So that was the case for the Spatial Hub, which rebranded then to Farm Map 4D.
AB
That is of immense value to know, over such a long term. I take it at the start of the 30 years, your data points were fewer and further between, but to have that such a long term smoothing graph of bare earth is slowly climbing?
Phil 29:34
And it’s really a testament to the work that NASA has done in keeping the Landsat satellites consistent and regular over the last 30 years to allow that kind of long-term history. And in fact, while we were running that project, we were highlighted on the NASA news page as a great example of using their data.
AB
Sounds like you were “employee of the month” there for a while.
To your other example – this one was a bit more recent the ‘large acronym’, yeah.’…?
Phil 30:08
The stuff with what became Farm Map really went out into the private world in about 2018, I think, and is still going and serving customers now. A bit more recently than that, we did work, again, with the team at University of New South Wales in automated and interactive property valuation.
So we ran a workshop a number of years ago with a whole range of, again, industry, academic and government people to try and find interesting problems where we thought that spatial data could play a new type of problem-solving role that it hadn’t necessarily been doing so far.
And one of the use cases that was raised by government and validated as a good industry problem as well was trying to understand when we do something new, major, like building infrastructure, like a train station, what impact does that have on property values?
And if we can get a way at the moment, that would cost you hundreds of thousands of dollars in consulting time to try and do something, answer that kind of question. Is there a way that we can quickly and easily model what it’s likely to be?
Because that then plays a critical role in a business case for infrastructure. Because as property values go up, that obviously benefits the community, but it also increases things like land tax to help to then fund that infrastructure.
So we did some work to try and work out what’s required to make that happen. And the biggest thing that was required to make that happen was an automated land and property valuation model.
We need to understand what the value is, but even more than what it is, why is it that value?
AB 32:03
Gotcha. So, the breakdown of the components of, you know, the closeness to the primary school adds this much. Was it that discrete or was it fine-grained?
Phil
We get a lot of really high-quality data assigned to each individual residential property across Australia’s capital cities, something around 10 million properties, I think. And it was a whole lot of data that was just kind of straight out of, you know, government databases or high -quality private databases, and then a whole lot of things that we kind of derived as well using, you know, geoscapes building products to look at, you know, the heights of buildings, all sorts of different things.
That then let us work out for each individual house, not just generically what might the value be, but for this house, what is its value and why is it that value? Because then when we understand why it’s that value, that’s when we can start to go about playing with future scenarios.
So either for an individual landowner, maybe looking to say, what happens if I, you know, should I renovate my kitchen or out of bedroom? What’s going to create more value for my house? Through to the government, looking to build new train stations, revitalise the quality of the local school, and the quality of the local school is a big driver of value.
Access to open space, all sorts of different things. We then built a system that let people interactively, you know, like SimCity, create that new infrastructure and then immediately see what the impact on property value was.
That was a great use and as well the actual valuations model itself was not just useful for the governments but also to banks who helped to make our risk decisions about mortgage lending and things like that.
AB 33:56
This is the holy grail, actually, of spatial intelligence. Sadly, it’s not quite the ‘hello world’ – I think it’s the first thing that everyone jumps to, to see if they can figure out how much their house is worth.
And then the way that you’re doing it is the way to crack it, of doing it large and doing it, throwing, I guess it’s, classically, the data science way. You throw all the data at the problem – you just throw the bucket at it, and you find…
Phil
…a billion data points in the model that we were running.
AB
Ouch! And then what falls out what actually was relevant, vs what was irrelevant. The number of cafes in 10 square kilometres didn’t really matter as much as hospitals and primary schools and police and those sort of things.
We tested well over 50 different modelling approaches as well to work out which ones were both accurate and explainable. Because having not just an accurate model but one that you could talk about with somebody was also really important.
Phil Delaney
AB
Yes, when computer says X, that’s nice. But in this vital field, you need much more than that.
Phil 35:02
Yeah, that’s right. So we put that out into a company two years ago. Andt we needed some people with great data about land – what we’d really built was the engine – we needed someone with fuel.
