Is point’n’click end-to-end low-code spatial AI… possible? What about *no code*? Spoiler alert: the answers are yes and yes. Knowledge of development patterns and code syntax and how many words you can type per minute are no longer barriers to entry for most of us. It’s time to get our hands dirty with spatial data!
This episode features Grant Case, the Vice President of Sales Engineering at Dataiku for the Australia Pacific Japan region.
AB and Grant discuss Dataiku’s AI platform and its capabilities in handling various data types, including structured, unstructured, and spatial data. Grant highlights Dataiku’s ability to cater to different user personas, from low-code and no-code users to pro-coders, through its intuitive interface and integration with open-source libraries.
On a wider note, we explore the advancements in large language models (LLMs) and their impact on data analysis, particularly in the spatial domain. Grant shares examples of how Dataiku leverages LLMs and digital twinning to enhance data understanding and decision-making processes. The conversation also touches on the role of Chief Data Officers, data governance challenges, and the trade-offs between building custom solutions and leveraging existing tools.
Connect with Grant on LinkedIn at: https://www.linkedin.com/in/analyticseverywhere
We’re also publishing this episode on YouTube, if you’d like to watch along in full living colour: https://youtu.be/1EU042y4_7A
Chapters
05:17 – Dataiku’s AI Platform and User Personas
Grant explains Dataiku’s AI platform, which caters to different user personas, from low-code and no-code users to pro-coders. The platform aims to bring these diverse users together across multiple technologies, allowing them to work in their preferred manner. Dataiku has been recognized as a leader in the Gartner Magic Quadrant for its completeness of vision, particularly in catering to low-code and no-code users.
10:16 – Advancements in Large Language Models (LLMs)
The conversation shifts to the advancements in large language models (LLMs) and their impact on data analysis. Grant discusses how LLMs have opened up new possibilities for unstructured data use cases, such as natural language processing (NLP) and spatial analysis. He provides examples of how LLMs can assist in tasks like understanding business locations and mapping data.
22:36 – Digital Twinning and Spatial Data Analysis
Grant highlights the concept of digital twinning, which involves creating virtual replicas of physical systems or environments. He discusses how digital twinning can be applied to various domains, such as disaster recovery, infrastructure planning, and manufacturing. Grant also shares examples of how Dataiku leverages LLMs and computer vision for spatial data analysis and decision-making.
35:45 – Open-Source Integration and Deployment Options
The discussion touches on Dataiku’s integration with open-source libraries and its deployment options. Grant emphasizes Dataiku’s ethos of being open to both proprietary and open-source technologies, allowing customers to choose the best solution for their needs. Dataiku supports cloud, on-premises, and hybrid deployment models to cater to different organizational requirements.
31:15 – Data Governance and the Role of Chief Data Officers
AB and Grant discuss the challenges of data governance and the role of Chief Data Officers (CDOs) in organizations. Grant acknowledges the ongoing struggle with data quality and governance, highlighting the importance of proving the value of data and AI initiatives to secure a seat at the executive table.
36:36 – Build vs. Buy: Leveraging Existing Solutions
The conversation explores the trade-offs between building custom solutions and leveraging existing tools. Grant advocates for evaluating whether a solution provides a competitive advantage or solves a unique problem before investing in building it from scratch. He emphasizes the importance of focusing on value-adding activities rather than reinventing the wheel for solved problems.
45:29 – Future Developments and Retrieval Augmented Generation (RAG)
Grant shares his thoughts on future developments in the AI and data analytics space, including the concept of Retrieval Augmented Generation (RAG). RAG involves combining LLMs with an organization’s own data to provide more contextualized and relevant responses. While RAG offers a way to quickly derive value, Grant acknowledges its limitations and sees it as a waypoint rather than the final solution.
Transcript and Links
AB
Well g’day, and welcome back to SPAITIAL. This is Episode 24, coming to you after, yes, a minor hiatus again. Apologies, I do often have things called ‘work’ and out-of-town-isms. Apologies, but we’re back on a regular schedule with this episode and one booked in for next week and the following week.
With me today I have the great pleasure of chatting once again, not with SPAITIAL, but back in old-school territory here with Grant Case. Grant Case is Vice President, Sales Engineering at Dataiku, Australia, Pacific, Japan.
Oh look, your title is long and varied. I’ll let you introduce yourself. Grant, welcome to SPAITIAL.
Grant
Thanks, Andrew. Hi, everybody. I’m Grant Case. I am the Regional Vice President for Sales Engineering here at Dataiku. For myself, I work in the Sales Engineering / Solution Engineering space, where I spend most of my time with clients across the region, but particularly here in ANZ, where we talk to organizations, both large and small, in and around analytics and AI.
I’ve been in with Dataiku for the last six years, but I’ve been doing everything, analytics, AI. Before we were calling it AI, it was all statistics, right, Andrew?
AB
It’s just math in the end. It’s just math.
