Machine learning is revolutionizing weather forecasts, moving from country-specific predictions to looking at global weather patterns, and pushing accuracy closer to traditional methods. WeatherBench 2, a new open platform, lets researchers rigorously compare models and accelerate progress for better weather predictions worldwide.
Weatherbench 2 from Google Research provides standard testing conditions for new and emerging AI/ML-based global weather models. With Moore’s Law firmly in place regarding available computing power, the developers of these global weather models insightfully determined that by moving from country-sized forecasting to continent-sized forecasting – the obvious final step is to keep going, and provide forecasting for the entire world.
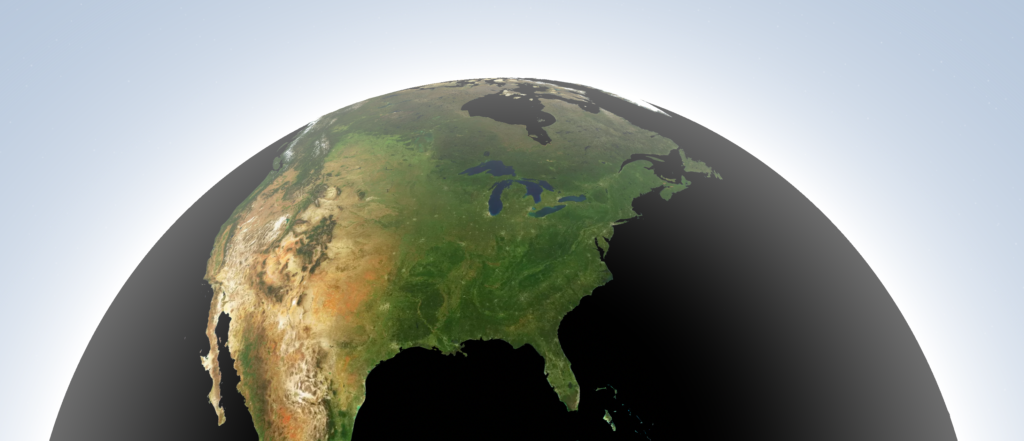
The 13 global models that WeatherBench 2 has already scored range in final resolution from 1.4° to 0.25°, roughly equivalent to 150km/100mi down to 30km/20mi resolution.
This kind of final resolution is edging close to the native resolution of national meteorology forecasting – so the obvious question is then: yes, but how good is it? Can these global forecasts handle regional and suburb-level localisations?
The answer is: hold the phone. Or to quote Dr Károly Zsolnai-Fehér – we’re looking forward to seeing what is possible in two papers’ time.
Below is one of the scorecards for all 13 models, rated against different deterministic and relativistic measures, all contained within the benchmark. Blue is better / red is worse predictions as compared to the known observations.
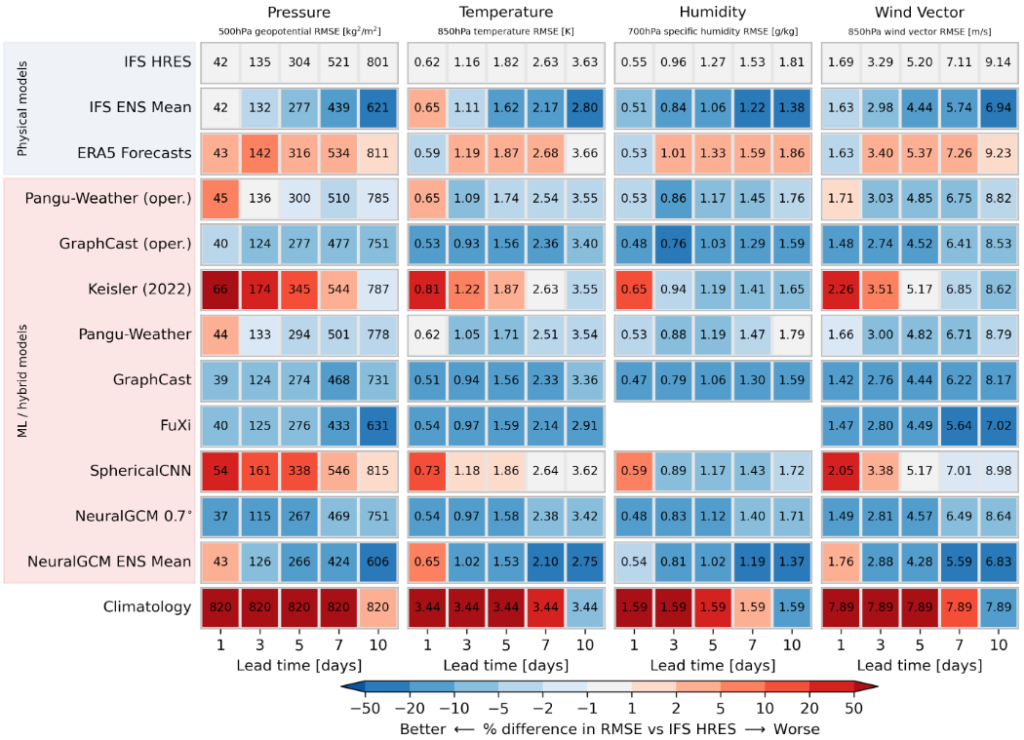
Perhaps the bigger question is: what will be the role of country-specific meteorological organisations when models such as these become another order of magnitude more accurate…?
More the point – how ‘good enough’ is ‘good enough’ weather forecasting if the entire world can have their own perfectly-personalised forecast – aka ‘nowcasting’?