And so we ended up commercialising the company with PEXA, who are a digital conveyancing company in Australia. For those not from Australia, every time you buy and sell a property, the transaction will go through PEXA’s infrastructure.
And so it really complemented a lot of the work that PEXA were doing, trying to diversify what else they understand about land. And so that Value Australia company has seen a great life growing as a commercial entity over the last couple of years as one of PEXA’s family.
AB
The digital marketplace is certainly a beautiful theory and when one organisation can not only be the transaction hub but also be the value add, it’s a bit of a slam dunk that makes a perfect marriage there.
Can we change tack one more time? One last time from acronyms and long project names.
Now, I said earlier, I do not have in my head what this acronym or wording is but the “Australian uplift of GPS”. Could you give us a briefing on that? Your involvement and where you see the future going?
Phil 36:28
Sure, so the generic name of the system is SBAS, which is Satellite-Based Augmentation Service, and Australia’s SBAS is called SouthPan.
Southpan is is a way for us to broadcast from space a correction signal to satellite positioning like GPS. There’s a number of different constellations up there operated by different countries or consortia, like the European Space Agency.
And every signal about positioning has an error that happens because the atmosphere changes over time. And so, when we position ourselves on our phones or on our cars’ GPS or whatever that might be, we generally are only accurate to 3 to 5 metres, which is great to know approximately where we are in the world and what road we’re on for navigation purposes.
AB
…just enough to be able to get you what lane you’re in, but that’s actually about the limit, yeah?
Phil
Not even – sometimes only just the road, because three to five metres is enough to put you on the other side of the road.
AB
Which would be bad.
Phil
And so what we needed to do was to work out a way to correct each of those signals.
So what we have is a bunch of really well-known locations around Australia and New Zealand, where we know really precisely where they are. And what we do is we collect all of the signals from the space -based positioning and say, well, for this, you know, for the little region around where this precise thing is, we know that the position from satellite X was out by about this much.
And so when you collect all those things together, you can start to greatly bring down the accuracy of the satellite position. But then you’ve got to go get that to the user.
AB 38:26
To find a way to tell someone that they’re off?
Phil
That’s right, and that’s where an SBAS solution comes in. So there are other SBASs around the world, and they’ve primarily been brought in for aviation purposes, so help with safety of life of autonomous flying in planes coming in to land more safely in airports and things like that.
But in Australia, we didn’t have enough airports for the use case to stack up just on flights.
AB
We talked about the ‘big hole in the middle of the continent’. Here in Australia, the population of people not on the coast in this continent be measure in hundreds of thousands.
Phil
So what we did a few years ago was work in partnership with Geoscience Australia and with Land Information New Zealand to get a pilot of this signal going from space, so where we got a communication satellite, we’re able to broadcast that correction signal, get some devices down here that could read and understand that, and then we deployed that across 27 different projects, I think on nine different application sectors across the economy in Australia and New Zealand, and then did a rigorous approach to look at what was the economic benefit in having this position, this kind of 10 centimetres, versus having three to five metres in agriculture, in resources, in navigation applications for the blind, in drones, in augmented reality, all sorts of different applications.
And essentially we came out to a figure that I think was around about $6 billion of benefit over the next kind of 10 or 15 years that was all driven just by having a much more precise location. So the government then used that to get operational funding for that, and that’s now a service that’s available to anyone really across the Asia Pacific region to access, if they’ve got an Airspace enabled device.
AB
Gotcha. So first off, gold star for you. That’s phenomenal. Secondly, what kind of device is needed to receive this correction signal as well? Is it beyond consumer products or is it coming down to that level soon?
Phil
And most consumer products at the moment, although we’ve got a range of open source kind of examples as to how people can build something, it’ll cost kind of $1 ,000 to $2 ,000, as opposed to maybe $50 ,000, which is what it was beforehand to get that kind of accuracy.
I’m saying that we also have another program that we’ve been working on with Geoscience Australia, which is called GNAN, which essentially distributes those same corrections but over the mobile network, so via internet connection.