Grant
It’s just math. It’s ones and zeros, but I’ve been doing it for the past 20 years across multiple different industries and quite a bit of that. Spending time within different domains, whether we’re talking about NLP, we’re talking about just machine learning, but also GIS has always been a very interesting background and interesting set of projects that I’ve worked with.
So happy to be aboard.
AB
So six years. Six years at Dataiku. I must say at the outset, I’m going to do the Australian way. I mean, you do the North American data.
Grant
I come from Queensland, right? Northern Queensland?
AB
VERY far north Queensland. But obviously from the US. For the record, Aussies versus Americans, you know, the ‘data versus data’. So we (we Aussies) have problems talking about data, the character in Star Trek versus data. That’s always wrong. But at the same time, we can, we can figure out the difference between ‘routing’ and ‘route’, which is nice. So we, we lose on one tech term, but we gain on the other. So that’s exactly, it’s a net neutral.
So that’s nice. Six years at Dataiku. I think I was chatting to you pretty much the month that you started. This is back before COVID BC and before the current sort of situation. You were, look, Dataiku was one of those tools – is one of those tools – that for me is still pure magic to sort of, you know, Vaguely quote Arthur C. Clark, you know, technology that’s in just indiscernible from magic is just, you know, a joy to use. And that’s really what it is. It’s a walk-up tool that does everything data related.
And yes, ‘everything’ is a big claim, but it really is. I’ve been describing to people with the catchy non catchphrase of it’s the no code, low code and pro code. It sort of doesn’t lock you out of doing the hard way if you want or doing a low code, which is the title of this episode – and a topic we will definitely come back to.
So if we do manage to low code is the graphical point click, no less powerful, but certainly if you need to do something quickly, look that goes to that goes to that, but the, uh, thing that really blew my mind and you’re probably going to blow my mind even more cause I haven’t caught up with the Dataiku world for a year probably — is the no code just press a button and have everything done for you? Do you want to give us a rundown on where Dataiku is and where it sits? What the other, yeah.
Grant
I’m gonna put you right in front of a client because you did a great job of it. So as a platform, we look at organizations today and try and understand that there are different levels of maturity, the different personas.
So anyone from just someone who just needs to consume the dashboard all the way up to someone like yourself, Andrew, that’s getting in, messing, fine tuning on different NLP algorithms, they all need to work together, right?
So Dataiku is the AI platform to bring all of those individuals together across multiple different technologies. So nobody, everybody works the way that’s best for them. Obviously, just from a pure number standpoint, we have more low code and no code users.
That’s just the way of the world, right? But the need is for everyone to be able to access. So yeah, so we’ve actually just announced as a leader in the Gartner Magic Quadrant this past year and are a few months ago and most, we are the furthest along on completeness of vision in many ways because of what the title of this episode is talking about those low code and no code users and how do you bring some of the sophisticated things that we were doing, you and I were maybe doing five years ago, maybe even two years ago in code. And now it’s run of the bill, anyone can do it.
AB 05:59
And those three different levels of approaches are vital because, yes, even if you have someone doing the pro code, the full on way, had you passed that down neatly to people who just want to get in and use the damn thing.
And then there’s the moral majority who don’t even know that they want to use it. You can have them in the no code mode where they just press a button and it’s done for them. They don’t even need to know the magic is just there.
And I think that’s, yeah, the realm of the data scientist/data analyst has traditionally been a bit of a lone wolf, not by choice, but by I’ve set up my environment, you know, how many years ago would have been my hotted-up PC with a water called GPU was the way to go. Those days have gone. Thank goodness. Then it was okay: I’m buying 35 minutes of time on a beast of a machine in a cloud. And now it’s like, okay, I’ve got to play nice with the rest of the organization.
Quite a quite a challenge to flip that around. So it’s nice to just have a place where people can do what they need to do. And if need be, and I’ll say this nicely, get into what they need and then go back to work as opposed to spend all day going.
Grant 07:18
Yeah, for, you know, and to be fair, especially as we talk in these different problems, right, problem sets right now, what ends up happening. So I’ve been around this block enough times to know that the things that the pro coders are used to do, it was only them.
Now that becomes run of the mill. And now those folks don’t want to deal with that. So, you know, if you, over time, then it becomes, hey, it becomes a problem. I need the data or need you to do this or add this in.
And to be fair, Dan Kenny, who is a good friend of mine, and in the United States, he is the commercial data science leader for all of the handsome pharmaceuticals, the division of Johnson and Johnson, he makes a comment, you know, especially pro coders, they want to do one turn of the crank, right?
So they want to get it to run. Yeah. And then they want to go away. But if they’re sitting there maintaining it, what happens, they get bored and everybody that wants to do something, they got to do it, you know, and they’re banging on the door, right?
AB
So I’m not a builder or an architect, but – I’ll again take out the dagger from the back in a second – it’s not nice to have to be a plumber or a tradie to have to maintain the thing for the next 20 years. Yeah, it’s nice to be able to drop it, leave and then move on.
Dataiku has traditionally set and its sweet spot is tabular data, Excel, spreadsheets, databases, text numbers, the whole lot. Again, brilliant example that might blow some minds is you can load up and do your data engineering mashing on the fly fixing of data and just say, I’m going to go to lunch, but I want to figure out why my sales or my widgets are working and do all the models in the next hour, even though you probably know that half of them are ridiculous, but do it all. And by the time you come back, it’s all been done. Sometimes it can confirm what you’re thinking and you get internet points.
Sometimes it can actually validate or invalidate what you’re thinking and you might learn something. So tabular is home turf without fail. I know you were just moving into multimodal, into images and rich data, rich media, especially with NLP and audio there a few years ago.
AB 09:40
Can I stretch that question into how far are you now leaning into richer multimodal, deeper nested data asterisk into spatial data?
Grant
I think that’s a great question. And, you know, we always hear about the 80% of the data is sitting in unstructured locations. I think the one thing I’ve seen over the last 18 months, and this was with GPT -35 coming on, I had started to play with GPT -3, uh, when it came out and I was, it was really blown away.
But once it got into, you got into a service and open AI, really releasing that, that’s when pretty much all of these unstructured use cases completely opened up, right? Because this was something that was really hard, took a lot of effort and really expensive.
So you and I were both working on a use case a few years ago, where you’re, in the back corner and doing something and it’s really edge, now that same use case, you know, I can pump that into, you know, llama three one out of the box and I’d probably get better results out of it today, right?
With no fine tuning. So to me, the, what we have seen over the last 18 months has really opened that up. And especially when it comes to spatial, right, where, when we talk about spatial, the first use case I ever heard about, you know, true, really interesting use case for me, I worked personally, I was doing, where do I put business bankers across the country?
Because in a former life I was working for Citigroup and where do I was taking all of this tabular data and trying to bring that together and understanding, but think about that, you know, that same problem.
Now I can actually just show it them. I can show something like the map, right?
AB 11:44
…and say here’s the continental USA, how that indicates density, coverage, yada, yada, fine with population and GDP, where my next five pins are going to go. It’s going to have a red hot guess and chances are it’ll get pretty close. Pun intended. It won’t be all. It won’t be hallucinating. It won’t give you all in Alaska, all in Hawaii. It will actually give a reasonable answer for the human to then come along and assess and figure out whether that’s an excellent guess. A lot of questions might get you there. By the time you’ve, you know, had your first cup of coffee for the day, problem could well be solved.
Grant
And again, I think that is the key when we’re talking about this, is the lot of the legwork, especially on the spatial side that you would have to do to get this stuff ready for a traditional machine learning model, even, you know, 18 months ago, two years ago, is now gone.
AB 12:47
So even doing boring – adding lat-longs to a tabular dataset and starting to say, okay, now draw me a map, now get, you know, a few years ago, that would have been incomprehensible. The data chain would have been dodgy, hard work, and three weeks later, you would have been tearing your hair out.
Grant
I’ll give you a good example. So I was working with an airport client here in the region. And part of the proof of concept is like I needed lat longs for a number of different airports across the region.
I gave it to the, I just pumped it into the LLM. It’s like, we don’t have to go search for that data. Obviously it’s out there someplace, but it’s sitting inside the large language model. And you just ask, here’s your row of data.
Give me that answers in and around it. So for me, especially when it talk, when we talk about that low code, no code user, and that use, and that was me at the beginning, a lot of beginning of my career as an analyst is how do I, as you say, how do I get my job done and I leave and I go off, right?
So the LLMs have really opened that up. And especially for any sort of GIS analyst at this point, you know, it used to be, to do this sort of work, you’d have to go have an Esri or an ArcGIS to do a lot of that kind of low end work.
Yeah, we saw a little of that visualization come in with the tableaus and clicks, and I could start to overlay a couple of maps, but now. I can’t, we can skip those tips. Yeah, you can skip them. And that’s amazing. That’s amazing.
AB 14:27
Yeah, I love the fact that GPT 3 .5 was good, was great. You could ask it to write the entire essay, all of what it wouldn’t. Go crazy by the end, it could do three, four, five thousand words and keep on point, but it was slow.
You could almost watch it as it was forming the words. At the start of this year, we basically went to Crazy Fast and I must say that’s been another epoch that we’ve talked about here on the show, previous episode, where ironically now it’s absolutely human to try and catch up with what you just asked.
You can say give it to me like this and bang. Okay, give me a few minutes now to figure out what you just did and ask the next sensible question. Before you had time to formulate the next sensible question, you almost had time to think about five questions in terms of going so slow or smaller chunks.
You can now on Anthropic Claude: You can say write me a web app on the side that does this and I can deploy it to blah, blah, blah. That almost is three months old, that kind of technology. We haven’t seen the same from OpenAI. Sure, they’ll all be copying and releasing any day now.