And for that, the accuracy is actually better. It’s down to around two to three centimetres. And in theory, we’ve got that working on Android devices. So we haven’t kind of mass marketed that on Android yet, but Android lets you have access to the raw positioning data so we can correct it here.
And we’ve had that kind of accuracy on Android phones and tablets. Hopefully, Apple might do the same kind of access to data at some stage, but… opens APIs. Yeah, but then you have the gaps in the marine areas, in the areas that don’t have internet coverage.
You aren’t able to get that correction, but anywhere that has mobile reception, we can start to then incorporate that in our daily applications.
AB
Is this a worldwide phenomena? Is this a movement that is going to be coming in the next two or three years that it’s just a matter of time and pressure and priority?
Phil
Ideally, yeah. I mean, there’s nothing that stops it working anywhere in the globe except for communications. And most of those accuracy correction services exist in most places around the world. So it’s certainly coming more and more.
As with any major new capability on, say, a mobile device, you’ve then got to balance things like battery performance. Because as you can imagine the compute required to go from what road am I on to where in the lane am I is substantially is a substantial increase.
AB
So it’s about spheres of influence and how good do I need to be right now? So if you need to be, yeah, choosing the right lane on the highway, that’s kind of neat. And I imagine most GPS’s are just guessing.
They assume you’re on the correct side of the road until you’re not and then politely inform you to come back. Gotcha. So might there be a future where just as we see world time service to, you know, go and check with the world clock or world clocks, would there be world time GPS grand station correction services as a…
Phil
Yeah, absolutely. And like I said, you can commercially buy them in many places in the world at the moment as well. A lot of the driver behind this was to make those even more accurate, but to also make them take away that cost barrier so that the benefits of that kind of accuracy could be available to anybody.
AB
I was just overseas in a place (that I didn’t tell my wife till afterwards) was the worldwide center of GPS spoofing.
Phil
That’s another topic entirely that we probably don’t have time for!
AB
We don’t, indeed. Phil, thank you so much. Absolute pleasure to have a chat.
What a seriously wide range of topics and projects you’ve been involved with. Not only through frontier SI, but deeper and darker history, your bio on LinkedIn is phenomenal.
https://www.linkedin.com/in/phillipdelaney
If you’re interested in MapAI, please reach out to Phil. We’ve got as a links to MapAI and there are, I believe contact details on for you on that website.
So just final thoughts: do you think that this year is going to be a groundwater year for, language being the tool of choice – is that where that AI is trying to strive for its future customers?
Phil 44:37
I hope so. I think if we go back, you know, 15, 20 years, Google Earth and Google Maps was a major explosion in the use of location-based information by everybody. And, you know, at the time, I think a lot of people were wondering whether or not it would be a threat or an opportunity, but we’ve got a lot more demand for spatial applications now that people think about spatial as part of their daily life.
Really, I hope that that combination of simple kind of chat and large language accessibility combined with location will be the next, you know, major inflection point for how useful location -based information can be around the world.
AB
Hear hear – I fully support that idea that I guess that’s why we’re here having a having a long chat.
Phil we all really appreciate it. We’ll let you get back to your day and for those listening and watching along, everything’s in the show notes. But for now we’ll sign off on this episode of SPAITIAL.
Phil
Thank you for your time.
HOSTS
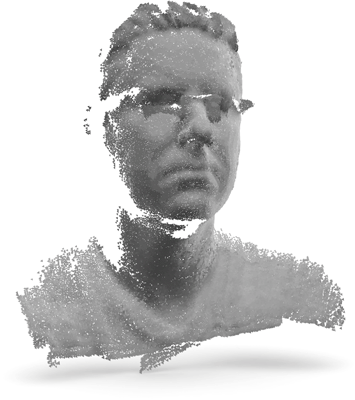
AB – Andrew Ballard
Spatial AI Specialist at Leidos.
Robotics & AI defence research.
Creator of SPAITIAL
To absent friends.