I think to your point, these epochs, the epochs are increasing in, you know, decreasing the amount of time between them, right? So it used to be two months. Now we’re seeing it every two weeks.
Grant Case
AB 15:55
Yeah. The chat leaderboards for LLMs are taking off. They have subcategories in them for math, for text, for understanding. They haven’t yet, although I’ll start a petition or I’ll start a bit of a trend for spatial, but certainly spatial in has, it’s got a few meanings, so by means happy to take any of them.
One of course is that geospatial mapping, laying out, understanding concepts. You would have to run to a graph database earlier to figure out this is connected to this. You don’t need to know the highway between town A and town B, but there is a line between them.
There are relationships between these dots of the map. They’re not just random noise. We’re now going to not just two -dimensional spatial, but three -dimensional spatial. What is behind that? Can you figure out what’s behind the chair in this image?
Things like that are now getting towards possible. Is data Riku going down the path of open format, open APIs, and connecting to anything and everything? Where is its special source? Where’s the sweet spot that it’s coming up with its, I guess, architecture?
Grant
And I think that’s a, you know, for us, and this has been a long term strategy. So you can go back 10 years from our founding. And at the very beginning, it has always been, how do we make the best use of whatever compute resources, you know, storage is available for the client, because our secret sauce is getting every people, people together, and actually being able to use and do things.
So when we talk about, especially, you know, open source capabilities around GIS, if we’re talking about data sources, if we’re talking about LLMs, there is a there’s value, especially for many of our enterprise clients being able to do all.
So right now, I’m working with a customer in the Singaporean government, they are hyper, you know, hypersensitive about being in the cloud, they need to be completely on prem. Well, you’re not going to be able to use open AI, right, that are anthropic, but you are going to be able to use something like, you know, llama three, one.
Yeah, right. So yeah, so for us, it’s playing all of the fields, knowing full well that, you know, as a as a technology, obviously, we, we sell our wares into organizations, but we make use of a lot of open source capabilities at last count, I think we had about 349 open source libraries, packages and applications as a part of our acknowledgments.
But that also goes to our ethos is, you know, hey, we don’t necessarily we’re selling our wares, and we’re not open source ourselves, but we also have to be able to be as open as possible to others. I know just from an ethos perspective.
Yeah, you know, if, if you go down, if, as I tell many, if you go to scikit learn, it’s a website, and you go scroll down to the bottom, there’s all, you know, here’s Microsoft and Nvidia and a couple of others.
Grant 19:10
Here’s a little known fact about Dataiku: both it and scikit learn started in Paris. That’s where all where we started. And that’s where our four founders, everybody knows each other that ecosystem. So so again, I think for us, and, you know, to be fair, anybody, you should be open to both those closed proprietary and open source.
And I see the value in both. But, you know, as we’ve seen, this stuff’s moving so fast, you got to be able to go both.
AB 19:41
That’s it, the rate of change. Yeah, so by staying agnostic, by staying ready to just embrace and roll with, you know, all of the different possible options. I can recall a version of data IQ I play with early installed locally.
I guess that’s still achievable, but not as the main course. Is the mainstay cloud one, two, three, or is it, yeah. Still both. So again,
Grant
So we now have cloud. And again, I think this is indicative of the entire ecosystem, right? So you have to be able to do both. Some organizations have completely gone to the cloud. So one of our partners, Databricks, or two of our partners, Databricks and Snowflake, they’re both cloud -based systems and they don’t wanna do anything on -prem.
But we as an organization know that you need a cloud instance for those who need to start and go. And guess what? You can be up and running in 20 minutes and starting to do your work. Flip side of that is you may wanna run on your local machine, as you well did.
And then you can run in the cloud. So it just, and run on a self -hosted or even on your data center. If you’ve got a Hadoop instance still out there and gotta help you, if you do.
AB
OK, so, but COBOL is finally out, is it?
Grant
Yeah, it depends. There’s still people using it. I don’t think anybody’s doing anything new.
AB
I did end up playing with a COBOL, a client who was using COBOL. And my first question was, hang on, are we talking green screens or orange screens? I said, oh no, orange. Oh, wow.
Grant
Oh, yeah.
AB
…there is hope. It was pretty rough. That was a, you know, 30, 40 year old bit of technology that should have been upgraded, you know, 37, 27 years before. Good claim fun. What are the sort of things that you’re playing with?
What are the new things that expand your mind? I know, you know, the data IQ realm was one of the tools six years ago that really, you know, showed me that there are much faster ways or more the point, I’m very happy to bury my own, my old code knowledge, keep it as a pattern or as a, yes, I used to know that language back would all that technique backwards, but I was more than happy to say goodbye to skills in favor of reaching for the next skills.
One of the things that really have expanded your mind and, you know, mind equals blown for you.
Grant
Mind = blown for me, too. I think right now things so funny for me is one of the more interesting things I’m seeing right now is digital twinning. So digital twins were the idea and the concept to have just, you know, I’m mimicking whatever my infrastructure is and how do I start to interact with it.
GHad a great conversation with John Blick, who is one of NAB’s kind of thought leaders in this realm, probably about two years ago, and he talked about how do you encompass an entire knowledge base of someone into and make that available.
In effect, he was talking about this concept and idea of LLMs maybe a little bit before his time, but this idea of how can I encompass an entire, you know, system itself or even a person in a way that makes I can start to interact with it.
To me, that’s probably the most interesting aspect I’m seeing right now.
AB 23:18
…as in a replication of a city, but as of a… It could be both. A systemic. Yeah.
Grant
So if you think about it, I can digital twin my network, I can digital twin a city, I could digital twin an oil refinery, I can now even really getting into the concept of digital twinning an individual.
So think about, yeah, that’s the crazy part about all of this. So for me, being able to encompass all of that makes for an incredibly interesting. So you start thinking about how do I interact from just to kind of play in the GIS space, disaster recovery.
A tsunami hits Northern Queensland, right? You know, what infrastructure is impacted? You know, what are the chances or what are the probabilities that a substation is going to fail in this area? We saw this in Cairns, what?
Probably three or four months ago with all of the flooding, right? You know, a lot of what’s going on and what’s happening is being able to look in and understand the impacts of those well ahead of time.
We’ve seen that, especially in, if you go into SCADA systems, into places like oil pipelines, refineries, going into electrical systems, these are things that have always been there. Yeah, yeah. So to me, yeah, go ahead. This is going.
AB 24:47
Beyond theory, I know in manufacturing circles, having sensors in places using AI to do outlier, when you have a bad day, you can circle on the chart. That was a bad day. Tell me when we are approaching a bad day again.
But those are full of sensors. They’re already connected by virtue of they’re the things that are turning things off wrong. How do you translate that up to, well bank sized or system or enterprise? Is that still, here you go.
How many years in the future are we talking or is here in?
It’s here. It’s now. So a great example of this is Western Digital is one of our customers. And they have a high speed assembly line, a manufacturing line of hard drives. So they have computer vision cameras sitting over the top, going through them.
Grant Case
And it’s immediate, you know, just being able to use the sensor, being able to do that computer vision, understand if there is, you know, some sort of circuitry that has, you know, a defect, being able to immediately pull that off the line.
That’s here today. There is, you know, steel manufacturers being able to understand based and this is all based in, you know, chemicals and numbers and all of that being able to understand, you know, what the hot roll is coming off and what is the purity of that steel and what’s necessary.
That’s an, you know, that’s being done right now, you know, by just modeling instead of somebody having to take a measurement.
AB 26:21
Yeah, so rather than having to rely on, yes, years of knowledge and a bit of a finger in the air and feedback that follows information, being close to real time and change the settings.
Grant
That’s, you know, you said like, Hey, without years of training and experience, I still think that that training and experience is still incredibly valid if it’s kind of the fact you have to know what the underlying math is to understand, you know, what’s impacting your problem, right?
So there’s still, maybe I don’t have to, it’s in many ways, yes, a lot of engineers can sit down and still do, you know, calc three and differentials and all of that good fun stuff. But, you know, there’s a lot of applications that can help.
AB
So it sounds like humans are rising up the knowledge curve and passing more and stuff, more, more things down into the data realm. What are the, what are the roadblocks to that? Data quality, data governance.
Same old, same old. Or can we just go straight to the LLM, a enterprise LLM and have it, have read everything that our organizations done and give a semi -decent answer to a really dumb question.
Grant
Yeah, well, again, I think, you know, it’s always garbage in, garbage out. Right. So, and to be fair, what I’ve seen from the LLMs so far, they don’t particularly do well with, you know, here’s a tabular set of data and go figure out the numbers.
If you upload something into an LLM, what is it going to do? It’s going to try to do a bunch of statistics and math and try to create those insights, right? Those are the sorts of things we can do anyway, but they’re not as great with math.
And, you know, with your context windows being so small, you think about a, you know, a good size Excel spreadsheet that would be beyond pretty much the context window of an entire model, right? Now start adding 250, you know, 250 sheets to that or, you know, doing a year’s worth of data on it and you start to up.
So to me, there’s still value there in understanding kind of both senses of what’s going on and what’s happening.
AB
Yeah, no, their windows are expanding rapidly, which is nice, but they’re definitely not database size. There’s a reason why we have large stores of databases and highly redundant. But I can recall that DataIQ would always take a look down in columns and have an educated guess at what things were.
Can it start to do that with richer datasets now? Can it sort of be looking ahead and saying, so file -based systems and interacting data and pulling that into, is it a central file format that it uses, or it just describes what it sees and the human to come along?
Grant
It’s a lot of description of what we’re seeing. So let’s take the concept of structured versus unstructured. We’ve been adding additional functionality into the tool to understand, obviously, the descriptive statistics, min, medium, mode, max, standard deviations, IQRs, all of that good fun stuff when we talk about numbers.
But what we’re seeing now is we’ve been adding this functionality as what you do with unstructured data. Because typically, that has been, what is the data drift inside of a column of text? That becomes an interesting question.
That’s impossible to ask.
AB
would be a rough one.
Grant
…but that is now, you know, available, right? So, we’ve got, you know, part of being able to understand an LLM is to evaluate the drift and what, you know, what it’s responding with, right? So, to me, this becomes the interesting aspect is now a lot of that feedback, you know, turning the unstructured into structural.
So, whether that is, you know, describing what it is or describing the governance around of what it is. So, you made the comment, do we need to know data or do we need to do a data governance? Guess what?
It’s always there. It’s always been there and it’ll continue to be there. I tell this story when I’m on stage and I ask, I tell, I went into a customer’s office and this, you know, CDO is telling me, you know what?
We’re not ready for kind of AI, machine learning, predictive analytics, because we can’t get our data right. We need to get our data right first. That was 2014. You know, that was 10 years ago. And, you know, it’s still the, I still get the same feedback from folks.
So, it’s an ongoing problem. But it is a show.
AB 31:38
I’ll not name an organization that I had contact with for many decades who was on, let me go, their fourth version of an enterprise data warehouse. That’s a fun story. Version two should be good, but version four, hang on, at some point in time.
Grant
Well, I think I did it this way, right? There’s a lot of, and this is one of the things if you, the average data executive is in seat for roughly two years before they’re moving on. So it’s roughly about four months as the standard deviation.
So if you think about it, the average, uh, that, and it takes you a year to be able to get to the nuance, to get the nuance, in effect, most people are going away and you start to understand why that is the case because people, it’s much easier to discuss, Hey, how do I get data from one location to the next?
It’s a solved problem, right? It’s an expensive problem. It’s a long-term problem and it’s a long running problem. But no, if, as you say, if you’re going through your fourth one, there’s a lot of people that are still going back in time and still trying to get value out of that.
AB
Is there a good news story with the Chief Data Officer, are they moving on and upwards or are they running for the hills and saying never again?
Grant
I would say, um, I think for most organizations, the CDO, um, and now a lot of times you’re starting to see if chief data and analytics officer, we’re starting to hear noises about the chief AI officer, all of these particular roles, they’re coming, they’re starting to, they’re not going anywhere.
I think the, especially for the CDO there, I’m starting to see them more underneath a CIO or they’re going to the office of finance. They’re not necessarily reporting all the way up. So for them, it’s, you have to show your wares.
Maybe you don’t, you know, you’re close to the table, but you’re not at the table at the moment. So, but for those CDOs, those CDAOs that are proving out value, guess what? They’re the ones that they’re the ones pushing these AI projects, you know, whether we’re talking about things in the spatial areas, such as, you know, doing an, you know, map analysis, computer vision, you know, even things as simple as, you know, boxing and understanding, you know, the number of cars in our competitors, parking lots, right? And all the way to, uh, the flip side of that, just the basic, how do I get data in and data out?
But you have to have all of that in order to have a self -sustaining data and AI strategy. And if you don’t, well, you’re going to be looking for a job in, you know, 16 months, right?
AB 34:43
I hear you. Last two questions were actually good around the topic. I think you mentioned it there for a second. Solved problem. I guess in our look, our both our bids, I think we paint on the gray hairs every morning, correct? Yes, they’re not really gray hairs. Good, excellent. There’s a lot of belief in starting from scratch. There is a good percentage of our population who says hardcore, no, notepad, insert tool here. I shall reach the book.
I’ll do it the hard way versus let’s skip 90% of that. I’ve been using the metaphor of God of the days to have to learn how to understand how the mystery of an internal combustion engine works before you can drive a car.
I just got rid of my last internal combustion engine vehicle, which is good, because when the change or light came on, I think it was the same episode. My mechanic was off the same mold. It’s like, you know, Jerry, Jerry, Jerry was, you should be stopping by the time this light comes on.
Then why does the light only come, there’s a level of understanding that is either you do want it and you go back to first principles. I know many people who enjoy that, but I’d love your point of view on the new benefit of just saying, given granted, if there’s a red button for it, I’ll press it.
If there’s no button for it, I’ll just ignore it. I just want to do the thing and I want to do the thing faster. Is that the kind of world that you were saying more and more?
Grant 36:19
I think it is, um, I talk, you know, it’s this concept of build and buy, right? So do I build it myself or buy it? And I did a presentation, you know, a few years ago, talking about that. And to me, it becomes, is it a bespoke?
Is it something that is going to give your organization competitive advantage? You know, is it something you can’t readily find out in the marketplace? Guess what? Go build it. It’s, but there are so many solved problems, Andrew out there that I’ll talk ETL for a moment, there are plenty of tools to do ETL and, uh, that will do it quickly, but some people will die on the, die on the Hill that they need to write it in SQL, right? That’s not, you know, that doesn’t return value to the business.
AB
No, it’s an admirable sideshow to the important superhighway that’s right there to your left.
Grant
Yeah. But again, if I’m doing that work, it makes sense for me. And I think this is where, if I am a leader inside of an organization, I have to understand what is valuable to my organization versus necessarily what is valuable to my people.
I’d love to have that interaction so that they’re the same. But if your team is doing nothing but building stuff, what else could they be doing with that? And guess what? If you can’t, then that’s going to be a problem.
AB
Speed is of the essence as we’re saying the gap between epochs in the field that we’re thankfully being able to, you know, take along the side. The field is reducing down to less players doing more of the things with a healthy open source copy paste of, you know, teacher student models actually models.
Grant
I got a good question for you. Do you think we’ll see more or less LLMs two years from now?
AB
Hmmm, there will be more by number. I think those top 5-10 companies will still be the only ones who can get speed to market. There have been a few initiatives of universities trying to pull together open source funding to, you know, do some mega training on the big foundational models.
I haven’t seen those come to fruition. And if they have, they definitely haven’t hit any leaderboards. Though I think it sadly is in the realm of Nvidia shares are probably the way to go. It’s not financial advice.
Grant
In a gold rush, you know, so pickaxe picks axes and shovels.
AB
Yeah, because we’ve gone beyond how to measure it, centuries eons of computing time to train these things. We’ve kind of run out of content. There has been a side discussion of what happens when AI starts to have to read previously written AI as new content fodder and is that gonna start a vicious cycle and race to the bottom?
Probably not. I dare say with having read the entire internet, we can do better with what we’ve got.
Grant
The average LLM sees the same amount of content that a four -year -old does. So think about that. So all of it’s been ingesting, you know, for everything, right? Yeah. So to me, that becomes the next, the phase of the LLM is computer vision, putting eyes on it, you know, cameras, walking around and understanding.
Then we open up a whole more can of worms. That’s exactly it. But if you think about that’s in effect what we’re getting today, what are we going to get in three years from now?
AB
Well, we’ve used, we’ve used the metaphor of the early LLMs, sorry, not LLMs, the early BERT. So I think we’ve forgotten half the name of these things. Yeah, that was the bi -directional. Transformers, yeah, CNNs, all of those. Could finish a sentence. It was handy, but it wasn’t very, wasn’t great. We quickly got to large models were as good as a primary school user, and then a middle school, and then finally a high school.
I think it’s safe to say we’re at academic tertiary level asterisk in many fields. But to then roll that metaphor back to, even with all that content of only having, you know, a preschooler’s level of content absorption, being able to extrapolate up to those levels.
Yeah, okay. I wonder what does happen in five and 10 years time when we’ve got, yeah, I’m drawn back to science fiction, as always. It’s 2001, Space Odyssey, HAL, not irrespective of the letters were one before IBM. We’ll leave THAT controversy a different day. But the Arthur C. Clarke’s metaphor there was that the creator of that supercomputer had spent almost a decade with it teaching, training it, talking to it.
So perhaps we just do need time and time will resolve itself. And the funny thing is less would be my prediction though. Surely we’re not going to have the plethora of copy paste. Each student decimated smaller versions of the large models will continue.
But I don’t think we’re going to have a random entrant from you or me in our garage going to take over one of the leaders.
Grant 41:58
I don’t think we’re going to keep the leaders, but one of the things I talk about is, you know, wisdom of the crowds with people. And I talked to folks as the price of the LLM API tokens will fall because they will, you know, that’s just the nature of, you know, unless you, unless energy prices go through the roof over time, the Silicon will decrease in price and we’ll be having, you know, H100s and A1, we’ll be able to get them a lot cheaper. What will happen is the LLMs, they don’t, LLMs don’t hallucinate the same way. Right. So if I can call the same question to four LLMs and then have a separate LLM, so in effect, chaining them together to get a response, I could probably be pretty confident.
So to me, that becomes that wisdom will be one of, you know, just how we use an ensemble of model machine learning models, we’ll be using an ensemble of queries back from an LLM to understand.
AB
So today we would have an LLM prompted, you are an academic, I want you to respond in this tone, having those being persistent agents and asking your own personal AI body of knowledge and then have them battle out for who gets to answer.
Grant
Yeah, well, even just being able to synthesize what four of them talked about. Right. So, and being, and then maybe fine tuning one that basically starts to resolve out when, you know, if there’s discrepancies.
So to me, that means there’ll be interest, even if you look at the biggest models right now, uh, they’re not just one model, they’re a collection of models underneath. Yeah. And we’re going to see more and more of it.
AB
Last question then for you, if I can. It’s a big one, which may not have an answer.
But I guess the last few topics, last few questions have been leading up to the concept called RAG, which the author acknowledges is if they had known what a concert was going to be, they would have given it a better name.
RAG is Retrieval Augmented Generation, which is not any help either. Essentially, it’s keeping your hot, hot, and your cold, cold, keeping your LLM changeable. And when that gets better next month, you can switch it out for the next one, but bringing your own data to an interface and then having you interact with it.
Right now, large models, any foundational models, don’t have a concept of, well, you or me. They’ve got a concept of people like T-shirt, green background, green T-shirt, chatting on a podcast. They won’t make a picture of Grant or myself.
They’ll make, we can prompt them to get close to Will Smith eating spaghetti, but it’s never, make me a video of Will Smith eating spaghetti. It’s, man, these features, spaghetti on a plane, go. RAG is the thing that actually brings those together and lets you have your own data set safe, brought forward, and then you keep on switching out what is the latest and greatest front end to be able to interrogate.
Open question to you, and is that something the data rock crew can help with to smash together all that digital twin of your own organization? Smash together with the latest and greatest.
Grant 45:29
So we’ve actually, RAG, when I talk to customers, RAG is in effect kind of, you’ve got your base answer, you’ve got your RAG, and then it’s out to fine tune if you really need to get it right.
Fine tuning is an incredibly expensive to do, but for most organizations, RAG is good enough. So last year, even with Dataiku, we worked with a LG Chemical in Korea for their health and safety data. So help it, you know, build a RAG inside of the tool in order to query that with their own external application.
So for us, this is, I see RAG, especially being a way to provide a lot of value very, very quickly to an organization, being able to ask those questions, being able to ask those questions very fast. I would say this, there is a question right now of context length, because in order to use a RAG, you have to, in effect, break up different elements of it.
So you’re chunking them or batching them for most part. And that, and in the end, what you’re asking it to do is do some, you know, basically do a Google search inside of it. Here are the five things that approximate your question, give that to the LLM and then the LLM responds.
It’s not a perfect, it’s not a perfect way of dealing with things. So for me, this is one of those where I’m gonna watch this space. We absolutely can do it. And I don’t think it’s going to go anywhere, anytime within the next two years, just because context length.
But is it the be all and the end all? I don’t think so. I think it’s a way point. It’s gonna be a, you know, it’s a way point in much the same way as settlers are moving, you know, in the United States to use my, they moved West and you’d have different outposts RAGs, just gonna be a really significant.
Grant 47:47
So for me, that’s, that’s what RAG is right now. It’s a, but it’s a way to get a heck of a lot of value very, very quickly.
AB
Yeah, but not the final result, gotcha. .
Grant
And more important thing, restricting down what the LLM can talk about, right? So being able to say this is the data, this is your data set to work with. That is probably one of the most important aspects.
AB
So this is your corpus.
Grant, thank you so much. Absolutely awesome to have a chat with you. We do need to do a follow-up by all means. Let’s get you back as a regular.
Grant
Sure, absolutely. At any point in time.
AB
Where do people find you these days? Or where would you like people to find you? Or any call to action you’d like us to leave us with for personal or data record?
Grant 48:34
So, if anybody’s interested, you can find me on LinkedIn. I’m typically posting something, you know, every few days or so that I find interesting. You may or may not, but that’s the best place to get in touch with me.
If you are interested in this space and your organization is trying to figure out, Hey, what do I just do with analytics all the way up to how do I get everybody working together, dataiku.com and feel free to try out, retrial of the tool or even if you just kind of a hacker.
Uh, I know Andrew, when you were going through school, you can, you know, you can download Dataiku into your, uh, your notebook and you know, work on it locally.
AB
It’s the closest thing to magic there for many many years There are other magic tools like LLMs being able to do all your code for you that you know I means that’s that’s the one to watch of a full -on architecture enterprise tool versus is there a magic? Invoke any of my voice assistants right now, but you know what happens when even that gets abstracted out But Dataiku is a brilliant tool and I heartily advocate for it. It saved my bacon many times.
Grant thank you so much for that. Enjoy the rest of your day. Cheers for the chat
Grant
Cheers. Thanks so much, Andrew, for the time.
AB
No worries. Well, that’s all of us from SPAITIAL. We’ll catch you next week with another special guest from a different part of the world. We won’t be daylight next time I’m chatting to you. For all of us here at SPAITIAL though, thanks for your time and we’ll catch you on the next episode.
Bye-bye.
HOSTS
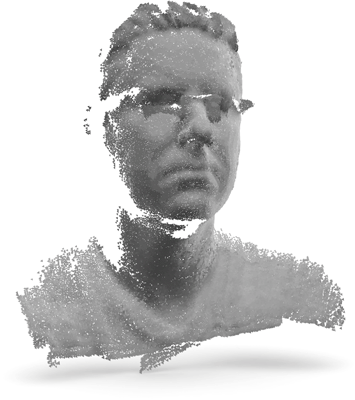
AB – Andrew Ballard
Spatial AI Specialist at Leidos.
Robotics & AI defence research.
Creator of SPAITIAL
To absent friends.